Labelbox•March 5, 2021
4 strategies for successful labeling operations
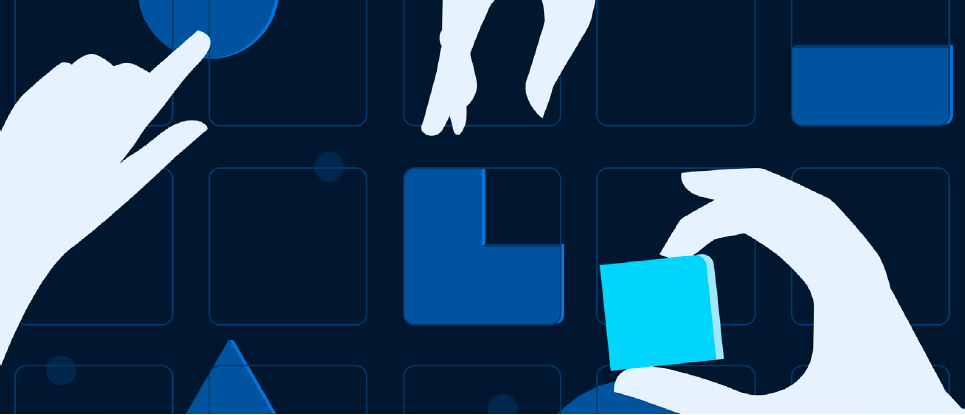
How do you label data for Machine learning (ML)? ML teams often require large volumes of meticulously labeled training data, and building a robust labeling operations process can be an arduous, and frequently underestimated, challenge.
At Labelbox, we’ve watched our customers develop their labeling operations for machine learning over time, both in partnership with the Labelbox Workforce and with their own labeling teams. Below are four key strategies to developing a labeling operation that serves your ML team with accuracy and efficiency.
Figure out the specifics of your business case
Define precisely what the model needs to accomplish first. A use case involving object detection will have very different data annotation requirements than classification, object tracking, or instance segmentation.
How the model will be used and by whom will determine the accuracy level that it needs to achieve. This will inform how your labeling ops team will source the training data, how the data will need to be adjusted and annotated, and how much training data they’ll need to develop. If the ML data labeling team starts creating training data without all the relevant information about the model and use case, the data is less likely to be effective and the training process will take much longer.
Start small and iterate as much as possible
Once you’ve established the business case, you’ll need a hypothesis for how the model will work and how training data will need to be annotated. The next step is to source and develop training data. Iterative, thoughtful development is key to creating a sufficient amount of high quality training data.
Rather than brute forcing a large dataset, the ML data labeling ops team should first consider how generalized the model should be and what variations the dataset should include (such as geographies, seasons, etc.). Then set up an iterative cycle with a small, diverse sample to train the model, test your hypothesis, find where it fails, and alter the amount of data or the way it’s annotated until the model is improving satisfactorily.
Adding breaks in your ML data labeling process to test and validate might seem like it lengthens the timeline, but they’ll be minute compared to the delays caused by a large dataset full of errors. While the initial timeline looks shorter for a non-iterative labeling process, teams find that iterating quickly and early delivers faster, better results.
Document and assess your labeling operations process
Labeling operations is still relatively new for most enterprises, and developing a robust system that serves an organization’s specific needs will likely require some experimentation. That’s why it’s so important to carefully document each part of the process. Here are a few key considerations to keep in mind:
- Every role on the team, from domain experts to labelers, should be performing their tasks according to the same guidelines. This way, the data labeling process is repeatable and scalable.
- When data quality issues arise, document what they are and how they should be avoided in the future, and alter your process accordingly.
- Monitor costs over time, so the team can better estimate expenses. When a cost estimate is incorrect, document the reason.
- Integrate a feedback loop to communicate adjustments, problems, and other information with everyone on the team to avoid confusion with an evolving process.
Invest in machine learning expertise
Enterprise ML models are usually built to solve specific, complex problems, so the ML data labeling operations team should include several domain experts who understand these problems and the issues you might face when addressing them. Labeling operations leaders should prioritize their voices when making decisions about the process.
ML data labeling teams will also benefit from experienced labelers. It can be difficult to find those with the right background (there’s no such thing as a degree in labeling — yet) but having this expertise on staff will save significant time and costs. Often, ML teams will have their own data scientists and ML engineers label data, and while they know what the labeled data needs to look like to train their model, creating it is an entirely separate skill. An experienced labeler will also be able to better communicate needs with external labeling teams, monitor their progress, and help build labeling guidelines that improve efficiency. For more insights, watch Labeling Operations — Your Secret Weapon.
Final thoughts on labeling data for machine learning
Ready to strengthen your labeling operations? The Labelbox Boost services offers services to help you create a data labeling workflow, create labeling ontology, train your labeling team, and much more.