Labelbox•April 26, 2022
6 fundamental sessions on improving your AI data
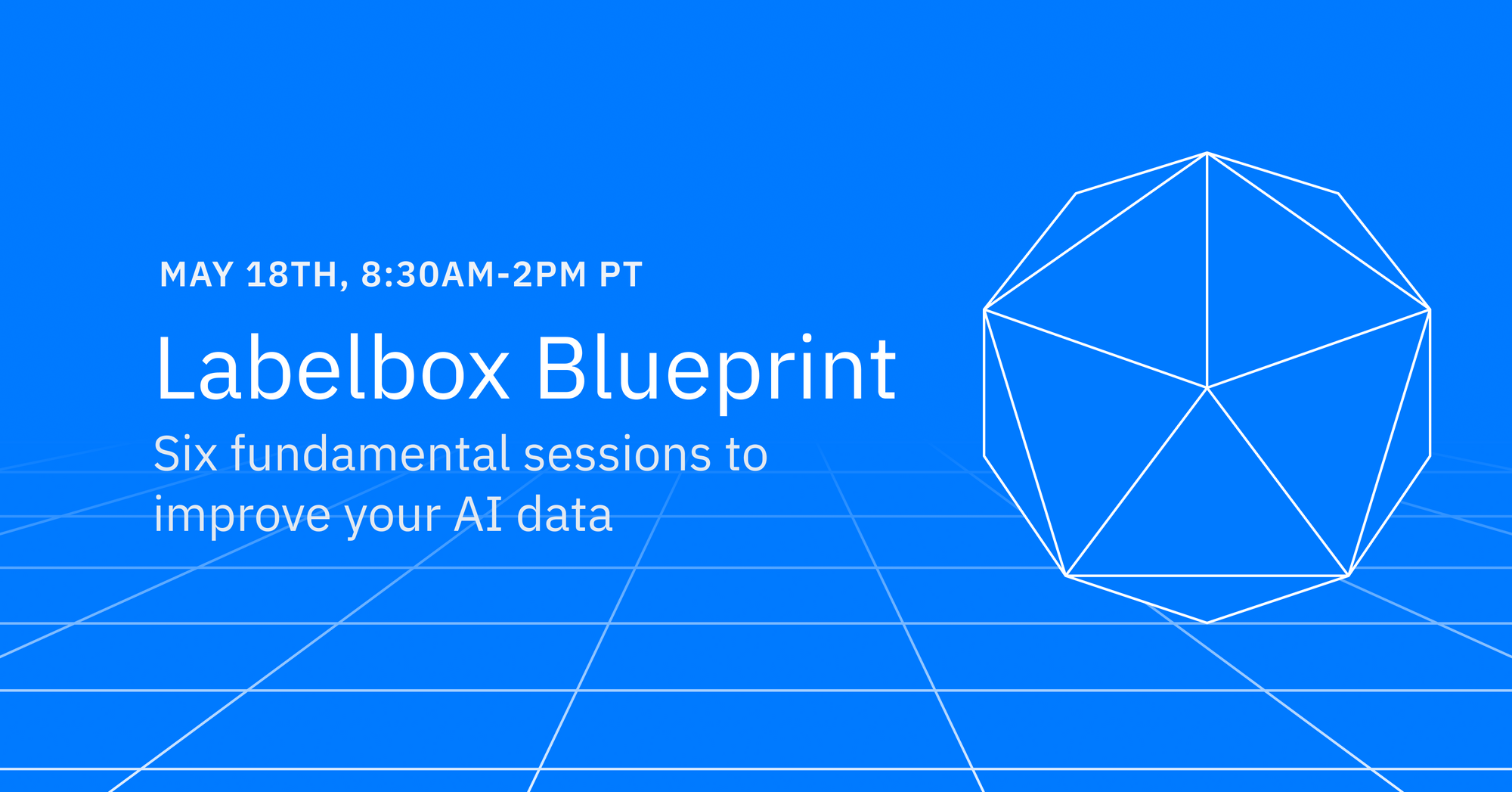
Join us at Labelbox Blueprint on May 18th - A virtual event covering six fundamental sessions to improve your AI data
Most AI-focused events center on the future of AI rather than practical guidance and information for data practitioners and AI builders who are on a journey toward efficient, data-centric production AI.
Labelbox Blueprint aims to provide this in six fundamental sessions, led by fellow AI builders, to help you find new ways to solve common challenges with data quality and improve your AI data processes. Keep reading to learn more about these sessions and what they'll cover.
Join us on Wednesday, May 18th from 8:30 am to 1:30 pm PT. Register today to save your spot.
How to build a data engine for your organization
AI is supposed to be transforming organizations, but data leaders find that it takes much longer than anticipated for their AI investments to get to full impact. At the heart of the problem is the process of converting proprietary data into revenue-generating AI and the exponential need for labeled data. To solve this, learn how leading enterprises have built AI data engines that allow their teams to prioritize and iterate on the right data based on the stages of their project’s life cycle, enabling them to build lasting competitive advantage and get to production AI faster.
How to improve performance with less data
Machine learning has evolved past the traditional method of iterating on models with a single large training dataset. Today, leading ML teams extend their focus to carefully selecting their training data, training the model, examining its performance, and modifying the next training dataset accordingly. Learn how to conduct model error analysis and data curation, both of which are vital to data-centric ML.
How to properly leverage labeling automation
Learn how to dramatically reduce labeling time using the latest in automation from specialized annotation tools to model-assisted labeling. See what workflows can help you increase labeling velocity whether you’re actively iterating on production models or are just getting started on your AI journey. Teams using model-assisted labeling have seen a savings of 50-70% in terms of annotation costs and can dramatically improve overall project efficiency.
How to upgrade your data QA process
Preparing training data for real-world models requires a sophisticated quality assurance plan, with considerations of multiple passes, consensus decision-making, gold data insertions, and more. Learn how to utilize the right workflows and infrastructure to get a real-time view of data quality and labeler performance.
How to collaborate in lockstep with your labeling ops
As more ML teams shift their focus from iterating on models to improving the quality of their training data, labeling operations is becoming a central pillar of their larger MLOps strategy. Creating, managing, and maintaining an efficient labeling pipeline that produces high-quality training data is an underestimated challenge. Learn how leading AI teams can improve their labeling operations to deliver accurate and scalable training data by emphasizing better collaboration, transparency, and communication.
How to build a strong foundation for ML when you don’t yet have a model
Learn how you can derive significant value from building a foundation to manage your in-house data and IP, even if your enterprise is months or years from deploying AI. This session will also cover how AI teams can more easily train, evaluate, and improve ML models by combining these workflows in their training data platform.