Labelbox•August 22, 2019
Labelbox August Updates
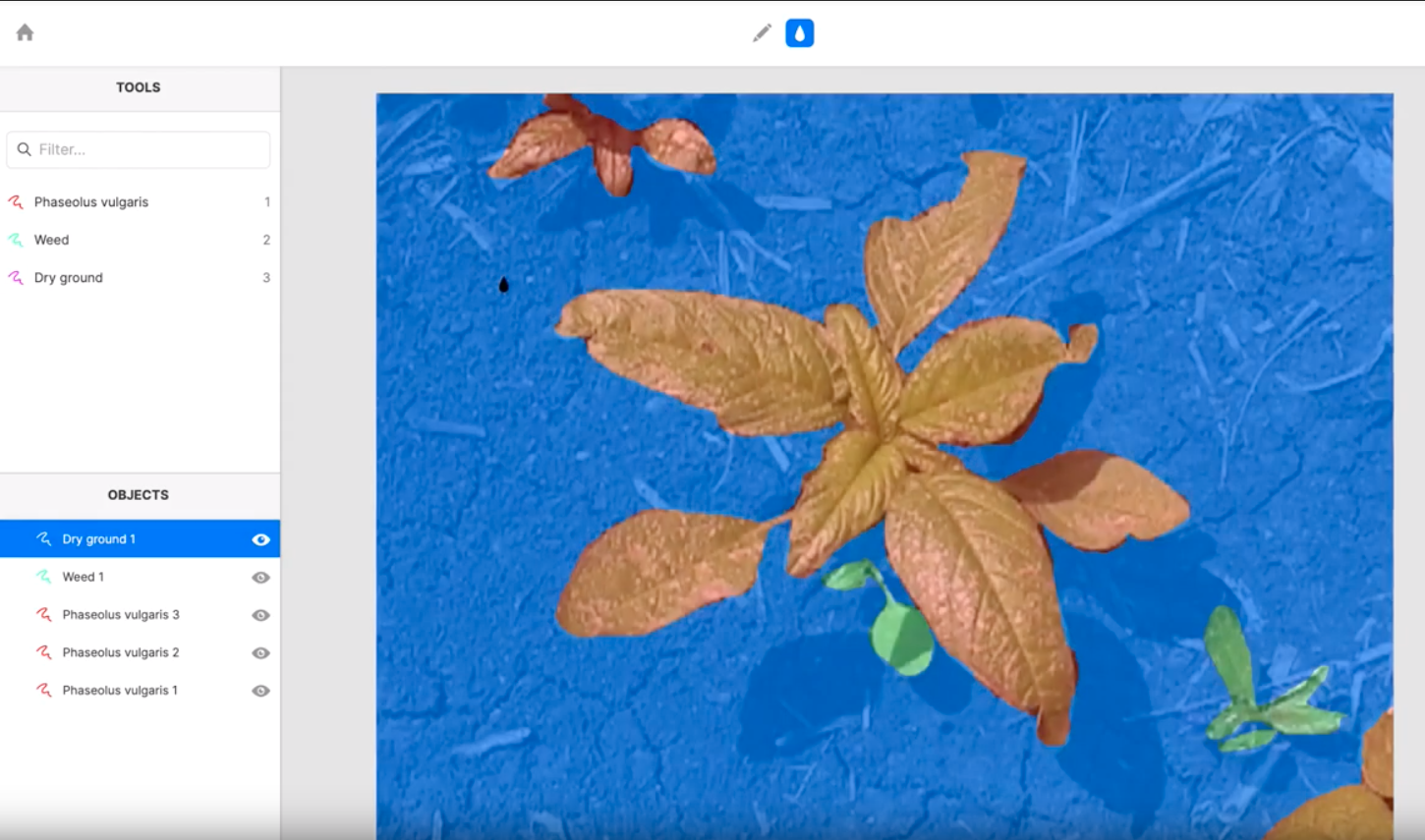
August Highlights from the Labelbox Team
It's been a busy summer for us at Labelbox. We're excited to share our updates from the past month with you.
Image Segmentation
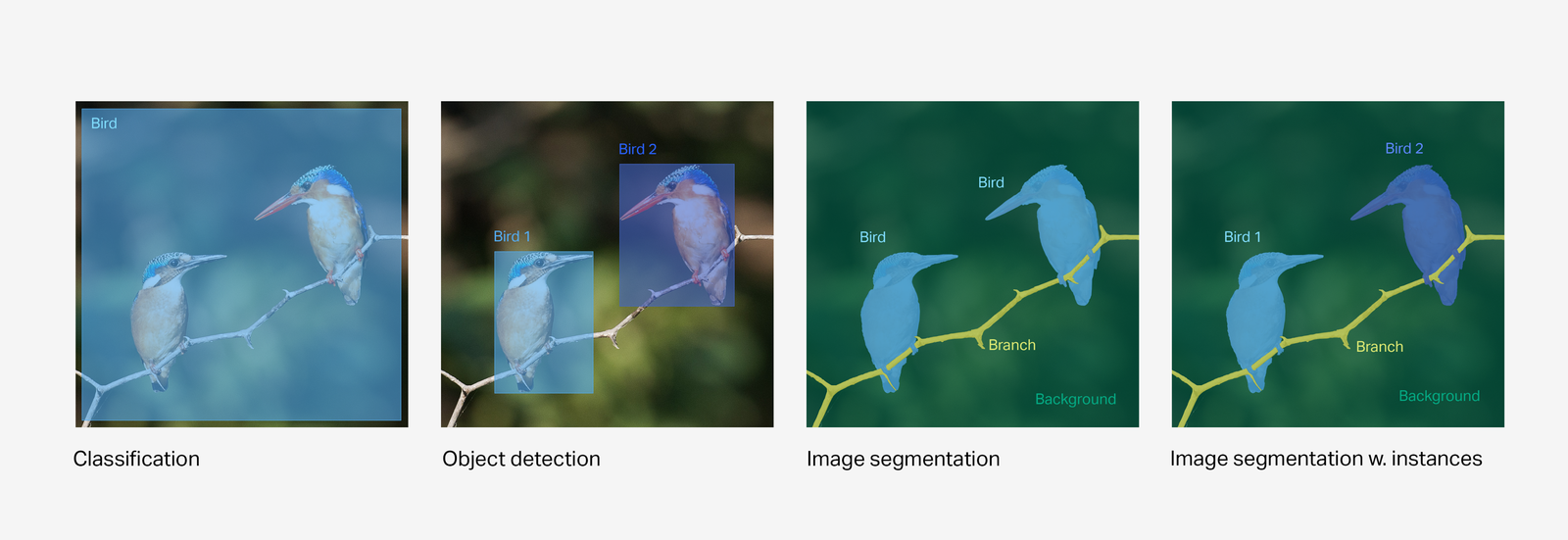
We recently launched image segmentation and instances. Image segmentation differs from classification and object detection by allowing you to have fast, pixel-level accuracy for your image labels. We see customers using this feature for computer vision applications that require high accuracy such as autonomous vehicles, medical, and retail applications. You can read more about image segmentation in our release post.
Fill tool
For customers using segmentation, our new fill tool allows you to easily label background pixels around an object. This can be helpful when identifying an object against a background such as a weed surrounded by fertile soil or a fish against the backdrop of the sea. We continue to focus on being the fastest way to create high quality training data through improvements to our labeling workflow.
Improved delete & re-enqueue
Sometimes a project has a large number of assets that need to be relabeled. We listened to customer feedback and have now made it faster for teams to work through iterative improvements to their datasets. We now have a "select all" feature for deletion of labels which allows you to select all assets that meet certain filtered criteria. When you re-enqueue (add assets back to the labeling queue), you also can leave the previous labels as a template for the next labeler to adjust instead of completely deleting all previous labels. This saves time and effort for labeling teams, especially when only minor corrections are needed.
Upload any file type
With Labelbox, you can customize the editor to label unique file types for your use case. We've now expanded the capabilities of Labelbox to upload any file type to our servers. This is helpful for customers who have custom file types that would prefer to store their assets in Labelbox instead of linking via URL to their own data stores.
Labelbox uses a CDN to ensure that uploaded files are optimized for performance into whatever region your labeling teams are, whether they are at company headquarters or with an outsourced team anywhere in the world. For faster loading times we also pre-load images in each labeler's queue ahead of time so that the next image loads instantly in their browser.
New project overview with object class distribution
To improve model accuracy, your models need to have enough labels for each class in the dataset. With this latest improvement to your project overview, you now have access to a list of all classes represented in your project and a chart of their relative frequency. You can click into any class on the list and view specific examples of those labeled assets too. We want to make it easier and more transparent for you to improve model accuracy by helping you identify and tailor the additional examples needed in your project.
The Side of Machine Learning You’re Undervaluing and How to Fix it
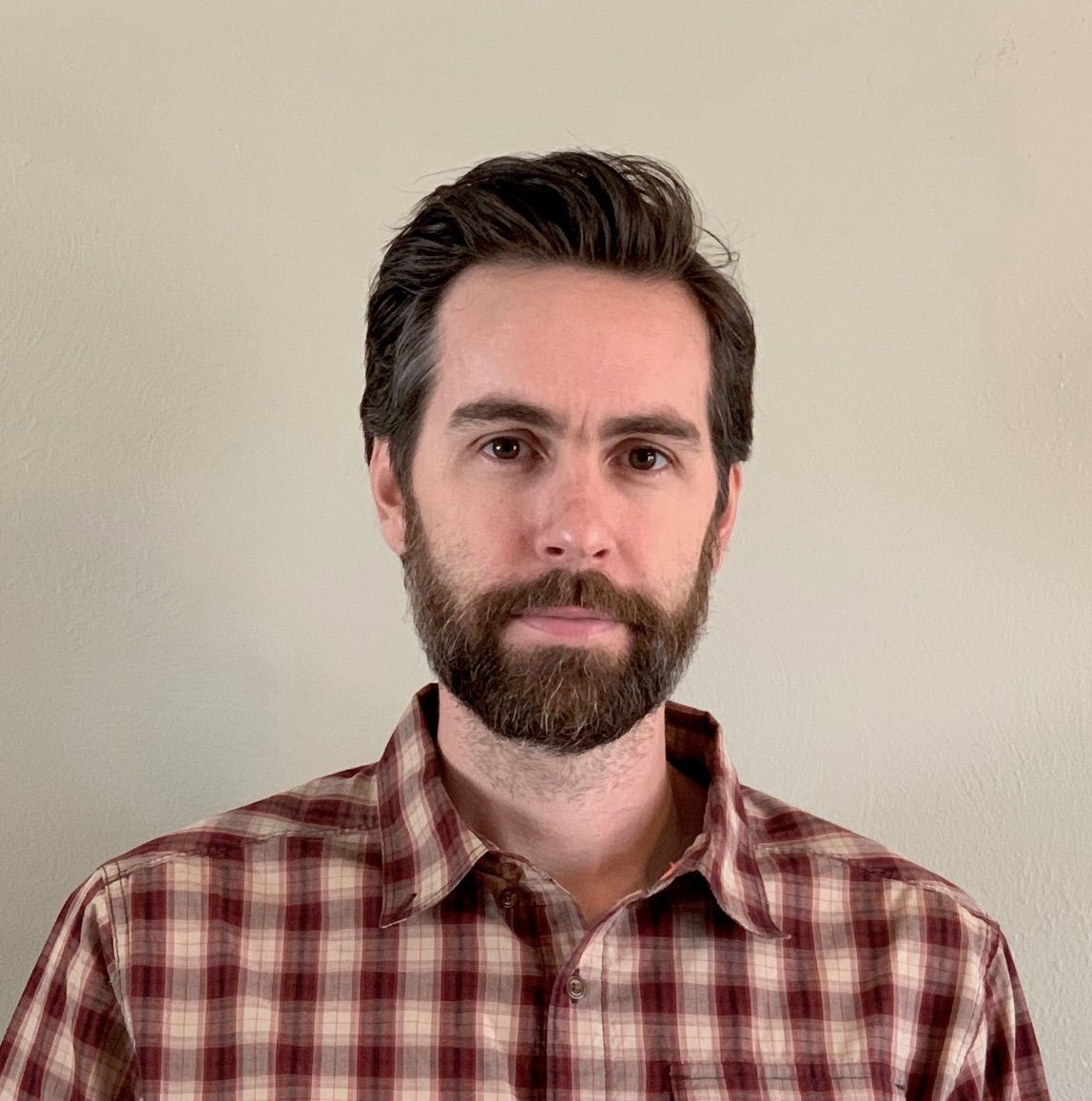
We recently invited Matt Wilder, PhD to contribute to the Labelbox blog. Matt brings a wealth of experience advising companies on their AI journeys, especially as they move to production use cases. In his post, Matt describes a well-oiled machine learning operation and how many companies undervalue the importance of quality training data, setting back their business objectives. You can read the full post and learn how Matt advises companies to avoid this common blind spot.
Supporting AI for Good Projects: Omdena's AI Challenge
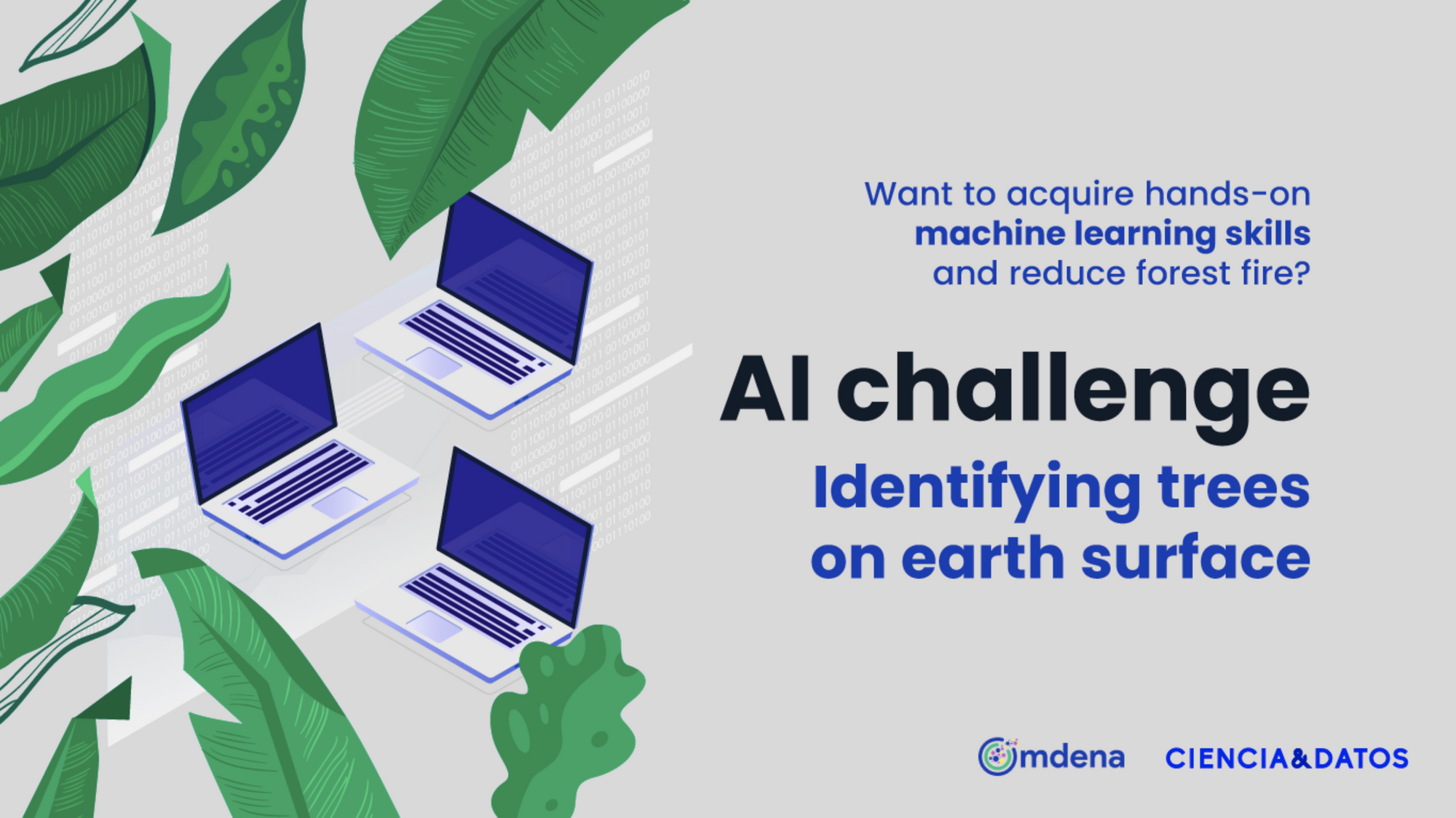
At Labelbox, we’re proud to support projects that serve the common good. A few months ago Leo Sanchez reached out to us about Omdena’s AI Challenge and how Labelbox might be the right tool to help them tackle a computer vision problem: how do we build a classification model for trees to prevent fires and save lives using satellite imagery. Well we were pleasantly surprised that Leo took the time to write up his experiences about joining an international remote team working on this project, what it was like running a labeling team, and how they used a U-Net convolutional neural network to find a solution. You can read the full story of Leo’s experiences and Omdena’s AI Challenge on our blog.