Labelbox•June 27, 2020
Stanford CS230 grad students research the next generation of AI-driven land urban air vehicles
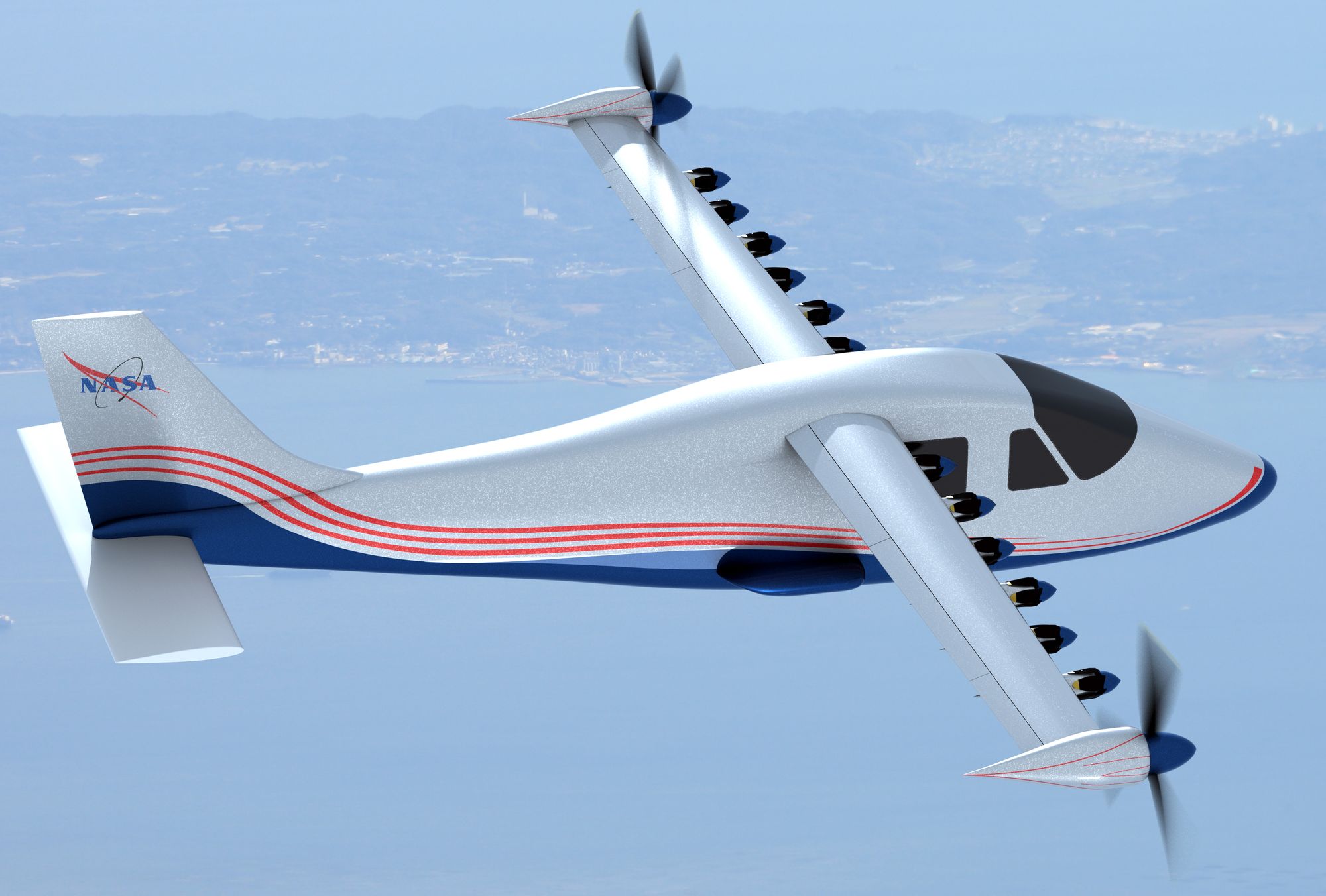
Overview
Andrew Denig and Seraj Desai are master's researchers at Stanford University attending CS230 Deep Learning, one of the most renowned programs for artificial intelligence led by Andrew Ng. As aerospace and aeronautical engineering students, their research project focused on identifying suitable areas to land urban air vehicles through satellite imagery.
Project constraints and obstacles
In the time span of 2 weeks, Andrew and Seraj needed roughly 5,000 satellite images segmented into regions of landable and not landable areas. This image segmentation task is highly non-trivial, as the task is even difficult for most humans to complete successfully and consistently. This is a more time-consuming task than object detection, which requires a bounding box, or image classification, which requires the labeler to identify what is in the image.
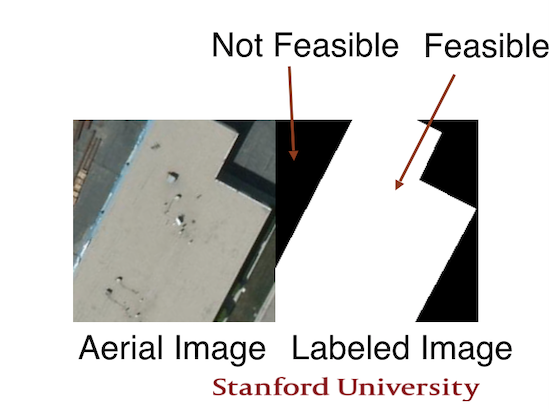
Because their Stanford 230 course project demanded an end product at the end of the 8 week period, Andrew and Seraj could not afford to spend 6 weeks labeling their training dataset. which was roughly the time required for two of them to complete the entire labeling task ourselves and given their availability to work on the project.
Enter Labelbox & Workforce
To solve the obstacle they encountered, Andrew and Seraj labeled 100 images themselves to serve as benchmarks for other labeling teams to help. Andrew provided a one page PDF with labeling guidelines. With this, the Labelbox Workforce team was able to begin labeling images themselves. Within a week of beginning the project, they already had more than 2,000+ labeled images which allowed them to begin constructing and training preliminary models.
When Andrew and Seraj compared the label quality that they generated in the 100 test images to the quality of the 5,000 labels created by Labelbox Workforce, the attention to detail in the Workforce-produced data was evident. One of the defining features of a high-performance labeling team is their ability to deliver quality labels with accuracy while not sacrificing speed. By watching some of these labelers in action, their precise abilities can be at times comparable to professional video game players who possess the manual dexterity to carry out a high number of 'actions per minute.'
The key is in the data
Andrew and Seraj worked with Labelbox's team to spend the time necessary on each image to make sure that the edges of the landable regions were meticulously traced out. This really aided their network in being able to distinguish the two zones from one another, as there were clear boundaries following contours and texture changes in the training images.
They were able to begin uploading their own data and labeling images within 5 minutes of making creating their accounts. Once the labeling task is done, the capability to export the labels as a JSON file makes loading the dataset into a deep learning model very simple, as leveraged the libraries available to work with JSON files.
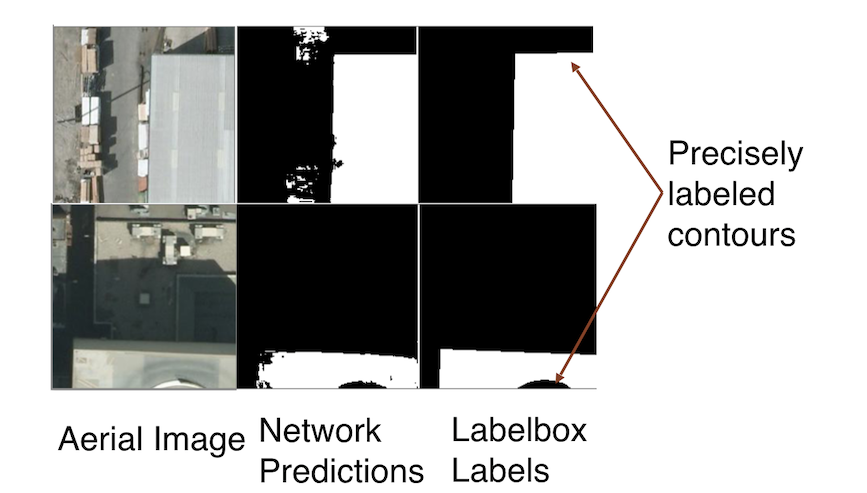
Instead of labeling the data themselves and only having 2 weeks to create and train their models, Andrew and Seraj were able to dedicate a full 7 weeks to training and improving their models. This advantage was very beneficial to the final outcome of the project which allowed their neural networks to learn patterns in the training data more easily.
Conclusion
Labelbox's training data platform and Workforce helped their research team create better labels. In their words:
“Using your platform and Workforce service has been very easy and effortless. The quality of the labels, especially with regard to the care that was taken to make sure the boundaries of each zone were traced out as accurately as possible. This has really improved the results of our neural network beyond what we could have ever achieved.”
You can check out the full video here: