Labelbox•January 22, 2021
Software to AI: a paradigm shift
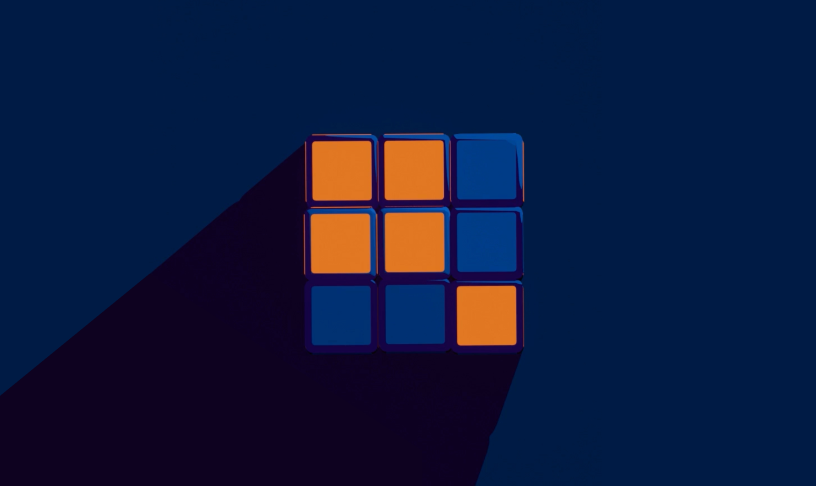
The first session of the Customer Summit in December was a fireside chat titled “How Data Unlocks AI,” between Labelbox CEO and Cofounder Manu Sharma and Andreessen Horowitz’s Peter Levine. A large part of the discussion centered around the differences and similarities between software and AI.
Manu kicked off the talk with a description of the shift from software to AI. “Building AI applications is fundamentally different. Instead of software engineers writing logic statements to solve a problem, domain experts are labeling data, which is then used to train neural networks.”
Iterating fast is much harder in AI — and it’s the key to success
Over the past few decades, we have built tools and workflows for software development that enables fast iteration, which is the cornerstone for success in software, as it allows developers to get feedback and make improvements faster. AI is similar: teams come up with a hypothesis to solve a problem, create a training dataset to teach the neural net, test to see if it actually works, and then make necessary changes. This cycle repeats until they have solved the original problem. However, the cycle is incredibly slow compared to software development. "The best AI teams today spend as much as three to four weeks from an idea to a model,” said Manu.
Software systems might take a day or even hours to iterate, while AI systems often take weeks. "As we move to edge computing, processing power becomes unlimited. The iterative loop needs to be very short,” said Peter. When data is collected in real time, but the AI takes weeks to iterate, it defeats the purpose of having edge compute. "We talk about this constraint like we have to get equal to software development. But I would argue that we need to be 10X faster."
Every company is going to be an AI company, and every domain expert is going to be a data scientist
Every industry is finding new ways to use AI, from claims automation in insurance to identifying cancer cells in medicine. "AI is going to be used with every function within a company, and I believe that every company becomes an AI company over time,” said Peter. Because the data used by each organization will be different based on their use case (and often proprietary), companies will need to acquire their own AI teams and processes.
Just as AI is going to become a necessary component of every organization, it’s also going to be created and used by everyone, not just AI engineers. With software, only programmers can write code. As we move away from code and towards data-centric programming, however, anyone can become a data scientist and participate in building AI. “We all practice the art of processing data,” said Peter. “Hundreds of millions of people are actually going to be using data for the benefit of the profession they work in.”
Managing and creating training data for AI requires a new class of tools — including a platform
AI models learn with data, unlike software based on a series of logical statements, so the underlying tools required are completely different. Because domain experts from every industry are set to become data scientists, "they need a new class of tools and technologies and workflows to be highly productive," said Manu.
Those creating training data need tools that let them annotate easily and efficiently, manage the labelers, engineers, product managers, and others involved in the process, and iterate faster. Peter and Manu also stressed the need for a platform made especially for training data. A platform enables data scientists to collaborate, and share what they learn. That collaborative effort, having people interact and exchange ideas, will ultimately be what solves the challenge of iterating faster in AI. Watch the full discussion below.
Want to learn more about how a training data platform can help your team accelerate their journey to a production ML? Download our Training Data Platforms 101 white paper.