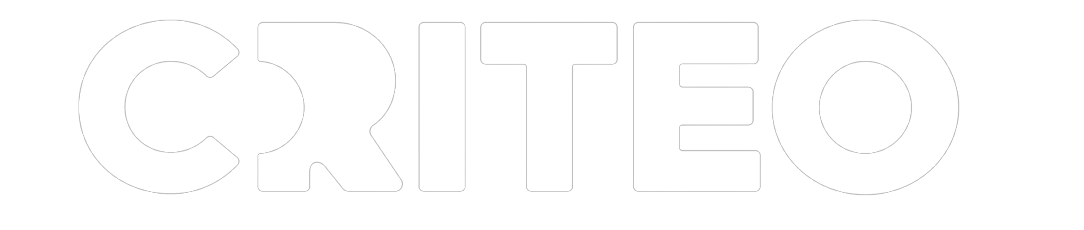
How Criteo builds better contextual advertising products with AI
Problem
Building products to improve personalized ad experiences require effectively harnessing high-volumes of unstructured image data. The Criteo team wanted to quickly scale their ability to improve the AI data used for their ML models across multiple project teams. This would allow them to more efficiently deliver on contextual ads such as identifying consumer products with complex backgrounds, as well as classify whether a web page is safe or not (i.e., if it was appropriate for users under a certain age range).
Solution
Labelbox Annotate was used as the primary platform to enable better internal team communication, resulting in a massive reduction in the back and forth needed to convert unstructured image data for AI use.
Result
Criteo’s Publisher Content team was able to immediately see a 40% gain in annotation delivery speed, as well as comparable increases in data annotation quality.
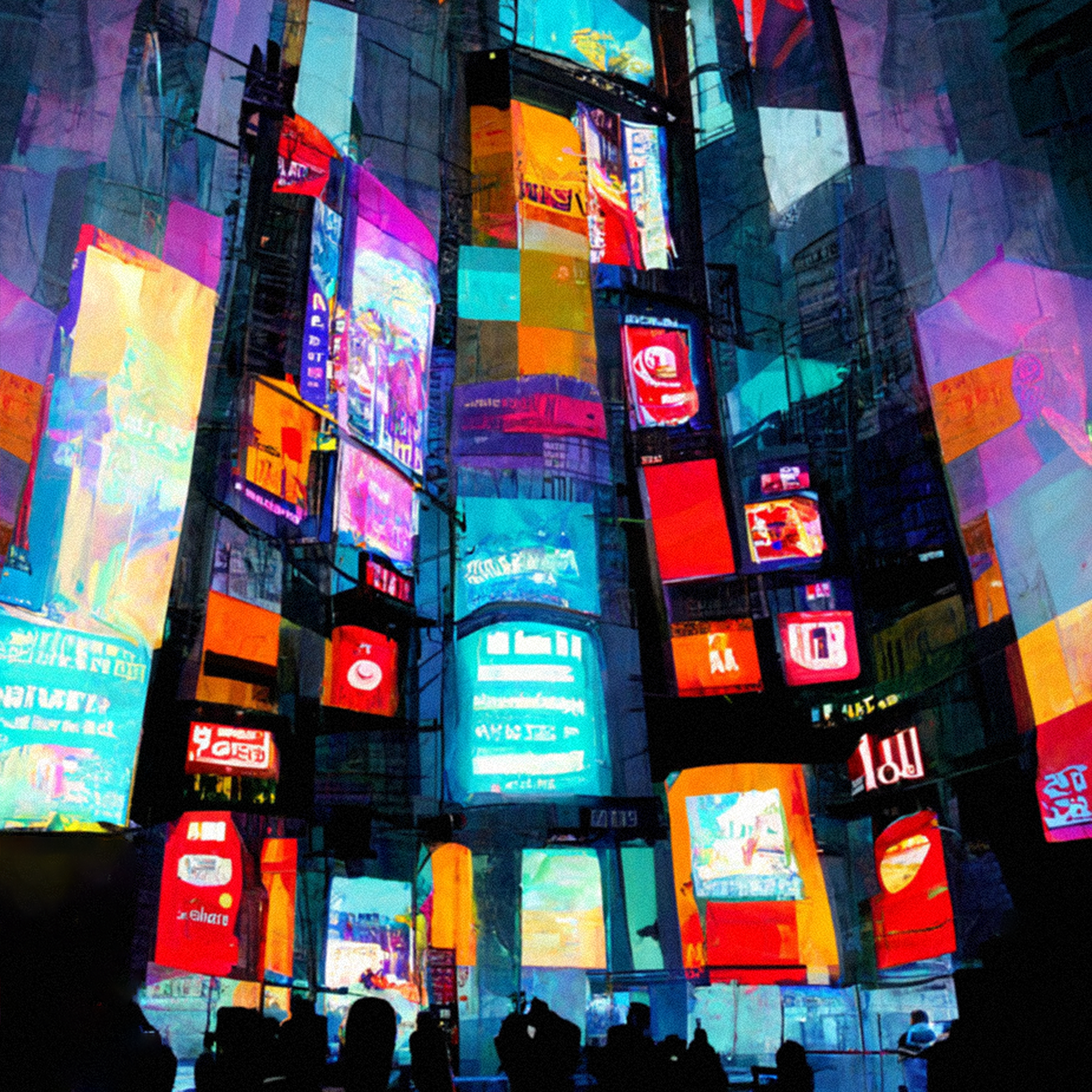
Note: This story is a recap based on a panel discussion at Labelbox Accelerate featuring Hong Noh, Senior Product Manager at Criteo.
Criteo is one of the world’s leading ad platforms that works closely with internet service providers to create personalized ads for consumers. Their mission is to deliver richer experiences to every consumer by powering the world’s marketers and media owners with trusted and impactful advertising. Criteo is harnessing AI and ML with the goal of driving the next generation of advertising engagement. Examples of this work include investing in AI-powered products that are able to make product recommendations and ensure brand safety within a webpage. Machine learning models are also used to understand and classify the context of websites from the open web, enabling marketers to show tailored messages on relevant sites and sites that will not harm the reputation of a brand.
Before Labelbox, Criteo’s Publisher Content Analysis product team faced an issue that many ML teams encounter when trying to build production AI. They were not able to reliably improve their label quality, efficiency and identify edge cases where their models were not performing well. Their team was reliant on managing all of their unstructured data through excel spreadsheets and defining what label quality meant through many back and forth internal emails. Criteo wanted to find an easier way to boost collaboration and allow their product and ML teams to focus on applying the latest data-centric approaches towards building ML. As a result, they started using Labelbox to work on a few core product initiatives where ML could help complement the personalized ads experience. This included background removal for better product identification, and whether a web page is safe or not (i.e., if it was appropriate for users under a certain age range).
After adopting Labelbox, Criteo’s ML team found that there was an enormous benefit to having a data engine and a dedicated platform for improving their training data and models. It provided their product and data science teams with the ability to better communicate with each other, resulting in a massive reduction in the back and forth that they had to do on a daily basis. For the background removal project, a few pixels could mean a lot of fluctuation which meant that having a dedicated platform where subject matter experts could do the human review would significantly impact model performance. Another advantage of utilizing Labelbox versus open source platforms or even in-house tooling was that the stability of the platform ensured that their labelers - who were often based in many different parts of the world - could access the solution anytime securely and seamlessly.
In terms of results, Criteo’s team was able to immediately see a 40% gain in both increased speed of annotation delivery, as well as comparable increases in the quality of their annotations. In the future, the Criteo team will be looking to build on its competitive advantage by enhancing its ML-powered products with more capabilities such as fake news detection, contextual signal detection on video content, and tracking user attention (eye tracking).