Labelbox•April 8, 2019
Labelbox Speaks on Ethics of AI at O'Reilly's Strata Data Conference
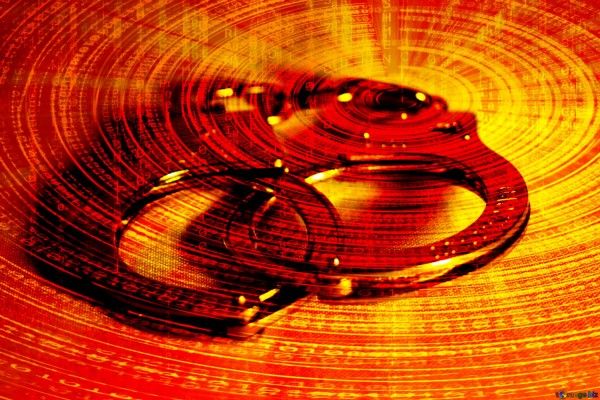
An ML Model Is Only as Good as Its Training Data
“Is your AI really making good decisions or have you built a deceptive black box that reinforces ugly stereotypes?” — Strata Data Ethics Summit, O’Reilly & Cloudera
An audience of data scientists, analysts, engineers, developers, and executives attended the Strata Data Conference to hear AI thought leaders answer this difficult ethics question. This article summarizes the talk given by Labelbox Co-founder & COO, Brian Rieger, who presented on how to solve for bias in data labeling in order to use machine learning applications to create a more just society.
An audience of data scientists, analysts, engineers, developers, and executives attended the Strata Data Conference to hear AI thought leaders answer this difficult ethics question. This article summarizes the talk given by Labelbox Co-founder & COO, Brian Rieger, who presented on how to solve for bias in data labeling in order to use machine learning applications to create a more just society.
The Root Cause of Bias in ML
In order to understand how bias enters machine learning, it is important to understand how industrial supervised machine learning works. First raw data is collected. Raw data starts out unstructured. Machines cannot derive meaning from unstructured data.
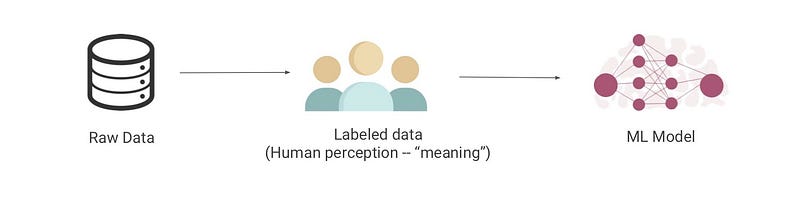
That’s where humans enter the picture. Humans are needed to label data in a way that is perceivable to machines. Humans are constantly bombarded with sensory data. We interpret that data to create meaning. Unlike humans, machines do not have interpretation structures like evolution, society, or upbringing to help them interpret unstructured data. Human labeling essentially structures data to make it meaningful. That labeled data is then used to train a machine learning model to find “meaning” in new, relevantly similar data.
Therefore, labeling is the key step! The more accurately data is labeled and the larger the quantity of such labeled data, the more accurate and useful the resulting machine learning model. Note that the algorithms themselves are at this point mainly commodities. It’s the labeled data that matters. Models base their decisions on labeled data. No data, no decisions. A successful model is a model that can make accurate predictions when presented with new data.
The key to building a successful machine learning model is having an enormous amount of high-quality training data. Training data is the labeled data used to train models. For computer vision, teaching machines to see (which is a requirement for autonomous cars, for example), people label images of streets, roads and pedestrians. In the images here, you can see various kinds of data labeling of different images, with different labeling tools.
Human Labeling Brings Human Bias into ML Models
Why are we talking about how data labeling works in machine learning models? Because we care about bias in machine learning — how it happens and how to stop it. Eighty percent of machine learning is data prep and data engineering, of which most of this time is humans labeling. Since humans are biased, human labeling is biased.
Data labeling is the key to successful machine learning and also a root cause of how bias enters into machine learning models. We have to figure out how to stop bias at the point where we label data. Doing that will solve the root of the problem. A machine learning model is only as good as its training data because models learn from and reflect the labeled data that has been used to train them.
Biased ML Models Have Harmful Consequences
Autonomous car detection of darker skinned pedestrians is on average 5% less accurate.
Researchers from the Georgia Institute of Technology published a new study in February 2019 claiming that object detection models for self-driving cars are 5% less likely, on average, to identify a dark-skinned person compared with a light-skinned person.
However, this study has been addressed with some skepticism because the test used academic models and publicly available data-sets since private autonomous car companies do not make their data-sets or algorithms public. That said, as a society, we may need to require auditing and testing of private models for bias.
COMPAS is used for criminal sentencing in US — and is racially biased.
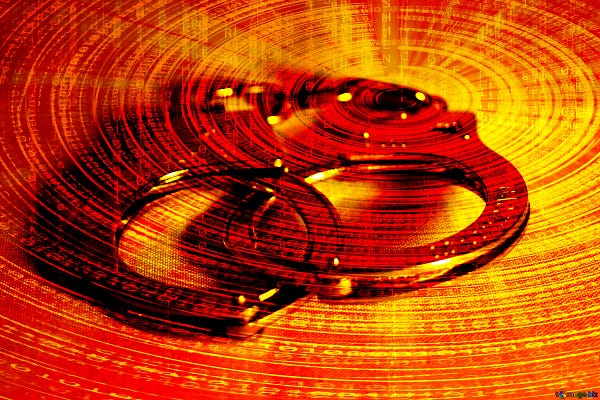
COMPAS is an algorithm widely used in the US to guide sentencing by predicting the likelihood of a criminal reoffending. In perhaps the most notorious case of AI prejudice, in May 2016 the US news organization ProPublica reported that COMPAS is racially biased.
According to the analysis, the system predicts that black defendants are more likely to commit crimes than they actually do and that white defendants are less likely to commit crimes than they actually do.
Equivant, the company that developed the software, disputes the report. It is hard to fully know what’s true here, or where exactly the bias may lie, because the algorithm and data that powers it are proprietary and so not open to public review.
Amazon’s facial recognition software performs worse on women, particularly darker skinned women.
The MIT Media Lab published a study in January 2019 that Amazon’s facial recognition software is very good at identifying white males and much worse at identifying women, particularly dark-skinned women. This software is used by law enforcement and so the ramifications of a wrong facial recognition here could be enormous. Microsoft is actually calling for facial recognition technology to be regulated in order to avoid bias in facial recognition software.
When algorithms of the most powerful companies in the world are biased, it’s an enormous social problem. To make an impact, we have to solve the root of the problem — how do we create less biased structured data which will, in turn, lead to less biased algorithms? To solve the root cause of biased models, we have to go back to the data labeling step and figure out how to make the labeled data less biased.
We need to build better tools for data labeling to help monitor and prevent bias in ML models. A key way to build less biased labeled data, which in turn will lead to less bias in models, is to create tools to monitor the labeled data, test it for bias, and make tweaks to the data accordingly. We need a system of record for data, along with quality assurance tools for the data.
Meet Labelbox
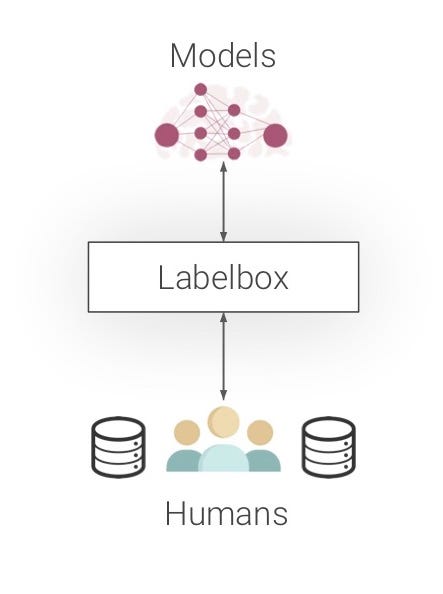
Labelbox provides a collaborative, extensible platform, to create and manage training data at scale. Labelbox supports quality assurance of model predictions that can be used to reduce bias. Labelbox is training data software for building machine learning systems. Labelbox supports machine learning by facilitating the 80% of time that is spent creating and managing training data. Every human is biased so it’s hard to stop it at the point of labeling. Quality assurance systems offer a way to systematically identify and correct for bias.
Labelbox is a system of record for organization-wide training data. Labelbox stores and tracks all data in one centralized place where it can be audited for bias in bulk, across organizations and teams. This data can be audited for bias and Labelbox can support quality assurance on a model’s predictions, in order to train it in a way that reduces its bias.
If society does decide to regulate machine learning algorithms we will need a system of record for the data used to develop such algorithms which can be audited and reviewed for bias. Labelbox provides such a system of record.
Data has never been more valuable — let’s ensure that data is being used to create a better world.
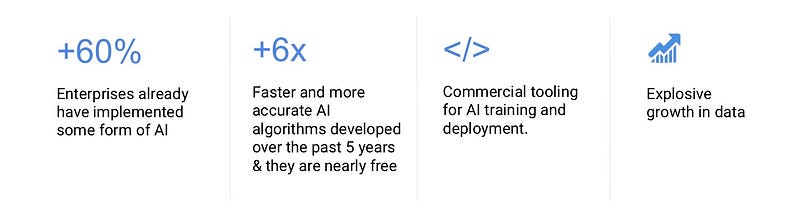
Why do biased data and biased algorithms matter so much? Data is the oil of the future — it’s a key piece of developing enterprise value in the 21st century. Access to more data and faster computer processing power is enabling ML applications in a way that has never before been possible. That data needs to be labeled and if we label it in a way that identifies and corrects for bias, the algorithms we create using that data can lead to a better, more just world.
Build Systems to Monitor AI Development
As a society, we need to develop systems and tools to monitor the development of AI and to limit bias in the systems that we build using machine learning. As data and ML algorithms play a bigger and bigger role in society, we need to develop systems and tools to monitor the algorithms that data is used to create. We need to make sure that our ethics is reflected in our algorithms, as they will play a key role in organizing our world.
To sum up, our default world is biased. If we can solve the problem of bias in the underlying training data, we have the opportunity to use ML to create a more just society.