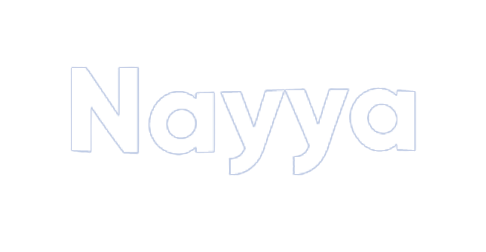
How Nayya advances personalized benefits recommendations using AI
Problem
Finding faster and cheaper ways to produce labeled data for both their offline model training, live prediction evaluation, and verification by subject matter experts.
Solution
Labelbox Annotate and Catalog, along with an SDK-driven approach to give their actuaries (SMEs) insights into how the model will generate predictions and evaluate these predictions.
Result
The Nayya team is now able to better visualize their unstructured data and leverage the Labelbox platform to maintain training data quality, save time, and train and test new models to improve their AI-first products.
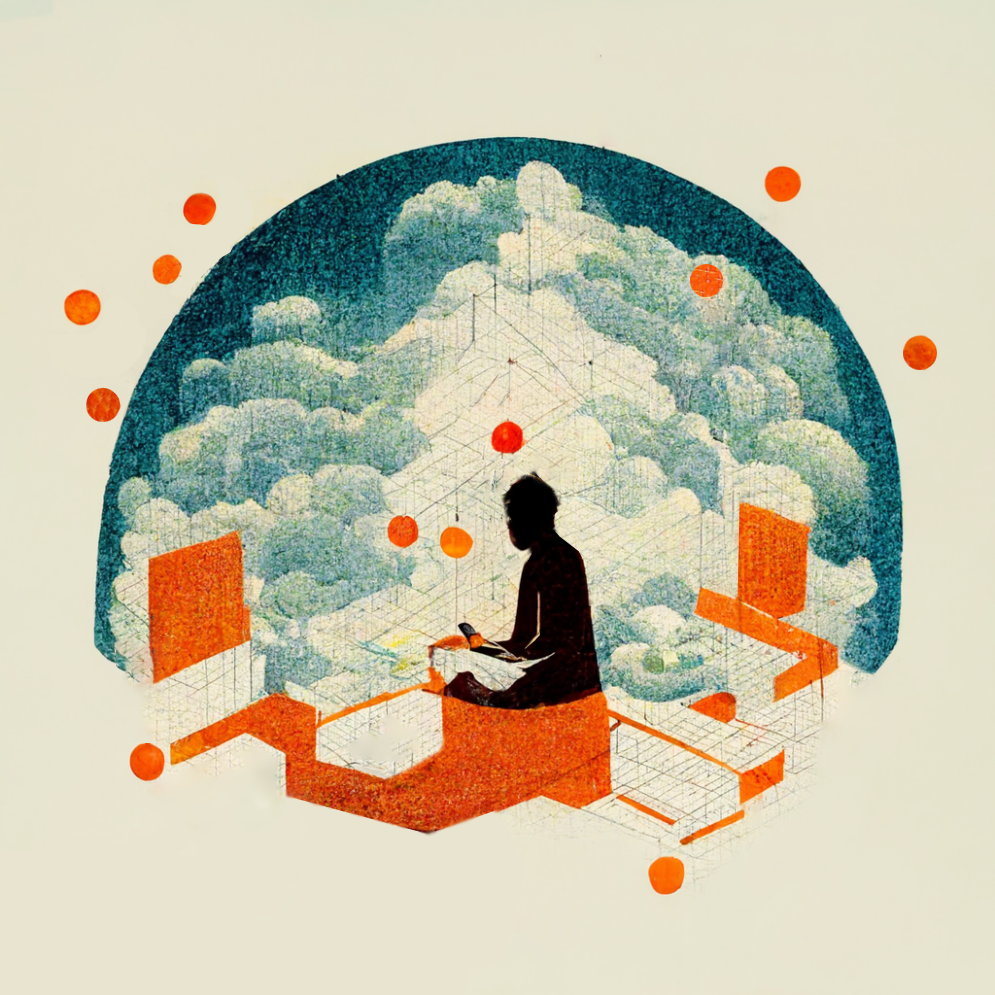
Note: This post is a shortened recap of a virtual talk from Ishan Babbar, Lead Data Scientist at Nayya during Labelbox Accelerate (Nov 2022).
Nayya offers an AI-first solution that helps individuals better understand and choose among their employer-provided benefit plans. Users take a ten-minute survey and get a plan recommendation that best suits their specific needs. Machine learning models work behind the scenes to power decision support tools for a personalized experience that includes financial education and bundled recommendations. To ensure that the plans recommended to customers are appropriate, the organization uses an automated feedback loop between their customer success team and benefit providers to evaluate and explain model-generated recommendations.
One of the main challenges to building performant models is producing all of the labeled data needed for both offline model training and live prediction evaluation, as well as verification by subject matter experts. Data sources used by these models include insurance plans, pharmaceutical data, geospatial data, and financial wellness insights. By building a streamlined annotation workflow with Labelbox's platform and the resulting labeled data (via a feature store) for both tasks, Nayya's ML team was able to keep up with their fast-growing training data needs. "The annotation workflow within Labelbox allows us to do this at scale and build a repeatable process for our data scientists as well as any subject matter experts that work with us,” says Ishan Babbar, lead data scientist at Nayya.
The ML team at Nayya also benefited from having an automated, SDK-driven approach to allow their actuaries (SMEs) to share insights into how the model will generate predictions and evaluate these predictions. “We use Labelbox's Python SDK almost religiously. Part of the main value we get from the platform is being able to interact with how labeling is going, performance quality metrics, and loop that information back to our actuaries and subject matter experts,” says Ishan. The Nayya team is now able to build reproducible pipelines as part of their MLOps infrastructure.
In terms of results, the Nayya team is utilizing Labelbox’s data engine to train models faster through more streamlined labeling and enhanced collaboration. For data that has never been labeled before, the team has set up a strong QA process within the platform to help speed up their efforts when it comes to cataloging and extracting the data from disparate sources. The Nayya team is now able to better visualize this data and use the Labelbox platform to maintain quality, save time, and train and test new models to improve their AI-first products.