Platform
Data curation, AI-assisted labeling, model training & diagnostics, and labeling services, all in one platform, to build better AI products, remarkably fast
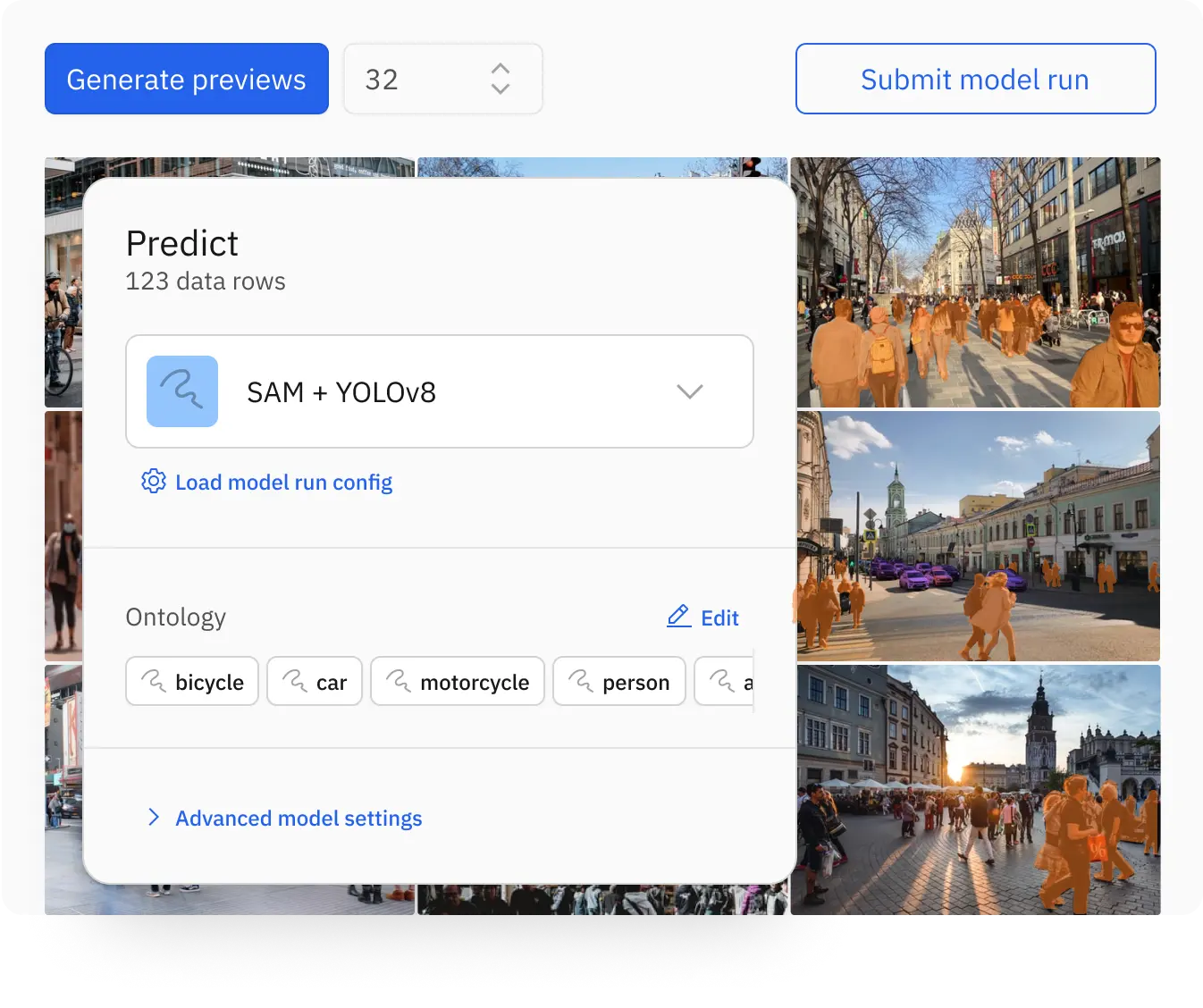
Auto label images and videos
Pre-label data in minutes without code using most capable foundation models or your own models. Model-assisted labeling accelerates data labeling tasks on images or video at a fraction of the typical cost and speed.
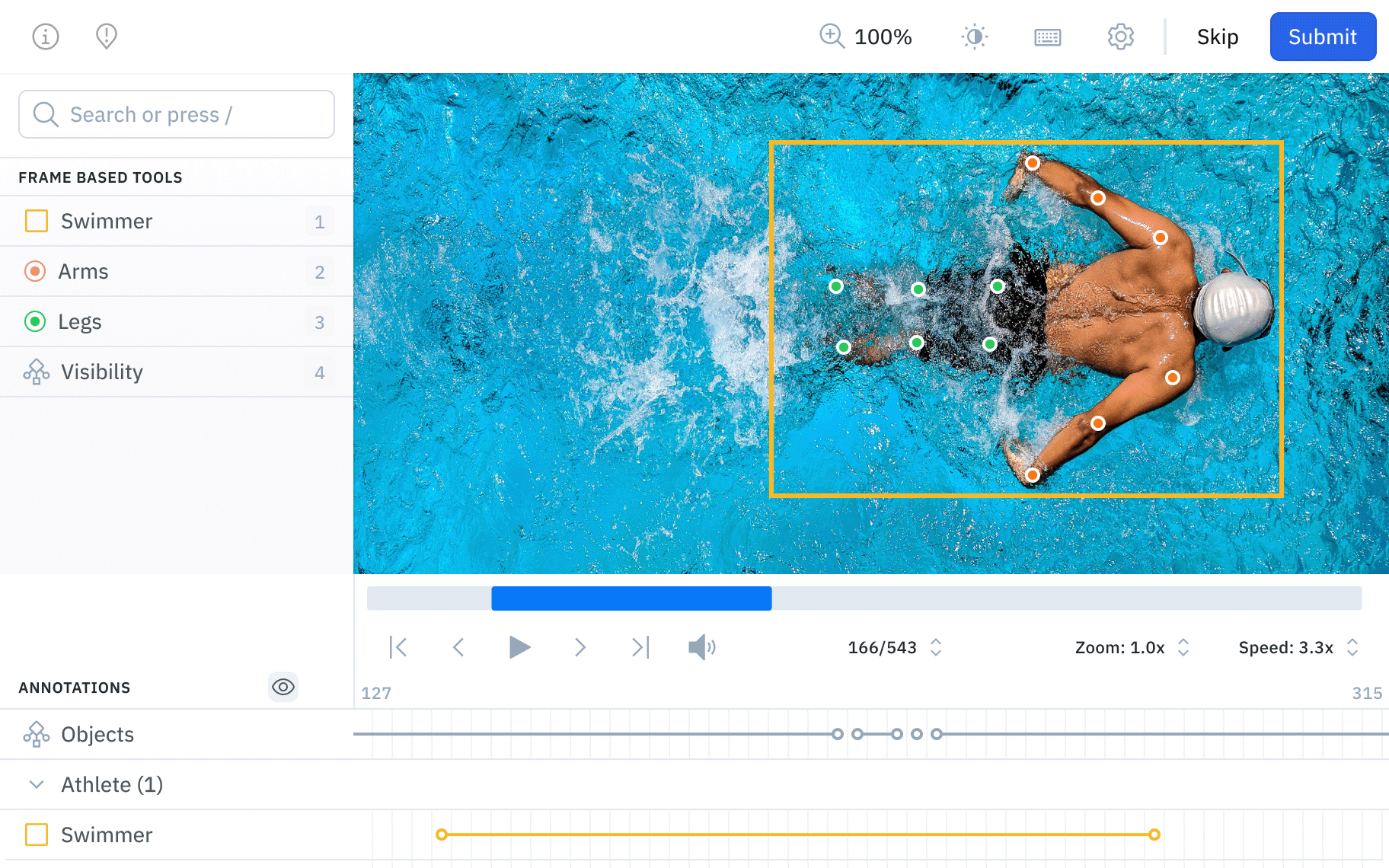
High performance tools for human supervision
Use the same high performance tools to label data with internal teams or simply QA data delivered by Labelbox Boost labeling service, all in the same platform. Labelbox supports common annotations across image, video, geospatial data and even HTML for infinite task possibilities.
Go from an idea to production data stream in days
From rapid prototyping and experimentation to production scale workloads, Labelbox helps AI labs produce more than 50 million monthly annotations across its customers.
Configurable workflows
Labelbox approaches data production similarly to modern factories. It lets you configure multi-step workflows that produce labeled data while connecting AI models and labeling teams.
Rich quality controls boost consistency and accuracy
Use the right tool for quality assurance and finding label mistakes: benchmarks, consensus, comparing ground truth with model predictions, multi step reviews, and AI critic in the loop
AI assisted alignment
AI Assisted Alignment is an approach developed by Labelbox to produce training data by leveraging the power of artificial intelligence to enhance every aspect of the process. From data curation to model-based pre-labeling and catching mistakes or providing feedback, AI assists humans to achieve significant leaps in levels of quality and efficiency.