How a leading e-commerce company continuously improves training data quality for personalizing the shopper experience
Problem
A Fortune 500 e-commerce enterprise needed large quantities of high-quality training data to develop AI applications that improve and personalize the shopper experience. The prior data annotation service that used AI to generate labels consistently failed to meet their training data quality requirements.
Solution
This enterprise adopted Labelbox Annotate and Labelbox Labeling Services for workflow automation and annotation through a software-first approach. They established an efficient system to liaise with their new AI data provider, incorporated model-assisted labeling to accelerate labeling, and prioritized review and feedback.
Result
The enterprise significantly improved AI training data quality and successfully unblocked its AI initiatives. They also increased labeling speed and efficiency by 50% without compromising data quality.
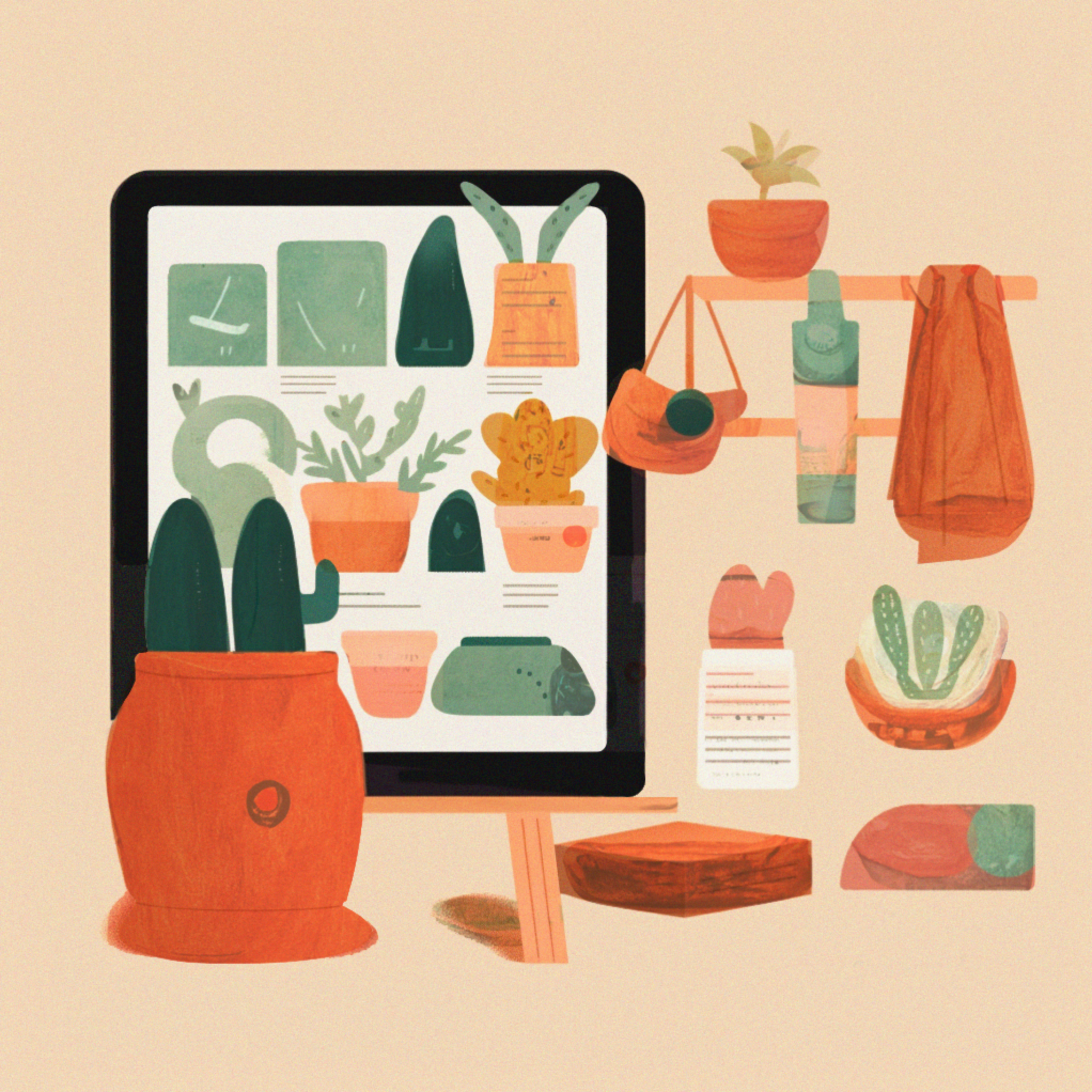
A leading e-commerce company that provides online storefronts for handmade crafts and vintage items planned to launch several AI projects aimed at improving their shopper experience. These image classification and object detection models required large amounts of high-quality labeled data and for their tens of thousands of product SKUs to be correctly tagged. The enterprise’s Search and Recommendation data science team originally enlisted a labeling service that uses AI to generate labeled data quickly. This service, however, consistently failed to meet the team’s labeling quality requirements. As a result, the team switched to Labelbox as their AI data platform, leveraging the core platform to prioritize and label data, as well as finding and working with the right labeling partners for their ML priorities through Labelbox Labeling Services.
As a large business with multiple AI projects and data labeling requirements, the enterprise set up a single point of contact inside its data science team to gather information about each use case, review and approve labeling projects, and transfer approved projects to the Labelbox Services team. With this efficient, streamlined system, teams across the enterprise can now request and get the training data they need systematically with a strong SLA and turnaround time. This approach also enabled the data science team to build a strong working relationship with their labeling operations team, making it easier and faster to meet the enterprise’s training data needs over time.
Labelbox’s Annotate platform provided the enterprise with workflows to automate much of the manual orchestration via the use of a Python SDK. This allowed the enterprise to simplify the data import process from Google Cloud (GCP), which was set up as a core part of their existing data infrastructure. Labels can now be easily pulled and pushed from BigQuery tables for structured data and easily created from the Labelbox and Google Cloud integration. In addition, model training can now be easily integrated by connecting complex training jobs to Google's Vertex AI for optimization.
One of the primary benefits of adopting Labelbox’s software was the focus on automation where it mattered most. For example, the business easily leveraged model-assisted labeling for their object detection labeling needs. The team had one dataset labeled traditionally, used it to train a baseline model, and then used the model’s output as pre-labels for subsequent batches of data labeling. With their own models generating pre-labeled data, the labeling team only had to review and correct the model’s labels, increasing efficiency by roughly 50%, while maintaining a high standard for data quality. As the team iterated on the model and improved its ongoing performance, the time required for labeling training data continued to decrease.
To further boost the quality of their training data, the enterprise prioritized the review and feedback process, also supervised by their data science team. Once the team received labeled data from Labelbox, they evaluated its quality and gave prompt feedback to iterate and fine-tune data that was most impactful for model performance. During ongoing projects, the team would review a sample of labeled data on a weekly basis, creating a reliable feedback loop to evaluate and continuously increase AI data quality. This extra investment helped the labelers improve their work and reduced the iteration time needed to accelerate multiple AI development projects.
With their newly established MLOps practices and continued support and expertise from their Labelbox Services team, the enterprise has significantly improved the quality of their training data, and can now scale their AI development quickly while keeping their operations cost efficient. The algorithms developed, deployed, and maintained with this system empower the enterprise to improve and personalize shopper experiences. In the future, the team is looking to further advance AI development by optimizing their data visualization with Labelbox Catalog and streamlining model training processes within Labelbox Model.