How a Fortune 500 creative tools company shipped generative AI across its products
Problem
This team previously spent many engineering cycles building out their own training data infrastructure for genAI proudcts, which meant project delays and siloed AI development efforts among disparate groups who were looking to find what data was available for ML use.
Solution
Labelbox Catalog & Annotate. Using a variety of native editors and the Labelbox Boost workforce, the enterprise has dramatically streamlined their end-to-end worfklows for identifying and collaborating on genAI training data.
Result
The company’s genAI product development teams have now experienced significant improvements in both efficiency and speed by utilizing Labelbox’s full spectrum of products, resulting in a 50% reduction in labeling operations time and a 5X increase in AI product deployment speed within just 8 months.
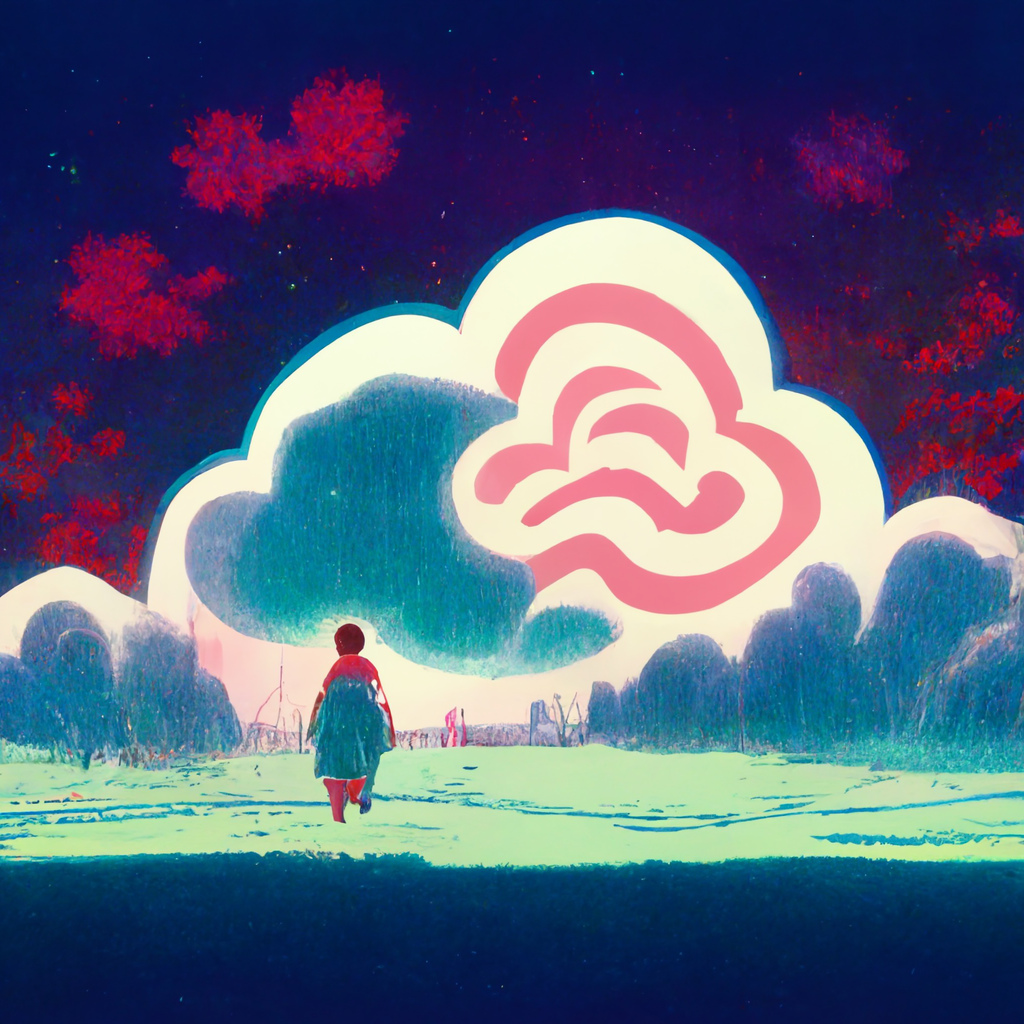
A Fortune 500 software company that provides creative digital marketing and document management solutions was looking for a single platform to consolidate and unify their data labeling efforts for generative AI product development. For many years, the company has been empowering their leading cloud products with AI under the hood, which garnered multiple industry awards and widespread recognition.
A major division of the enterprise’s R&D arm became one of the very first adopters of Labelbox. This team's mandate was to deliver innovation through video understanding via the use of transcripts, joint vision and language, as well as document understanding. This team previously spent a lot of engineering cycles building out their own training data infrastructure, which meant project delays and siloed generative AI development efforts among the disparate groups who were looking to find what data was available for ML use. The team chose Labelbox because it provided a central standardized platform across their entire division for efficiently collaborating between ML & AI teams, as well as their internal and external labeling teams.
The company also found that prior to Labelbox, the process of evaluating the quality of their AI data was a highly fragmented process. This led to lower confidence in their models, with AI/ML initiatives taking much longer than expected to yield a return on their investments. To alleviate this problem, the company leveraged Labelbox for a variety of their primary data modalities - which included text, PDF, images and videos - and tied the process of finding unstructured data with their core annotation process.
Labelbox Catalog provided the ability to quickly leverage metadata and custom embeddings for filtering all of this unstructured data, saving the team hours of time every day for a process that would have taken months of custom engineering work. The customer was then able to leverage Labelbox Annotate to develop a consistent process for curating high-quality AI data. Using built-in quality assurance tools, the team quickly saw increases in model performance, specifically for their NLP and computer vision projects which focused on understanding the complex structure of PDF documents.
Using Labelbox’s Annotation suite for collaboration, multiple data science teams and product teams are now able to more effectively work with internal stakeholders to define what data they are interested in, and to translate requests into specific labeling instructions, while evolving project ontologies as business needs change.
The company’s product development teams have now experienced significant improvements in both efficiency and speed by utilizing Labelbox’s full spectrum of products, resulting in a 50% reduction in labeling operations time and a 5X increase in AI product deployment speed within just 8 months. Their AI Assistant products, which provide a comprehensive understanding of PDF structure and content, have been released to production in 2023, enhancing quality and reliability. By integrating Labelbox's unified platform with a specialized workforce through Labelbox's Labeling Services, the company can also now process tens of thousands of PDFs using a dynamic queueing system, prioritizing labeled data to enhance generative AI outputs.