How a leading education technology company utilizes greater transparency and control to develop their training data
Problem
Due to rapid growth during the pandemic, the enterprise found that the sheer number of annotated text data they needed to train their ML models was not scalable given their previous solutions using Amazon Sagemaker and Prodigy.
Solution
Labelbox's collaborative text annotation capabiltiies, along with advanced user permissioning and QA tools.
Result
The education technology company was able to rapidly deliver the hundreds of thousands of annotations needed for their ML models in record speed, while tracking both productivity and training data quality.
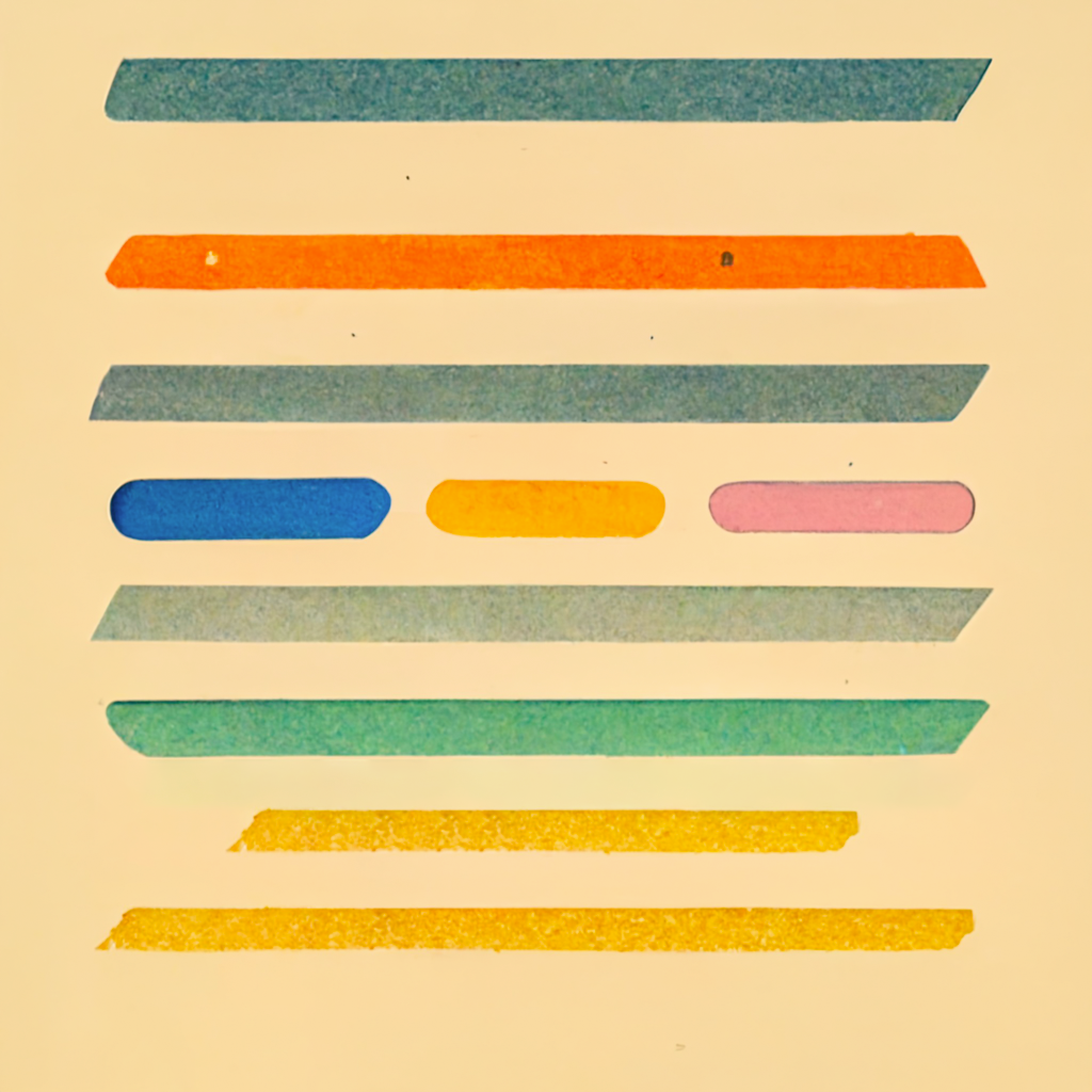
A leading education technology company previously used services such as Amazon SageMaker GroundTruth and Prodigy while also having an in-house built tool for labeling all their text data. Due to rapid growth during the pandemic, the company found that the sheer number of annotated text data needed to train their models was not scalable given their current solutions.
The company turned to Labelbox platform, as well as Labelbox's Workforce team, to start the process of labeling hundreds of thousands of text data for their ML models. Students answering questions from their software were typically done in the form of screenshot images, which meant needing to convert OCR data back into text data so that experts can more effectively annotate them. The enterprise also built an in-house comparison system within Labelbox for measuring the accuracy of different OCR labels. Labelbox was able to more easily scale with this enterprise, while going beyond basic text labeling capabilities because of advanced user permissioning management, text catalog dataset management, as well as quality assurance using consensus tools.
Three months into their project, the education technology company is now benefiting from the enhanced visibility that Labelbox provides. In their words, "tracking productivity and quality in other services felt more like a black box because after submitting responses, there was nothing else that we could do. In contrast, Labelbox provides the ability to count the number of labels done, revisit submitted labels, fix errors, run a full quality assurance pipeline and manage labeler productivity."
AI and ML is also now able to make the complex question-answering process smoother and also help speed up the answering process for experts. The streamlined workflow helps populate data fields faster and make better question/answer recommendations, as well as ultimately boost student learning and outcomes.