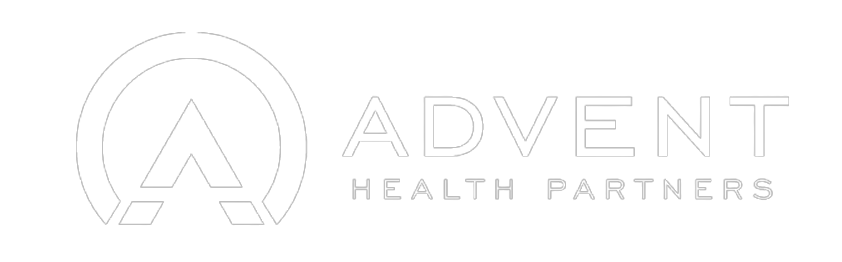
How Advent Health Partners uses active learning and automation to quickly process medical records
Problem
Extracting data from paper copies of millions of medical records to train ML models is a time consuming and expensive process because these records can be regularly over five hundred pages if not thousands of pages in length. These records typically come from numerous different providers, hospitals, insurance companies, with no consistent formatting and contain image and text files of varying formats such as PDFs, faxes, scans, etc.
Solution
The AHP team leveraged Labelbox’s platform for automation and active learning, which gave them a rapid way to evaluate the accuracy of entity extractions using a combination of labeling vendors and in-house experts for human review.
Result
The AHP team is now able to dramatically speed up the process of processing medical records for training their ML models through easier collaboration and better QA for their labeled data. Classifying each page in the past typically took labelers around 13 seconds. By leveraging active learning and automation techniques such as model-assisted labeling within Labelbox, the AHP is now able to reduce the average time per label to just 8 seconds.
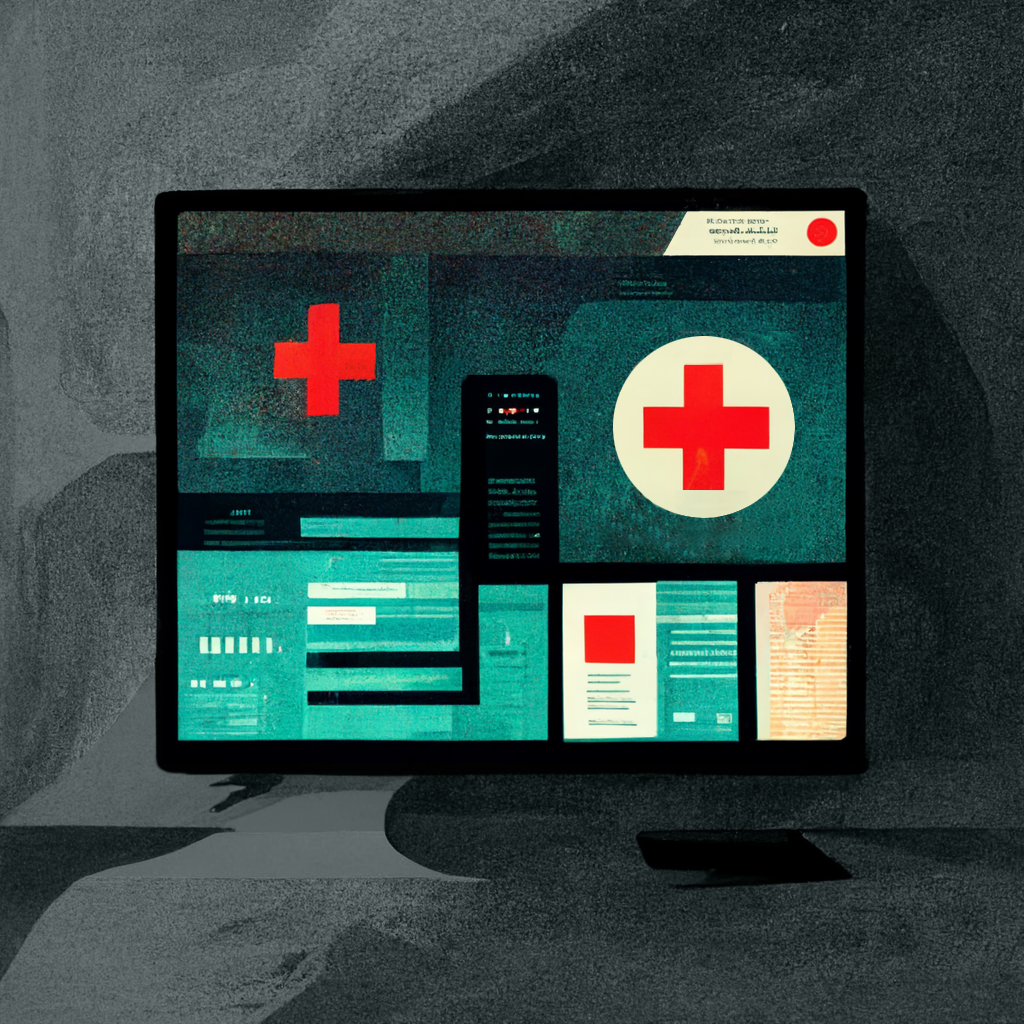
Note: This post is a shortened recap of a virtual talk from Robert Coop, Chief AI Officer at Advent Health Partners during Labelbox Accelerate (Nov 2022).
Advent Health Partners (AHP) is a health care service and technology company focused on efficiently driving healthcare reimbursement and offering proprietary medical record review technology. One of their flagship AI-powered products is the CAVO software platform which delivers an interactive interface for medical record review used by both insurance and payers. The AHP data science team is focused on leveraging the latest advances in optical character recognition (OCR) on these medical records and applying AI through the use of natural language processing (NLP) in the form of entity extraction.
Extracting data from paper copies of millions of medical records is a time intensive and laborious process because these records are regularly over five hundred pages if not thousands of pages in length. Furthermore, these records typically come from numerous different providers, hospitals, insurance companies, with no consistent formatting and contain image and text files of varying formats such as PDFs, faxes, scans, etc. The AHP data science team’s primary goal is to analyze and feed this vast amount of information into their clinical machine learning models for focused review, and to examine whether or not the documentation has all the necessary elements to justify the course of treatment that a patient took.
To reduce the friction required of turning unstructured data into valuable AI data, the AHP data science team employed Labelbox’s data engine which gave them a faster way to evaluate the accuracy of entity extractions using a combination of labeling vendors and in-house experts for human review. Their team was then able to quickly build a page classification model where they took paper records and automatically tagged emergency department records, discharge notes, and types of documents that human reviewers can check within the Labelbox interface and review for accuracy.
The AHP team also utilized a series of active learning workflows to find areas where their classification models didn’t have high enough accuracy. Their in-house team of domain experts would review both confident model predictions to prevent errors and utilize uncertainty sampling. As they set out to build a platform that extracted information from medical records to help hospitals, insurance companies, and other organizations process claims, appeals, and payments faster and more efficiently, they had to train their AI on new classes.
To sample data for the project, they evaluated their existing model over unlabeled samples and calculated the entropy of the output classification vector. The AHP team grouped these calculations into two buckets: one with low entropy (meaning where the model was confident on this data), and one with high entropy (where the model had low confidence). The team could then more easily sample data from these two groups, taking only 5-10% of their data from the low entropy group and the rest from the high entropy group, to train their ML models on these new areas.
Once they trained the model on this dataset, the team realized that they had a significant class imbalance. One class was 25% of the dataset, while the least represented classes were only about 7% of the dataset. To correct this, they used the earlier version of their model to create a semi-supervised model that would balance the classes within their unlabeled data which resulted in better model performance.
In terms of the immediate results from adopting Labelbox, the AHP team is now able to dramatically speed up the labeling process of medical records for training their ML models. Classifying and annotating each page in the past typically took labelers around 13 seconds. By leveraging active learning and automation techniques such as model-assisted labeling within Labelbox, the AHP is now able to reduce the average time per label to just 8 seconds. “By using Labelbox's model-assisted labeling workflows, we have been able to cut a full 25 hours off of the amount of time for each specific labeling task, and we’ve found that our labelers have an easier time through a software-first approach,” said Robert Coop, Chief AI Officer at Advent Health Partners.