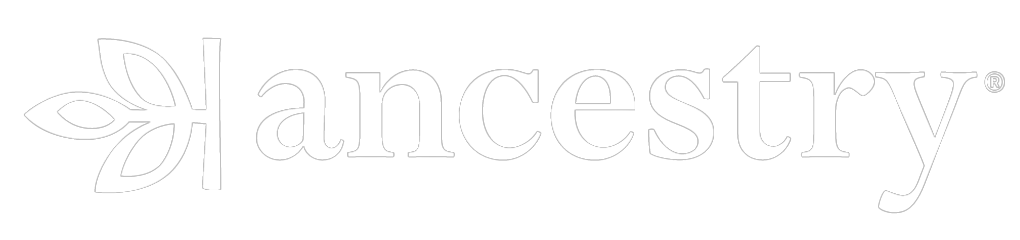
How Ancestry prioritizes collaboration and training data quality to enable genealogical breakthroughs with ML
Problem
Ancestry's data science team was looking for more efficient ways to decode census data and to train their ML models faster, shifting from solely building models to taking a more data-centric approach and finding ways to optimize their entire MLOps pipeline.
Solution
The Ancestry team adopted Labelbox’s Annotate product, leveraging the latest in labeling automation and workflow collaboration including model-assisted labeling, annotation relationships, as well as Boost labeling services.
Result
The team is now able to better contextualize their historical data by using Labelbox's data engine to maintain training data quality, save time when collaborating with domain experts, and train and test new models in record time.
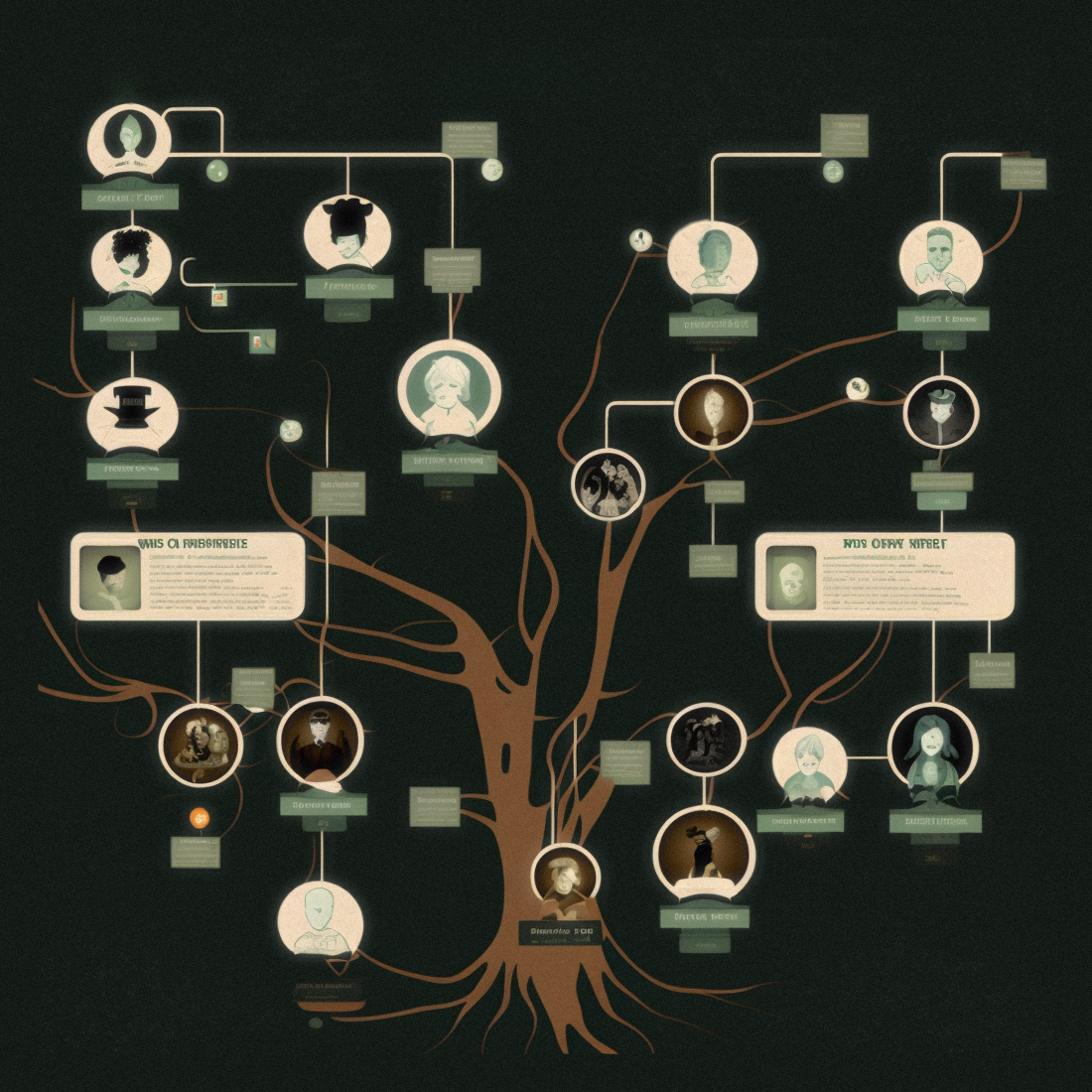
Note: This post is a shortened recap of a virtual talk from Stanley Fujimoto, Data Scientist at Ancestry during Labelbox Accelerate (Nov 2022).
Ancestry is a leading genealogy company that combines billions of rich historical records, millions of family trees, and samples from their AncestryDNA network. Their data science team focuses on leveraging the latest techniques in neural networks and transformers to extract genealogical data from historical artifacts. The team was looking for more efficient ways to decode census data and to train their ML models faster, shifting from solely building models to taking a more data-centric approach and finding ways to optimize their entire MLOps pipeline.
Prior to Labelbox, Ancestry’s data scientists would typically own some of the core labeling task from start to finish. When they didn't write labeling specifications themselves - they discovered that while their experts had strong domain experience - they would have less insights about how their models worked. Finding easier ways to involve their domain experts during the labeling and review process was essential because it helped unlock the insights of these subject matter experts who have a wealth of knowledge in looking at historical documents in order to prioritize what unstructured data needed labeling.
As a solution to this, the Ancestry team adopted Labelbox’s Annotate product and working within the native image and text editors, the team was now able to leverage the latest in labeling automation and collaboration including model-assisted labeling, annotation relationships, as well as Boost labeling services.
“Before Labelbox, we could train a model pretty quickly and evaluate against validation test sets, but getting data labeled and reviewed took forever. Having a strong collaborative annotation platform helped us get to a weekly iteration cycle”, said Stanley Fujimoto, Data Scientist at Ancestry.
The ability to evaluate how labelers were annotating data with analytics and in-depth metrics further helped the Ancestry team to be able to communicate in real-time and correct labels as needed. The Ancestry ML team found that having a dedicated platform that enables data scientists to easily raise questions and issues for annotating images and text in PDF documents at scale has also been incredibly helpful.
In Stanley’s words, “our team is able to collaborate more efficiently by dropping a pin on any image [asset], where we’ll have a question and we can respond to our labelers in line. For other platforms, the labeling process is a complete black box as we have to wait for all of our labels to come back before we can review and give any feedback. We've found that writing labeling specs always includes some level of ambiguity. For that reason, clarification and iteration speed is essential.”
To gain momentum towards their goal of weekly model releases, the Ancestry team is utilizing Labelbox’s data engine to train models faster and evaluate validation test sets more easily. For data that has never been labeled, the team has set up a strong QA process within the platform to help speed up their efforts when it comes to extracting the precise location of historical records, and then performing analysis such as handwriting recognition on samples. The Ancestry team is now able to better contextualize their data and use the Labelbox platform to maintain quality, save time, and train and test new models in record time.