How a Fortune 500 shipping and logistics company uses AI to optimize package deliveries
Problem
The enterprise's data science team needed to organize and produce high-quality labeled image data in order to train their ML computer vision models. The team did not possess dedicated software for visualizing all of their unstructured images, nor did they have scalable in-house labeling capabilities.
Solution
The enterprise utilized Labelbox Annotate, Catalog and Boost to prioritize their object detection use cases, quickly classifying truck interiors and package fill rates while sorting based on important attributes (metadata) such as camera number, date & time, and trailer unloading process.
Result
By labeling a small number of initial images based on a specific trailer unloading process inside of Labelbox, and then importing these labels over high-volumes of data (hundreds of thousands), this automation workflow saved their team approximately 50% of time and costs, while reducing workloads by thousands of hours.
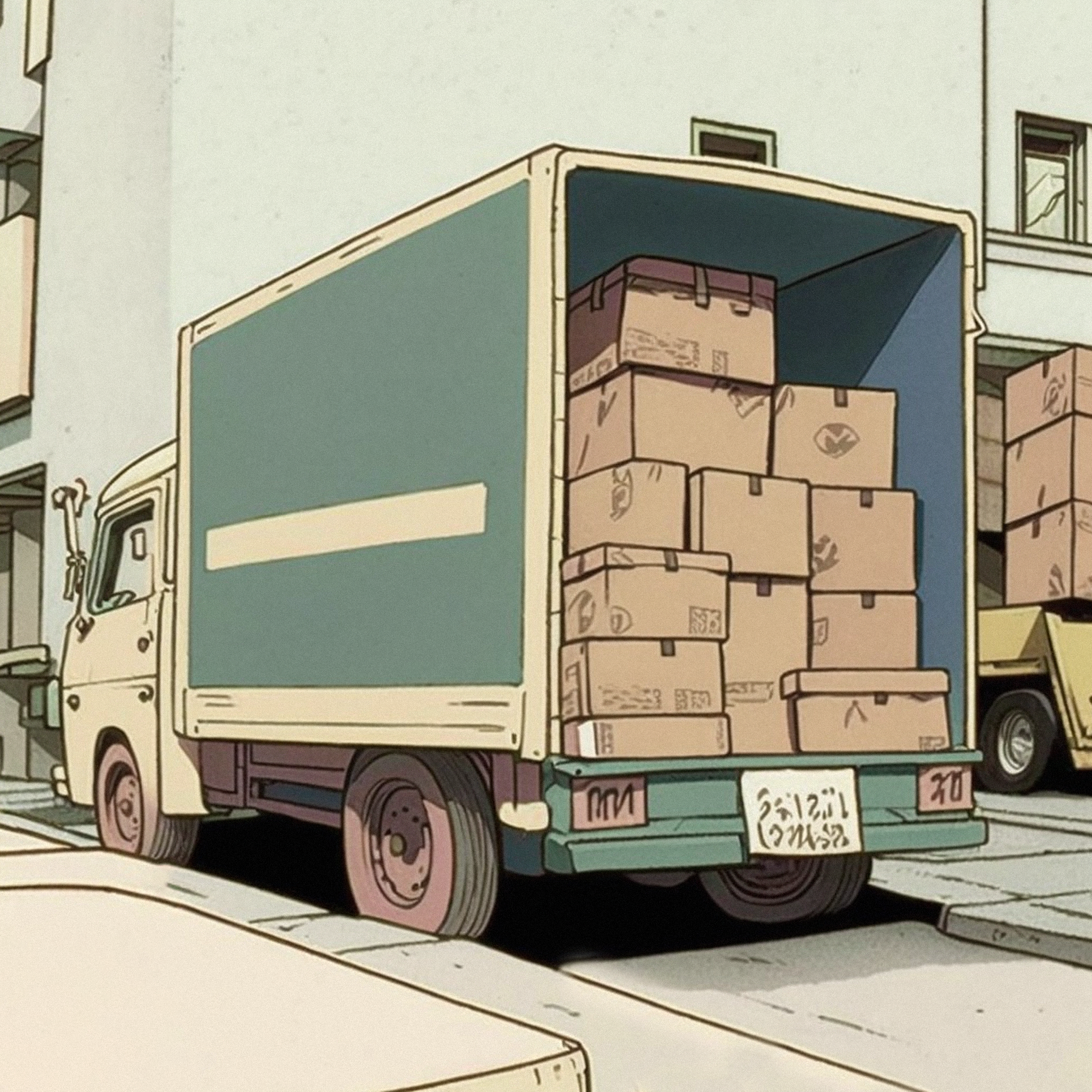
A Fortune 500 multinational shipping and supply chain management company wanted to leverage machine learning to assess the state of packages inside the interiors of their shipping vehicles. By determining the fill levels of these trucks using the latest advances in AI, the enterprise could then most effectively maximize the volume of packages that they can ship on a recurring basis as well as optimize the trailer unloading process.
However, as a commonly experienced bottleneck, their data science team did not have the ability to produce all of the high-quality labeled data needed in order to train their ML computer vision models. Additionally, the team did not possess scalable in-house labeling capabilities and was seeking dedicated software for visualizing all of their unstructured images in a unified place. This would allow them to to prioritize what to label, and more easily find edge cases and skip duplicates.
To solve this, the enterprise chose Labelbox to serve as an agile platform that could provide effective annotations and allow them to visualize what data was available to annotate in order to help them launch production-ready models in a matter of five months. Their data science team utilized Labelbox’s Annotate and Catalog products to prioritize their object detection and bounding boxes projects, quickly classifying truck interior packages and sorting them based on important attributes (metadata) such as camera number, date/time, and trailer unloading process.
In terms of immediate results, the data science team worked with Labelbox Boost to strategically preprocess their image data in the form of importing baseline predictions. By labeling a small number of initial images based on a specific trailer unloading process inside of Labelbox, and then importing these same labels over high-volumes of data (hundreds of thousands), this automation workflow saved their team by approximately 50% of time and costs, reducing their workloads by thousands of hours.
The team is planning to expand on their use cases with additional computer vision projects including robotics for sorting and identifying packages, and finding ways to automate many more of their manual and auditing processes with the help of innovative machine learning systems.