Labelbox•July 16, 2021
3 Key takeaways from Labelbox Academy: Mastering the platform
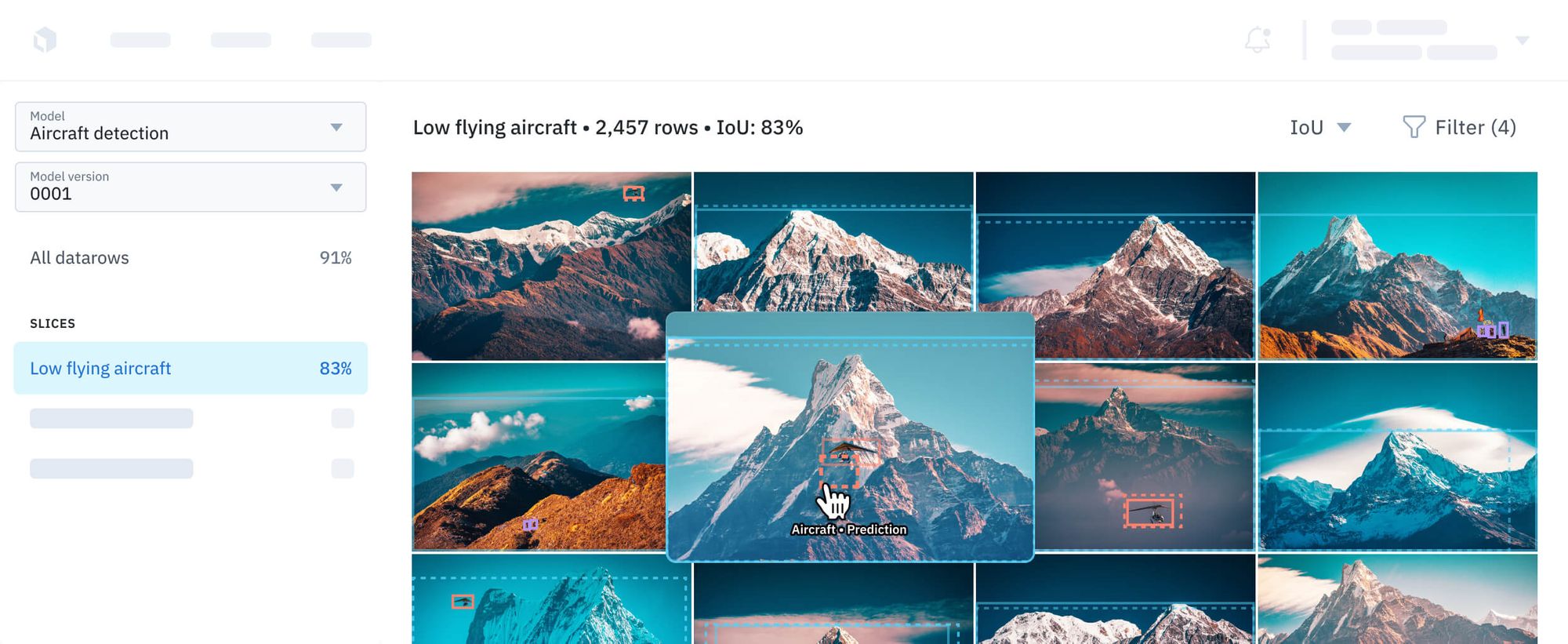
Our second Labelbox Academy event took place on June 30th, 2021, and covered some of the more complex capabilities available with our training data platform, along with best practices and the philosophy behind these features. Read on to learn three important takeaways from the event.
Retrain your model with MAL
Since Labelbox released its Model Assisted Labeling (MAL) feature, many of our customers have used it to reduce human effort and costs over time. The Accelerate your pipeline with Model Assisted Labeling session included a detailed demonstration of how an ML team can use the feature to bring a model’s output back into Labelbox, correct its labels, and retrain it to achieve higher accuracy.
The session also featured ImageBiopsy Labs’ Senior ML & AI Engineer Caroline Magg, whose team used MAL to sort large datasets of medical imagery. They labeled a batch of data and trained their first model, which generated predictions on a second batch of data that the team also needed to annotate. The output was then corrected using MAL in Labelbox, creating a larger, more enriched dataset for the team.
“Once you finish the first iteration, the wheel is spinning,” said Magg. “We were able to double the amount of data we could handle in a given time with Model Assisted Labeling.”
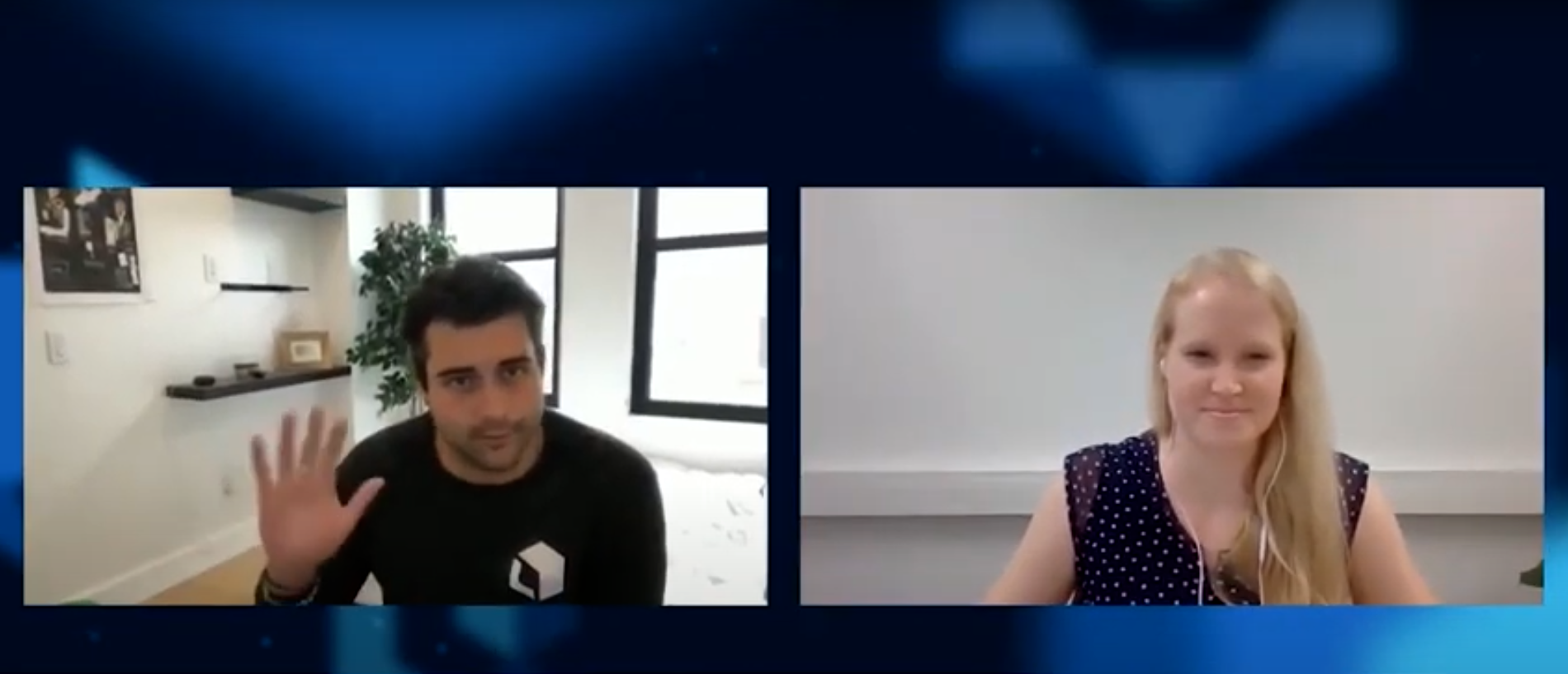
Misconceptions about labeling operations
The event also included a session about best practices in labeling operations. Led by Labelbox COO and Cofounder Brian Rieger and Labelbox Director of Labeling Operations Audrey Smith, the session included a discussion of common misconceptions surrounding the subject.
- Labeling teams will produce top quality annotations immediately. In reality, labels will increase in quality by 30% after the first few days or weeks, depending on the complexity of the task and the quality of the instructions that labelers were given. Like all of us, labelers need time to learn the specifics of their task.
- Labeling instructions and ontology stay the same throughout a labeling project. The best labeling workforces will usually come back to the ML team with questions and ideas about how to do the job better or more efficiently — and the instructions and ontology should be iterated on to reflect these ideas. Communication between the ML team and the labeling workforce should ideally be a two-way street, with both sides learning and improving the process.
- Teams should iterate on models, not the labeled data. Actually, iterating on training data will produce better results. Improving the way your data is labeled can often correct model errors and low confidence zones better than changing the model itself.
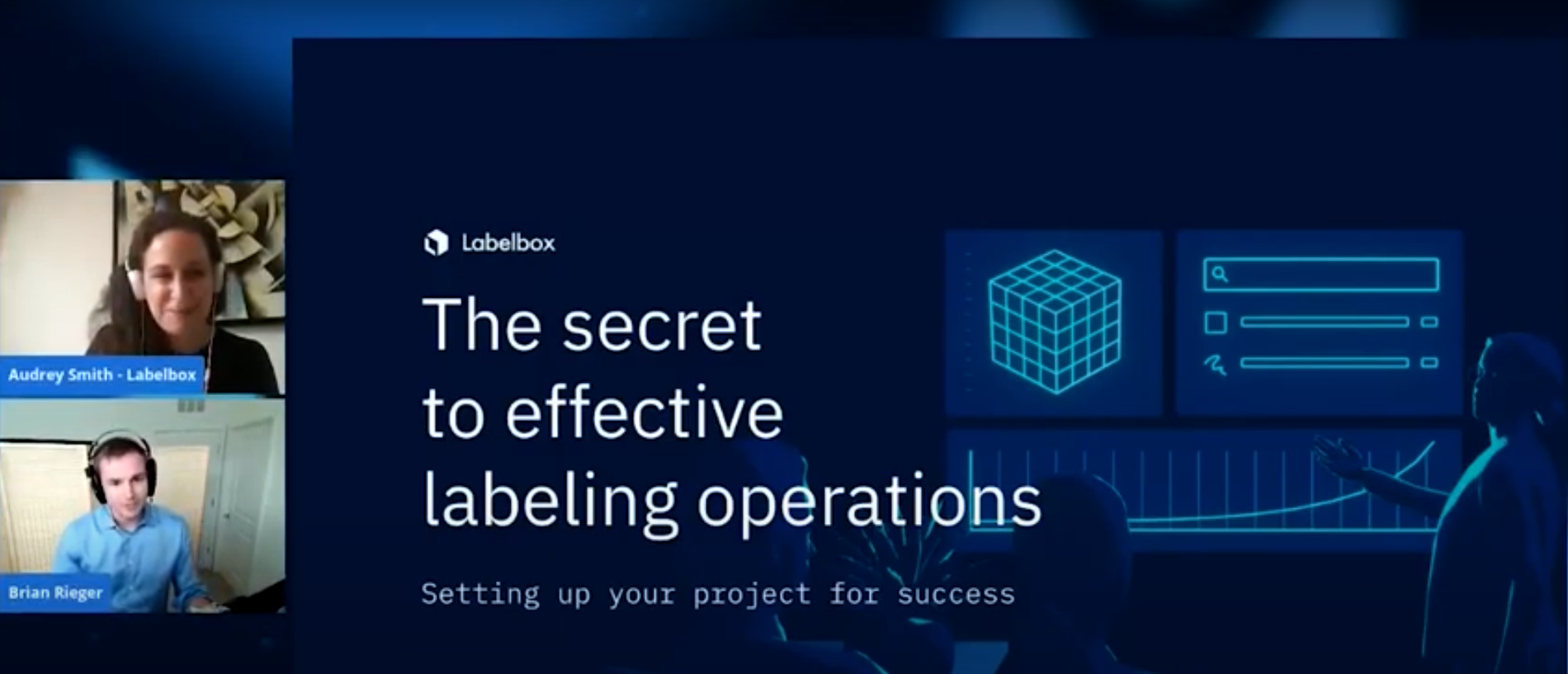
Increase observability in ML
In software development, there are plenty of tools and services that allow teams to monitor their product’s performance by providing traces, logs, metrics, and dashboarding and other investigation tools. ML teams, however, currently lack these resources. In the Monitoring the performance of deployed models session, Labelbox Product Manager Gareth Jones and ML Engineer Matt Sokoloff discussed three primary challenges facing ML teams that want to monitor their models:
- Trust. ML models today are often “black boxes." The people using them don’t usually understand what they do or how they work. Scaling any product requires the team to know everything about the model and how it’s used to ensure quality and consistency.
- Integration. Any performance monitoring solution should integrate seamlessly with a team’s existing ecosystem of ML tools. Tools that are isolated from the rest of the ML workflow are less likely to be completely accurate, and can be frustrating to use.
- Iteration. ML models face a much slower iteration cycle than traditional software. Because of this, any model errors need to be caught as soon as possible in the training process, so that teams have time to address them.
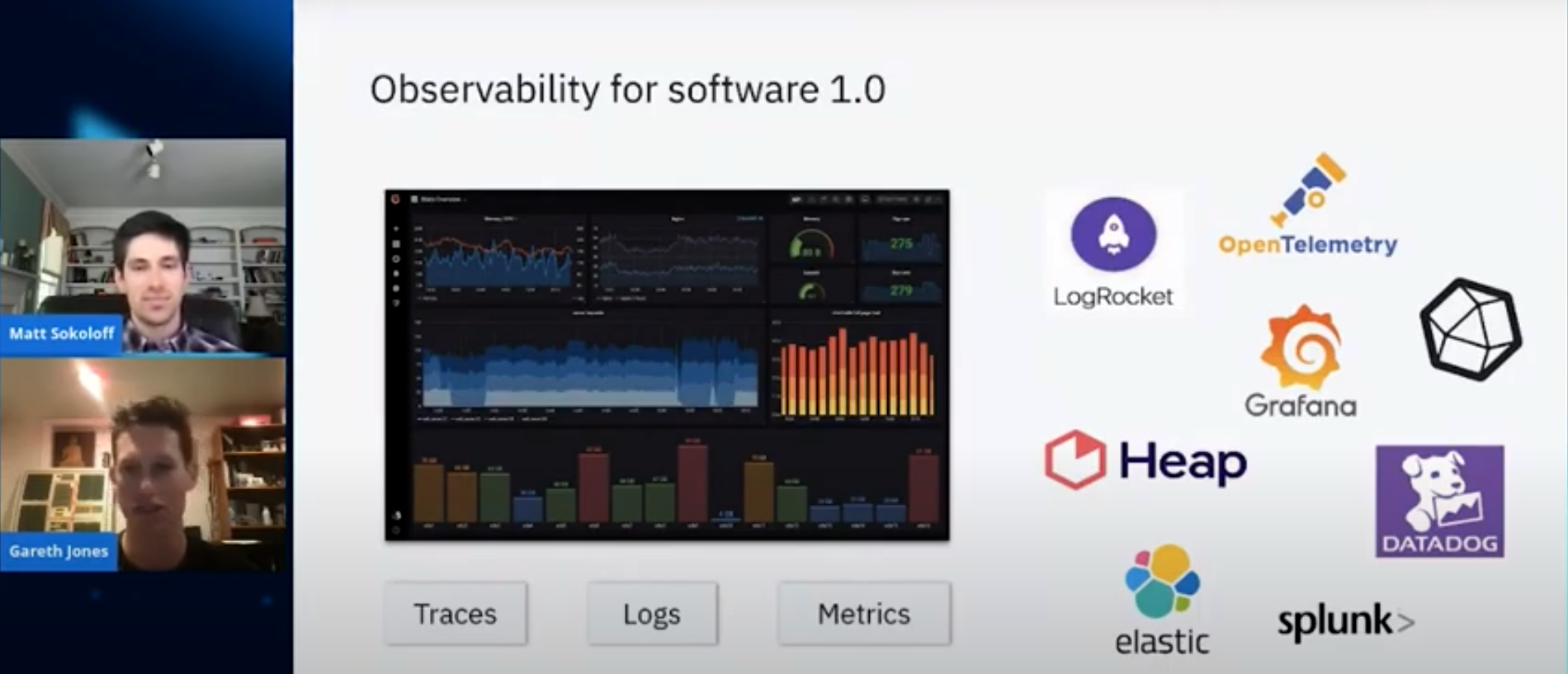
If you’d like to watch any of the sessions from Labelbox Academy: Mastering the platform, please contact us at hi@labelbox.com or send a request through the chatbot on this site. You can also learn more about MAL, labeling operations, and Model Diagnostics here on labelbox.com.