Labelbox•October 24, 2020
Automate labeling with your own model
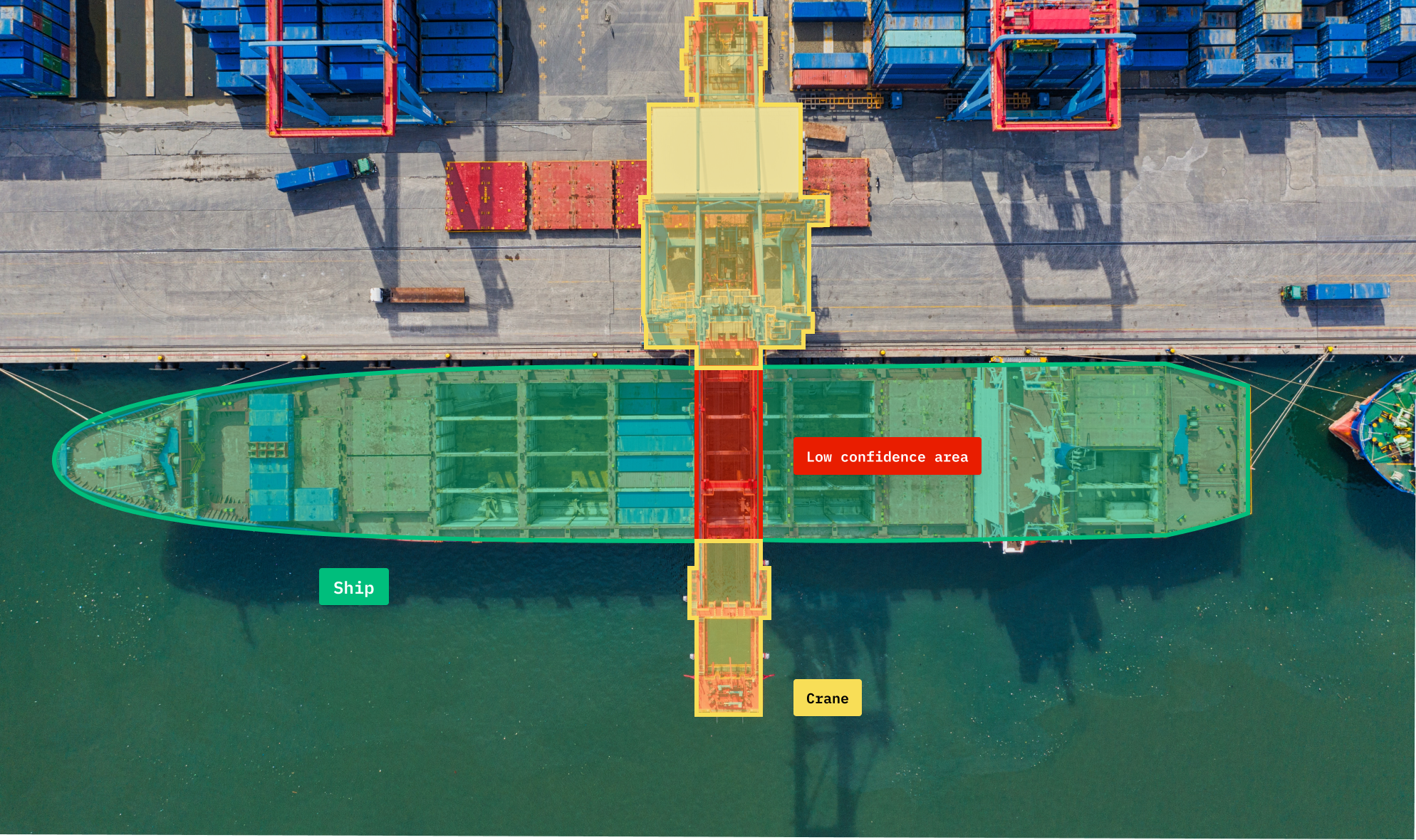
Model-assisted labeling from Labelbox is a powerful way to make your labeling easier, more accurate, and faster by using your own model to pre-label data. This fundamentally changes the labeling process by letting your labeling teams focus their time on refining, accepting, or rejecting model predictions rather than starting from scratch with each label. Labelbox customers using model-assisted labeling have seen a savings of 50-70% in terms of annotation costs.
Without model-assisted labeling, labeling costs increase linearly with each additional human annotation, but model performance increases with diminishing returns because your model is learning to identify common examples with fairly high accuracy.
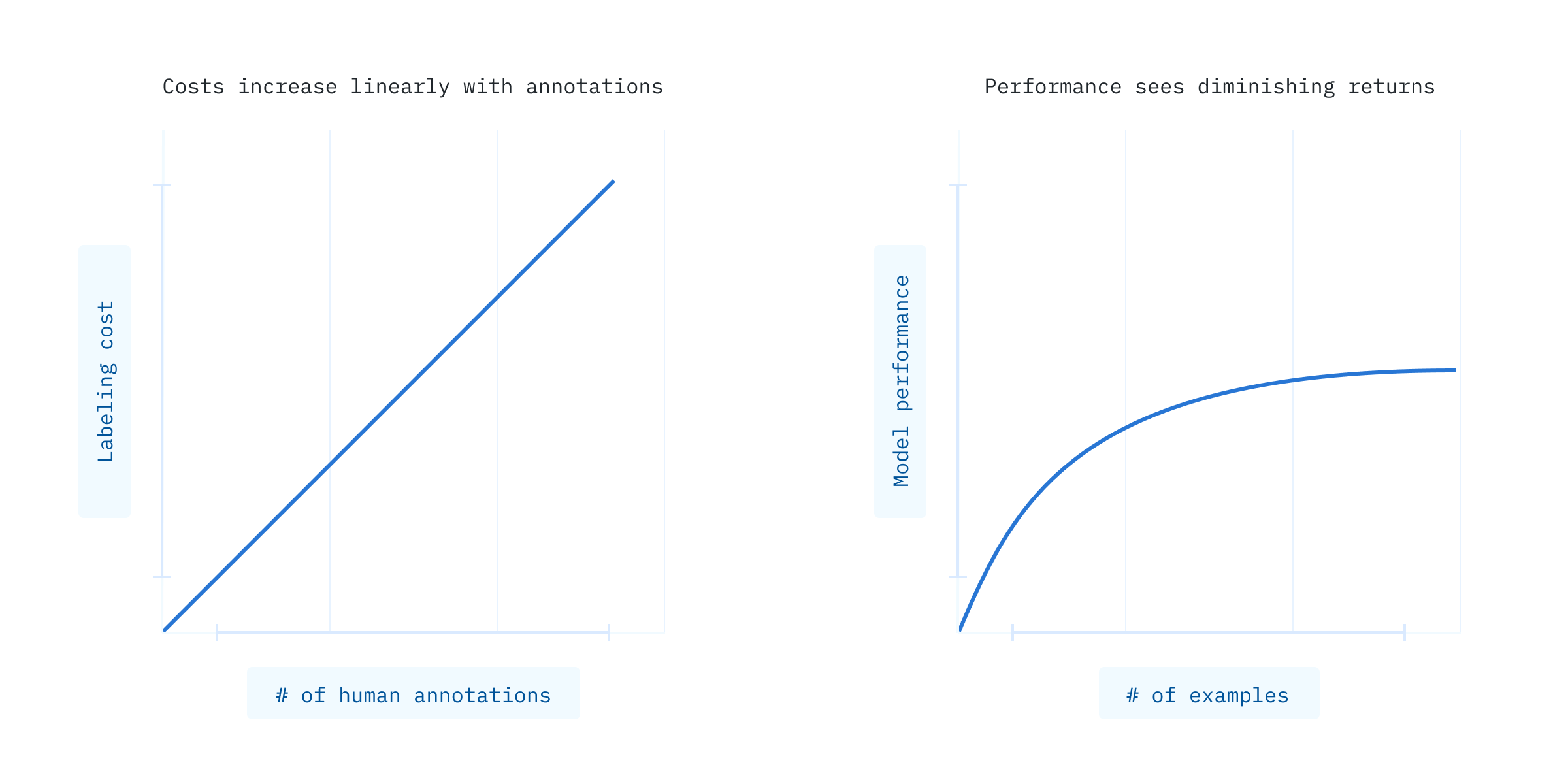
As you continue to iterate and drive model performance higher, you can use your own model to automate labeling with more accuracy than an off-the-shelf model trained with a more generic dataset. Because you’re using your own model to automate labeling, labelers can simply review and accept or refine high-confidence predictions. This also lets you guide labelers to low-confidence predictions where they can apply the most impactful annotations for improving model performance.
Using off-the-shelf models trained on public datasets can, depending on the domain, be useful in the earliest stages of model development to test data modalities and model architectures. However, as the complexity of your model increases and you start needing more domain-specific training data, you’ll see a limit to such a model’s ability to drive performance improvements.
Labelbox is designed to help bring your models to production as quickly and efficiently as possible, and we believe there’s no better way to do that than by using your own model. There are a number of ways to apply model-assisted labeling, and one of the simplest methods is to accelerate model iteration cycles by focusing labeler time where it’s needed most.
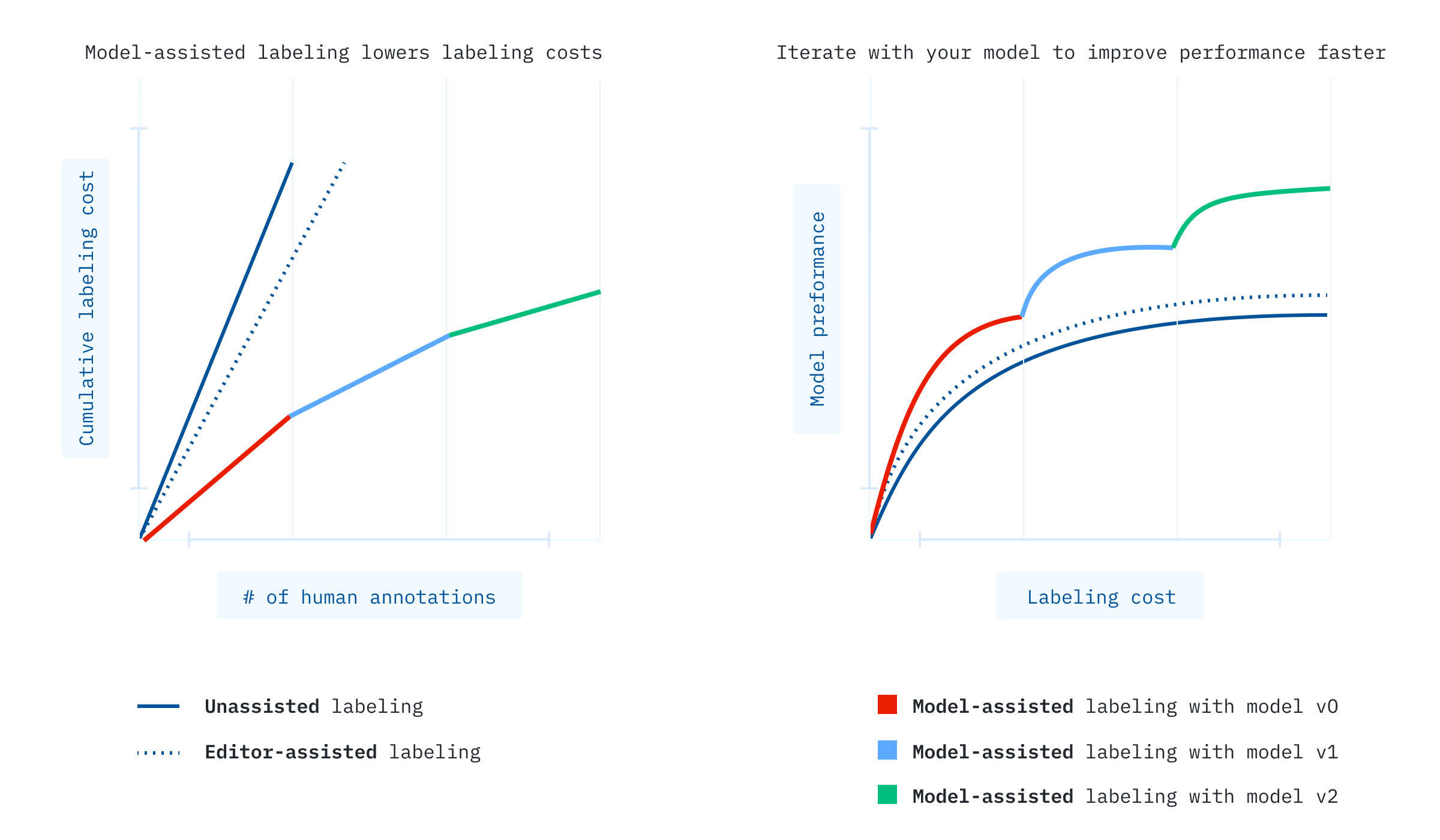
As your model predictions improve in accuracy and confidence, labeling costs will fall on a per-asset basis, leading to a slower accumulation of total labeling costs. Labelbox customers using model-assisted labeling have seen 50-70% reductions in labeling costs driven by dramatic reductions in labeling time and complexity. Labelers can spend less time creating annotations from scratch, and are free to spend their time accepting and refining labels. Not only is this a faster and simpler workflow, it’s often higher quality because of the reduced cognitive load on labeling teams.
Model-assisted labeling is one of the best ways to train your model more efficiently. Rather than using brute force and labeling more and more data by hand, you can guide human labeling with intention to where it’ll drive the most impactful performance improvements. Labelbox makes every step of the process intuitive and quick to set up, letting you get straight to training your model with one of the most powerful, domain-specific tools available: your own model.