Solutions Accelerator
Personalized Experiences
Leverage end-to-end workflow to build the best personalized product recommendations engine
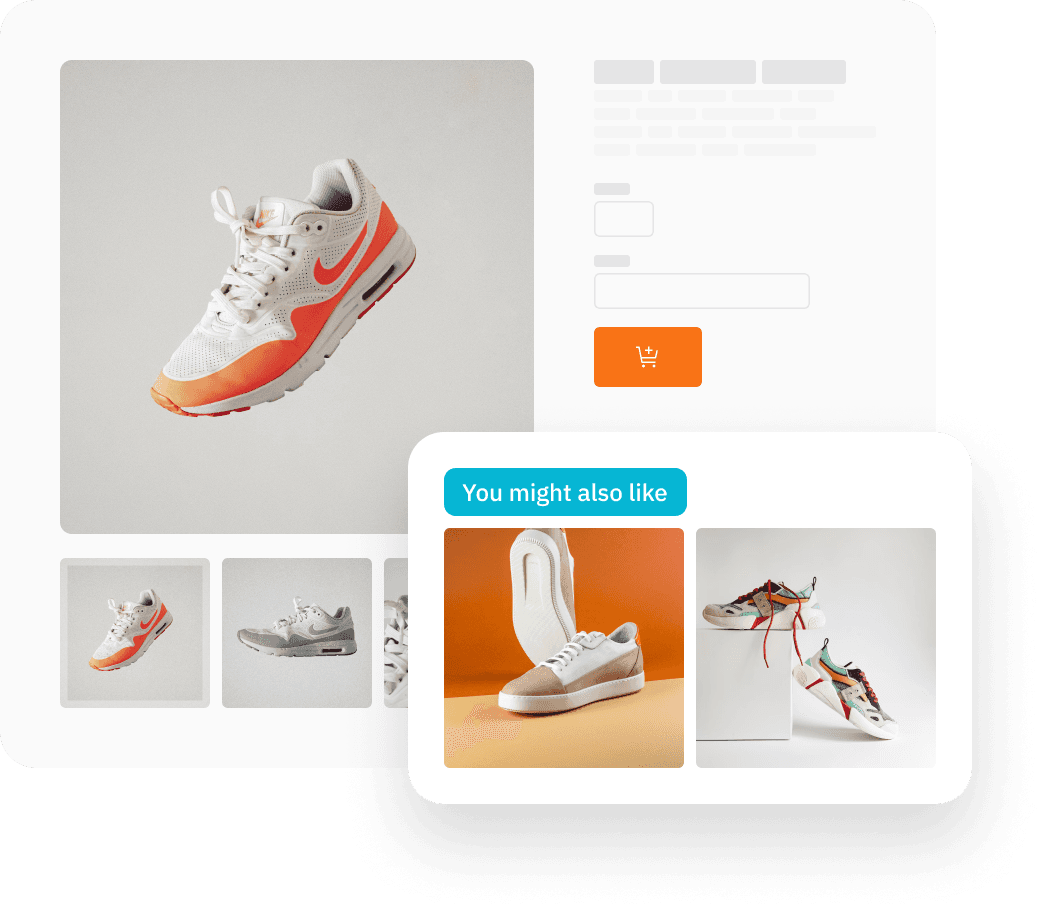
How it works
Data sources
Product images
Listings information
Data-centric AI platform
Explore
Natural language search
Similarity search
No-code search by listings info
Enhance
Bulk classification of listings into categories
Prepare
Dynamic, saved searches
Versioned, explorable listings classification dataset
Evaluate
Data-centric model performance metrics
Corner case detection
Output
Product categorizer model
Enriched listings
Foundation models
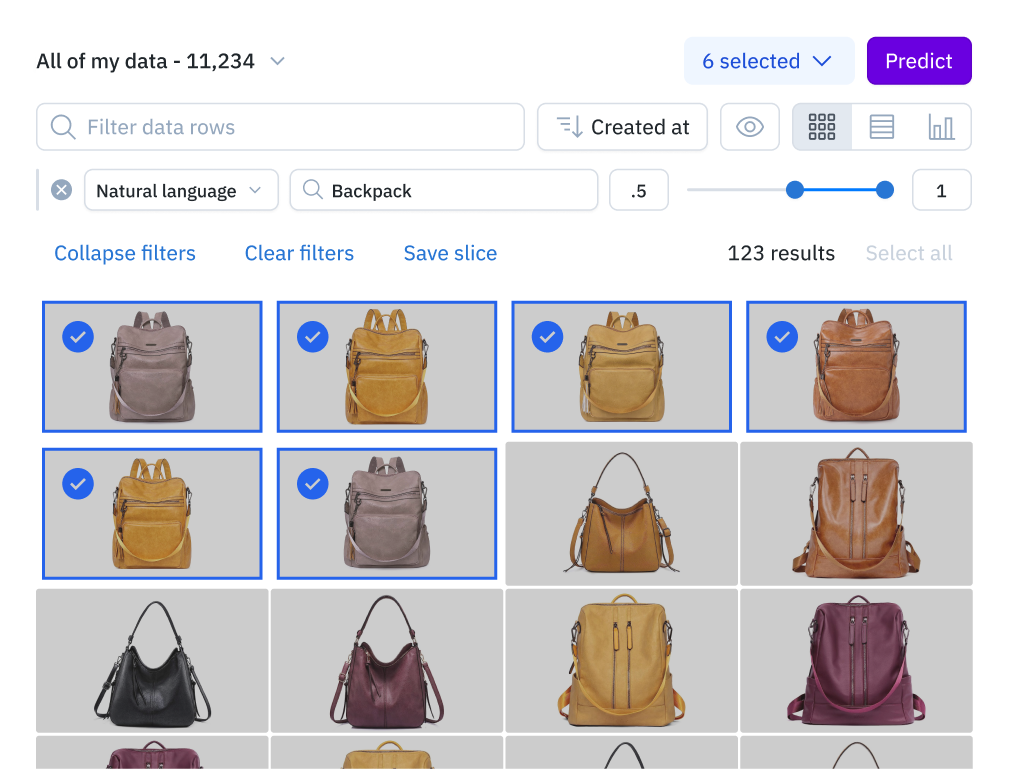
Painlessly consolidate all your product data
Building a recommendation engine requires consolidating data from various sources, which can include product, business, and customer information. This can be challenging to orchestrate and maintain, especially since data is often present in silos in such situations.
Sync all the relevant data for producing recommendations in one place:
Use native integrations with Google BigQuery and Databricks to import and export data between Labelbox and your data storage
Get a holistic view of your data by adding custom metadata and attachments to each asset
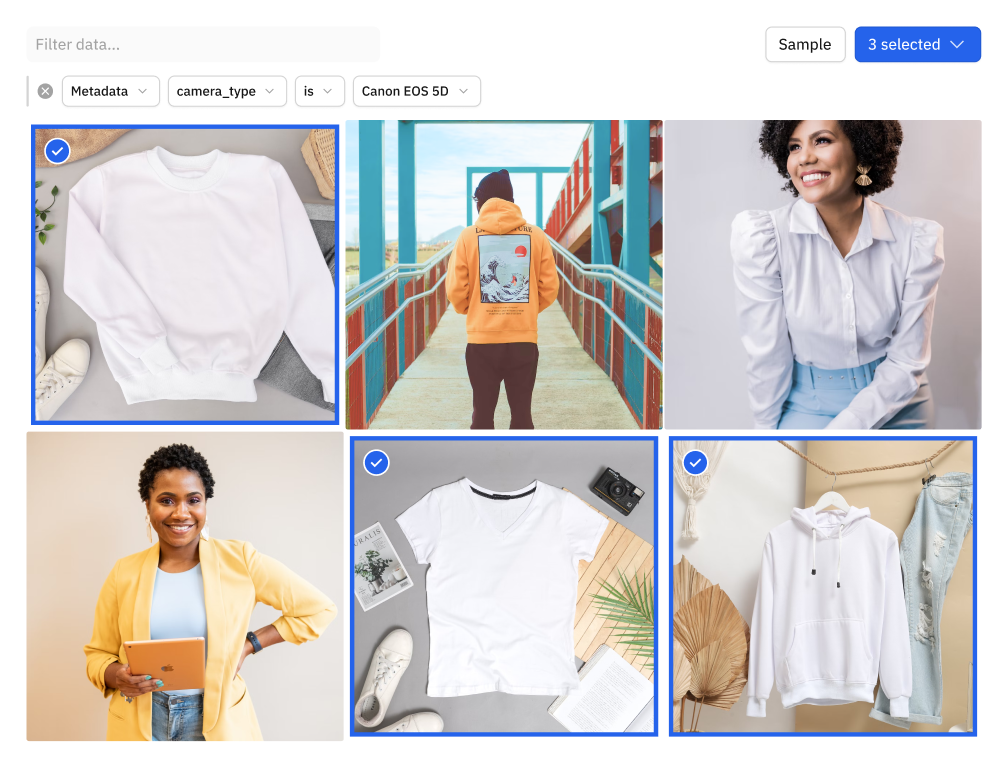
Accelerate product discovery across your entire catalog
Building a powerful product recommendation engine requires a comprehensive understanding of your product data, including product tags, categories, and more. But when you have hundreds or thousands of products, it can be a challenge to search, organize, and manage that data for machine learning.
With Labelbox Catalog, you can easily:
Visualize all of your product listings with key metadata (like product name, model, SKU, color, size, type, etc.) on a single canvas within Catalog
Leverage custom and out-of-the-box smart filters and embeddings to explore product listings, surface similar data, and optimize data curation for ML
Explore your data with natural language queries
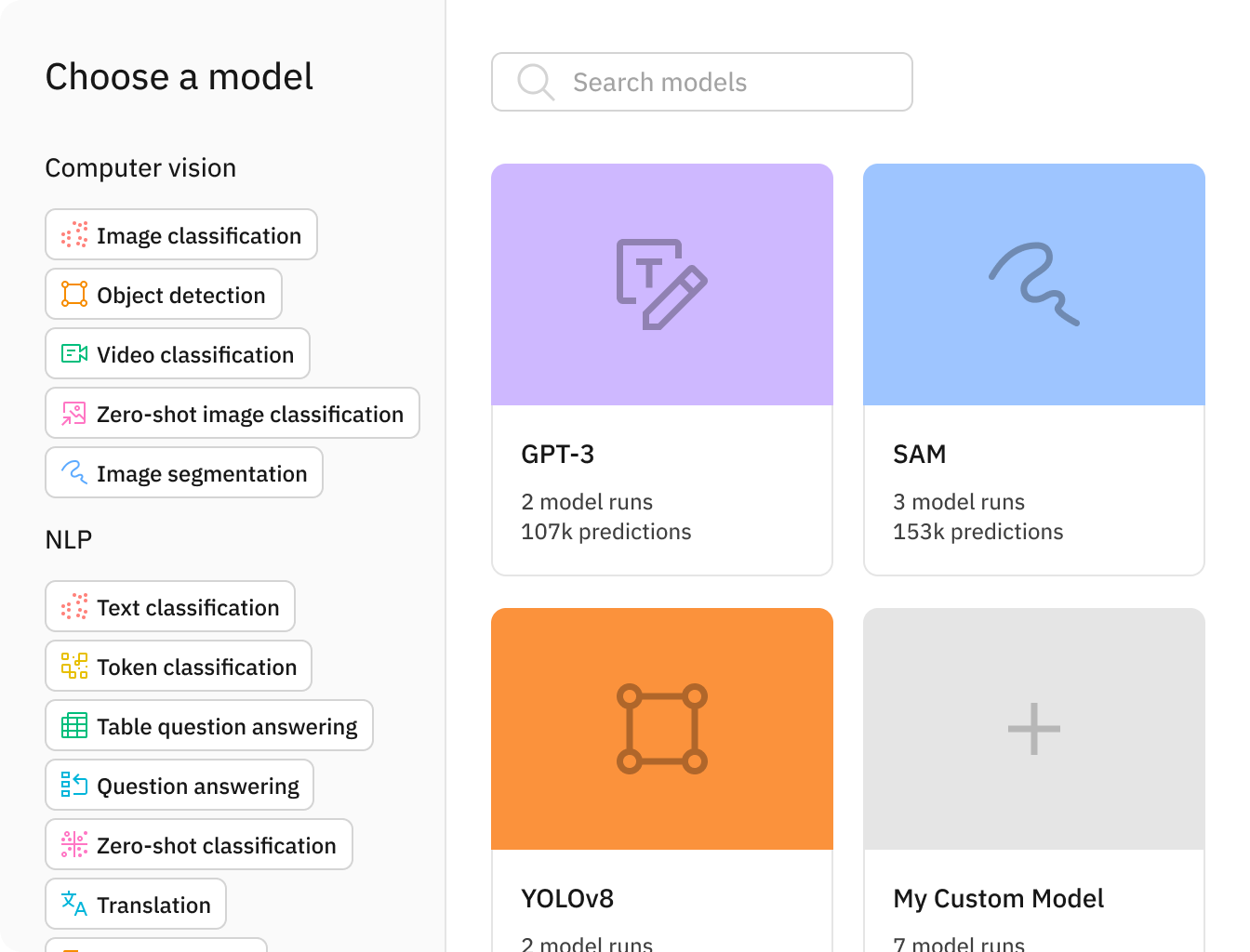
Categorize and curate product listings faster with foundation models
Training product recommendation models requires lots of data with a wide array of features, all accurately labeled. Labeling 500K+ datarows like this with human annotators is often prohibitively expensive and time consuming. By leveraging foundation models with Labelbox's seamless data enrichment and curation capabilities, you can scale easily and create a powerful ML solution, fast.
Enrich data with embeddings and natural language via foundation models and domain expertise to cut labeling time in half
Leverage bulk classification in conjunction with these capabilities to curate data for labeling in a few clicks
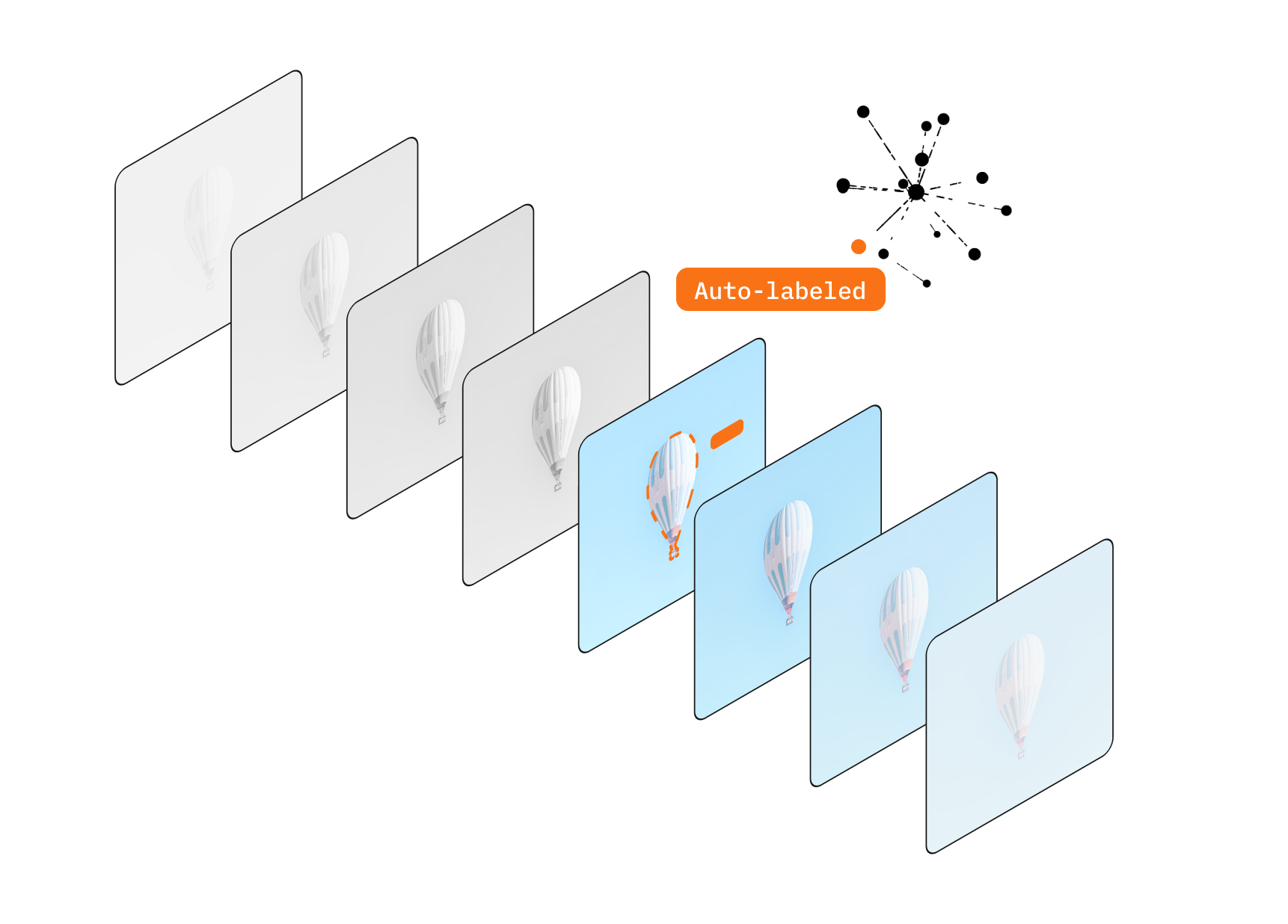
Ensure quality outcomes with automatic metrics and human-in-the-loop verification
While foundation models can automate much of the labeling and data enrichment processes, human review is necessary to ensure that these annotations are correct and appropriate for the use case at hand. With Labelbox, you can see powerful auto-generated performance metrics, and integrate domain experts and human-curated data into your model development process.
Set up custom workflows to review only the labels that need it by using filters for specific annotation types
Quickly conduct model error analysis after every model run and curate your next training dataset based on model performance
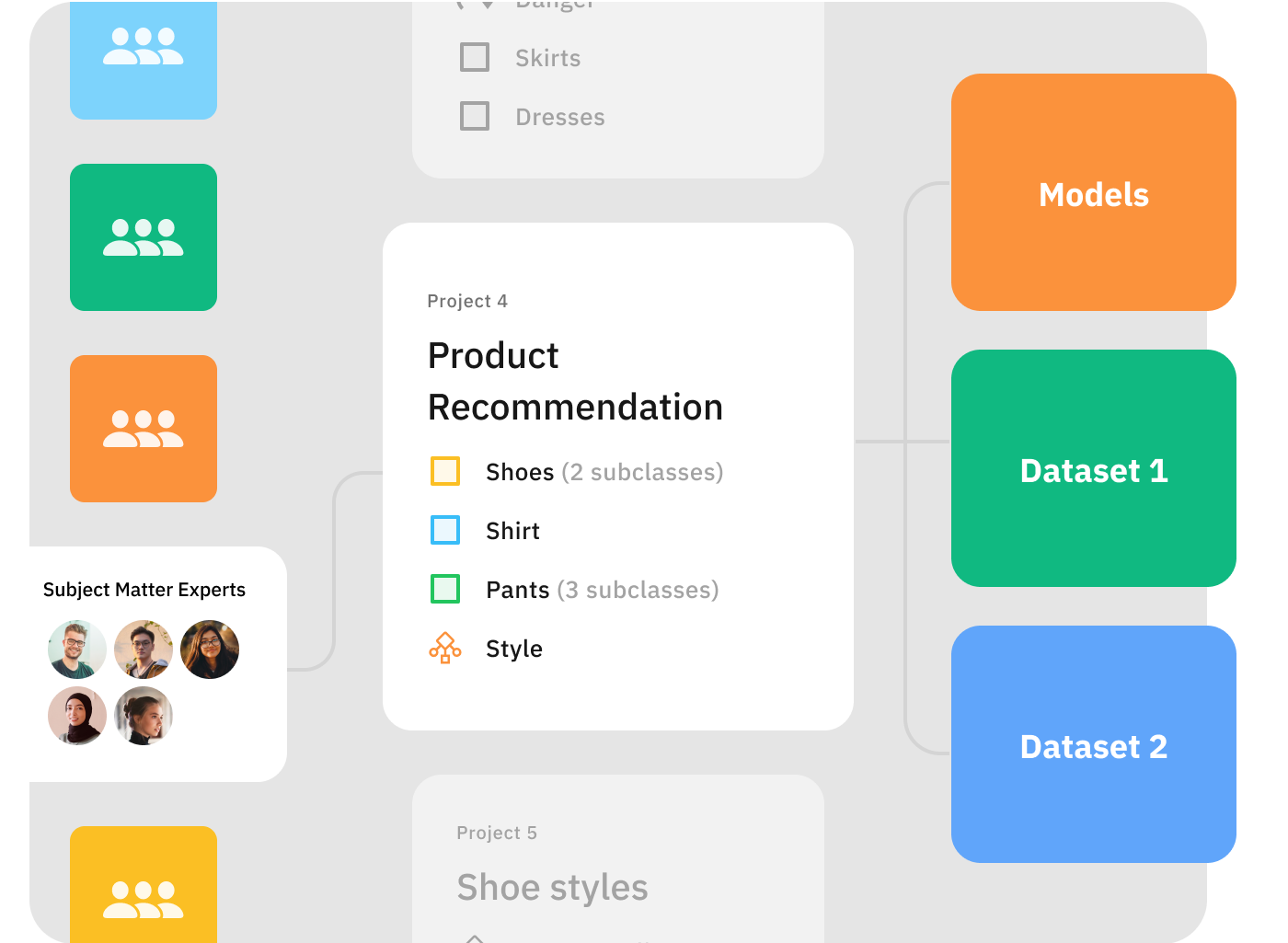
Boost collaboration with enterprise-grade security and access management
Building a recommendations system involves collaboration between data engineers, SMEs, ML engineers, labelers and more. Labelbox supports role-based access controls and workspace isolation so you don’t compromise on security.
Assign roles and associated permissions to ensure that individuals access only what they need
Use workspaces to separate permissions for various teams to maintain security at scale