Labelbox•November 17, 2021
How to optimize your entire ML pipeline
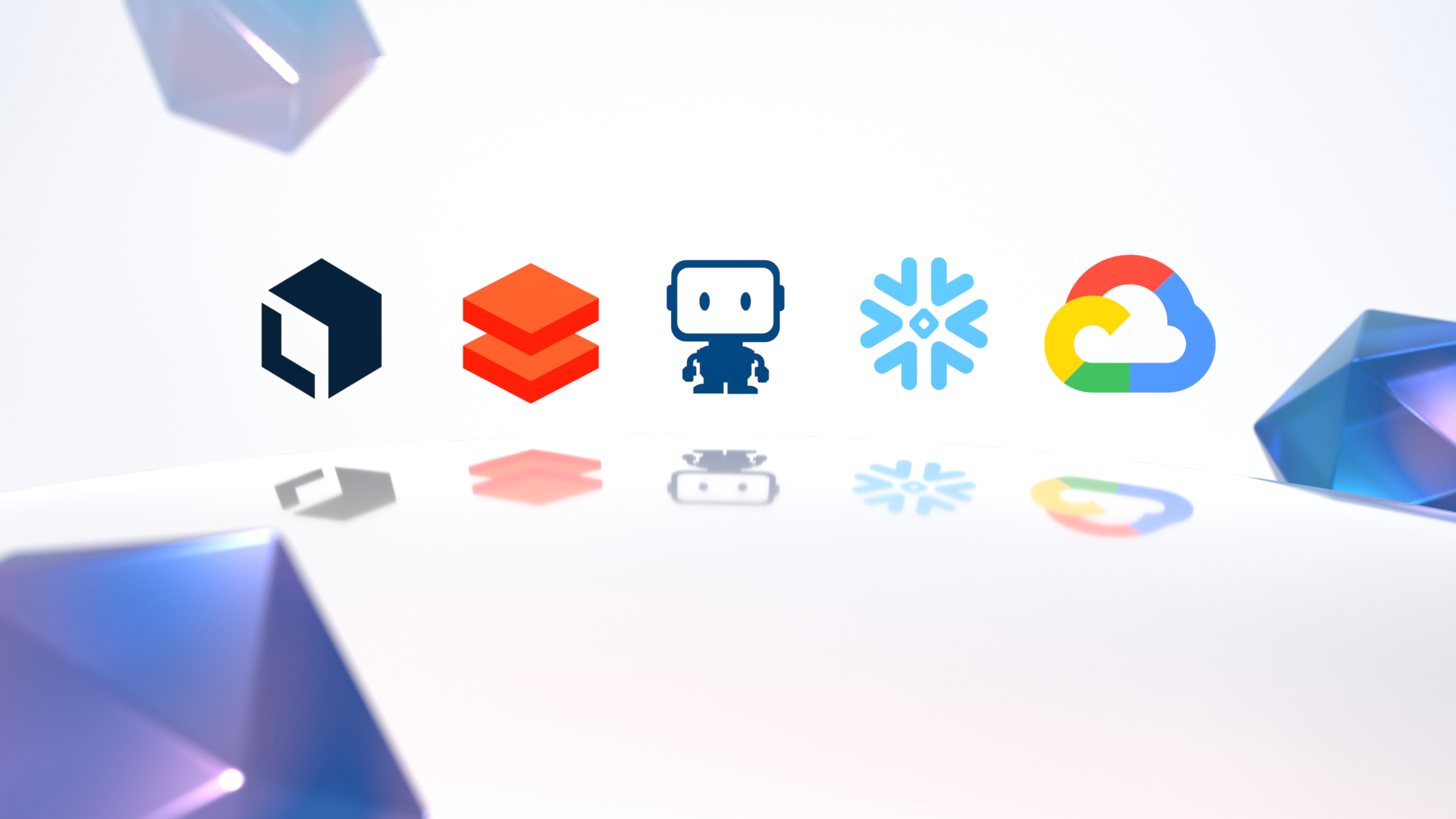
Labelbox’s annotation and automation capabilities have resulted in significant time and cost savings for many of our customers as they generate training data. However, creating quality training data is just one (important) part of the machine learning workflow. ML teams often rely on a variety of providers and platforms for data storage, data management, model development and deployment — all essential components of their process. That’s why Labelbox has partnered with Snowflake, Databricks, DataRobot, and Google Cloud Platform (GCP) to ensure seamless connections as teams store and manage data as well as train and deploy their models.
Add structure to unstructured data
Those working with unstructured data within Databricks often need a way to structure their data, whether it’s for machine learning or business intelligence. ML teams can now use the Labelbox Connector for Databricks to bring unstructured data into our training data platform, annotate it for their use case, and bring it back into Databricks as structured, tabular data. You can learn more about the connector and how to use it by reading our previous blog post on the topic.
Labelbox and Databricks have also been partnering for specific use cases, such as tackling cyberbullying and toxic language in online gaming communities, and two new solution accelerators, which will be available by 2022.
Create an automated, end-to-end data engine
ML teams that embrace automated labeling workflows can get their models to production-ready performance levels much faster than teams who need to hand-label every asset. Creating an automated workflow like model-assisted labeling requires a working ML model — an off-the-shelf one works for some use cases, but more complex projects can require a model designed specifically for labeling purposes.
With Labelbox connectors in DataRobot and Snowflake, this workflow is now easier than ever to set up. Teams can add their unstructured data in Snowflake, use DataRobot to catalog it for governance and transparency, and then develop and deploy the model within the DataRobot platform. This model can then be made accessible to Labelbox, where its output can be used as suggestions or starting points to make complex annotation tasks easier for labeling teams. The data engine available through DataRobot and Labelbox is also the first commercially available deep learning data engine.
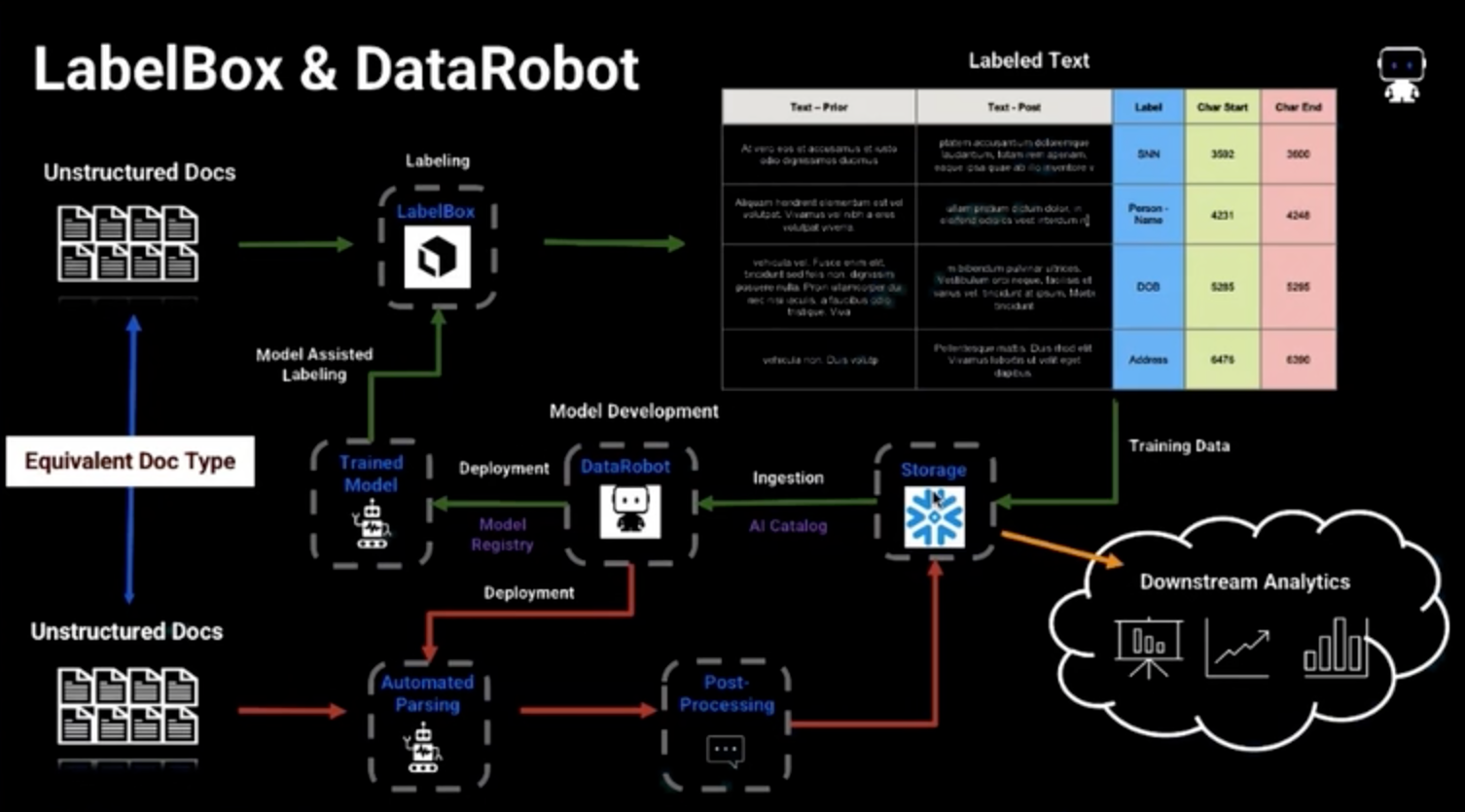
Get Labelbox through the GCP marketplace
Labelbox is now fully integrated with Google Cloud Platform, so you can use the full power of the GCP data cloud. Using GCP with Labelbox makes it easier than ever for anyone to build ML models, from ML engineers and data scientists to non-technical roles such as analysts, marketers, and more. GCP users can now purchase Labelbox from the GCP marketplace.
Watch this upcoming Databricks webinar to learn how you can tackle the challenges of computer vision projects with Databricks and Labelbox. You can also learn more about using Labelbox with Databricks, DataRobot, Snowflake, and GCP by watching the demos from our partner sessions at Accelerate 2021.