Michael Haag•April 9, 2025
Introducing a powerful, new interactive Workflow editor
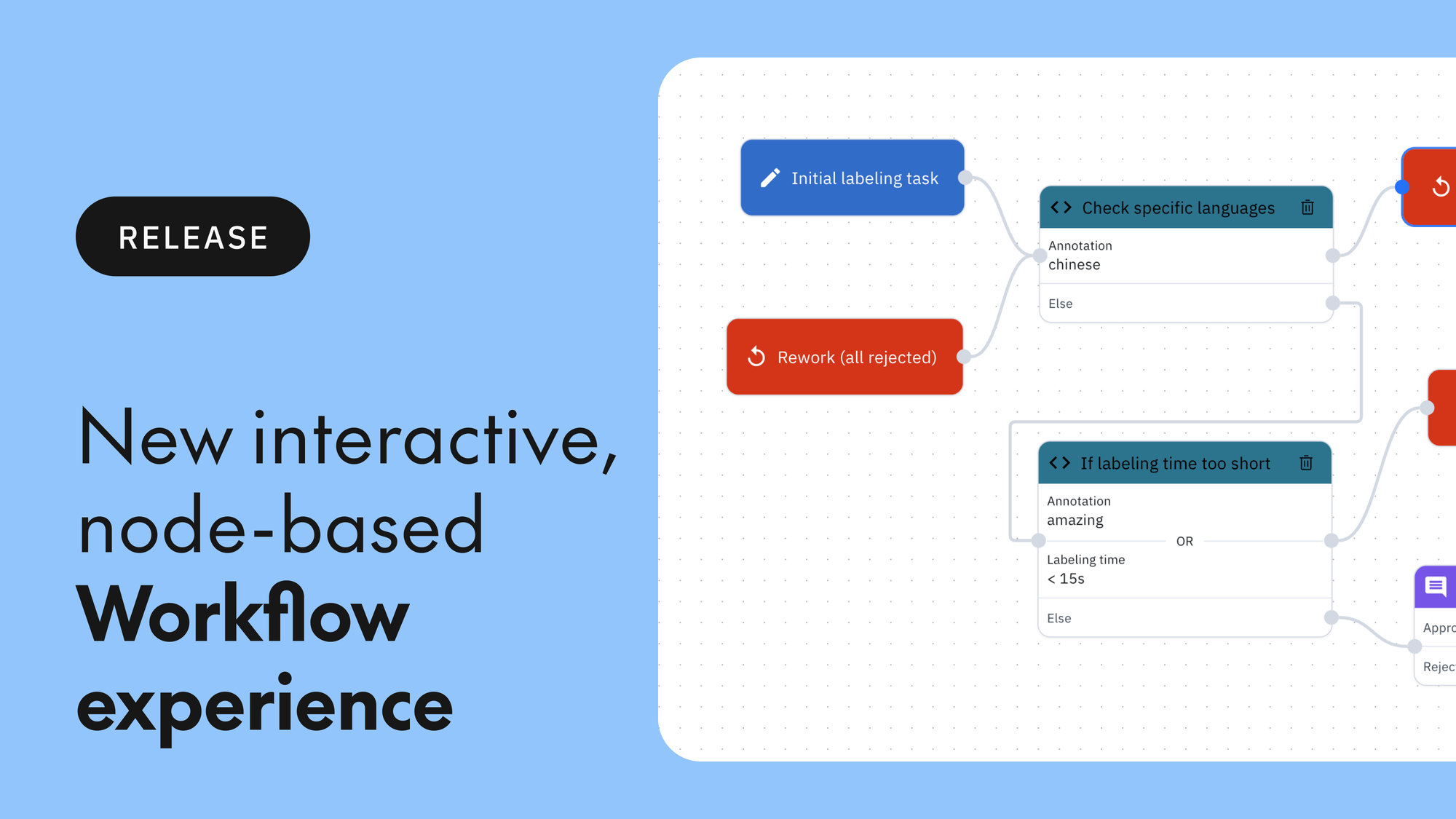
The foundation of powerful AI models lies in high-quality, accurately labeled data and robust quality assurance (QA) processes. Today, we're thrilled to announce a significant enhancement to the Labelbox Platform that gives you unprecedented control and visibility over your data quality pipelines: the new Workflows experience, featuring an intuitive, interactive, node-based editor.
This new Workflow editor empowers AI teams to design, manage, and visualize complex, multi-step review and rework processes with ease while seamlessly incorporating intelligent, AI-assisted quality checks.
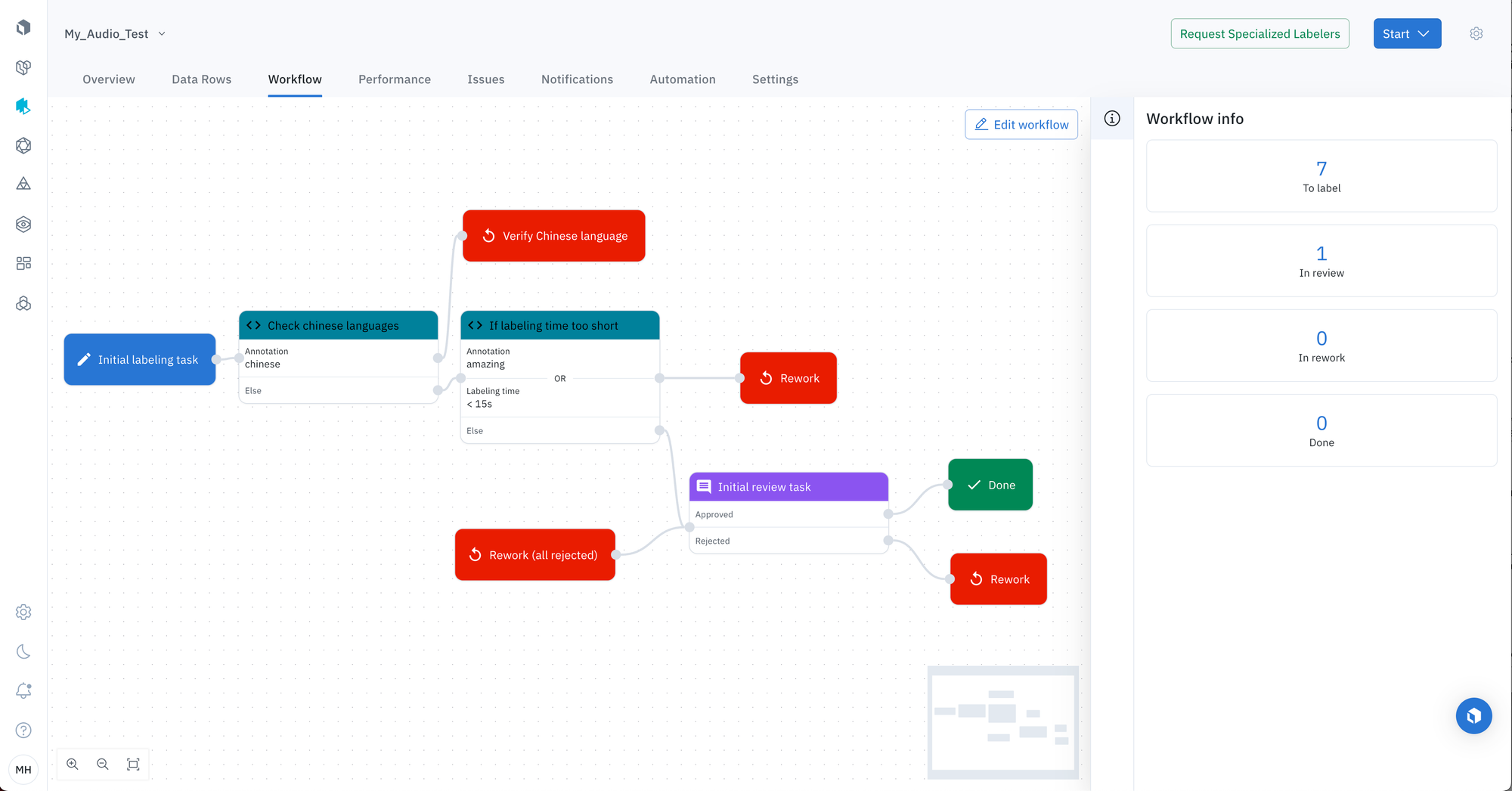
By providing greater customization, improved clarity, and the ability to integrate LLM-as-a-judge, the new Workflow editor helps you ensure data consistency, improve label accuracy, and ultimately accelerate your AI development lifecycle.
If you only read this far, know this: building custom, high-quality data pipelines just got much easier and more powerful in the Labelbox Platform. Read on for more details and what this means to you.
The Critical role of review in high-quality AI data
Developing increasingly complex AI systems demands meticulously curated training data and thorough model evaluation. Achieving this level of quality requires a robust review process. Multiple layers of scrutiny are often necessary to catch errors, resolve ambiguities, and ensure alignment with project guidelines.
This becomes even more critical when leveraging large or diverse teams of labelers or domain experts, like Alignerrs—Labelbox’s global network of highly-skilled AI trainers. These trainers span numerous domains, such as STEM, finance, and multilingual, and vary in background and experience. A well-defined workflow with clear review stages ensures consistency, provides opportunities for feedback and correction (rework), and maintains high standards across the AI trainers.
In addition, with the increasing use of LLM-as-a-judge, modern pipelines should make it easy to incorporate AutoQA steps to streamline and accelerate the review process. Without a strong, adaptable review mechanism, data quality can suffer, leading to underperforming models and costly rework.
Announcing the new Labelbox Workflow editor: Intuitive, powerful, and customizable
Labelbox Workflows have always allowed you to define review steps for your labeling projects. Now, we've completely redesigned the experience to make this process significantly more intuitive, flexible, and powerful.
We've replaced the previous interface with a graphical, node-based editor. Think of it like a flowchart for your data quality process. Each step—labeling, review, rework, and done—is represented by a node. Each node is visually connected to show the path your data takes. This makes it incredibly easy to understand complex logic, identify bottlenecks, and modify your pipeline on the fly.
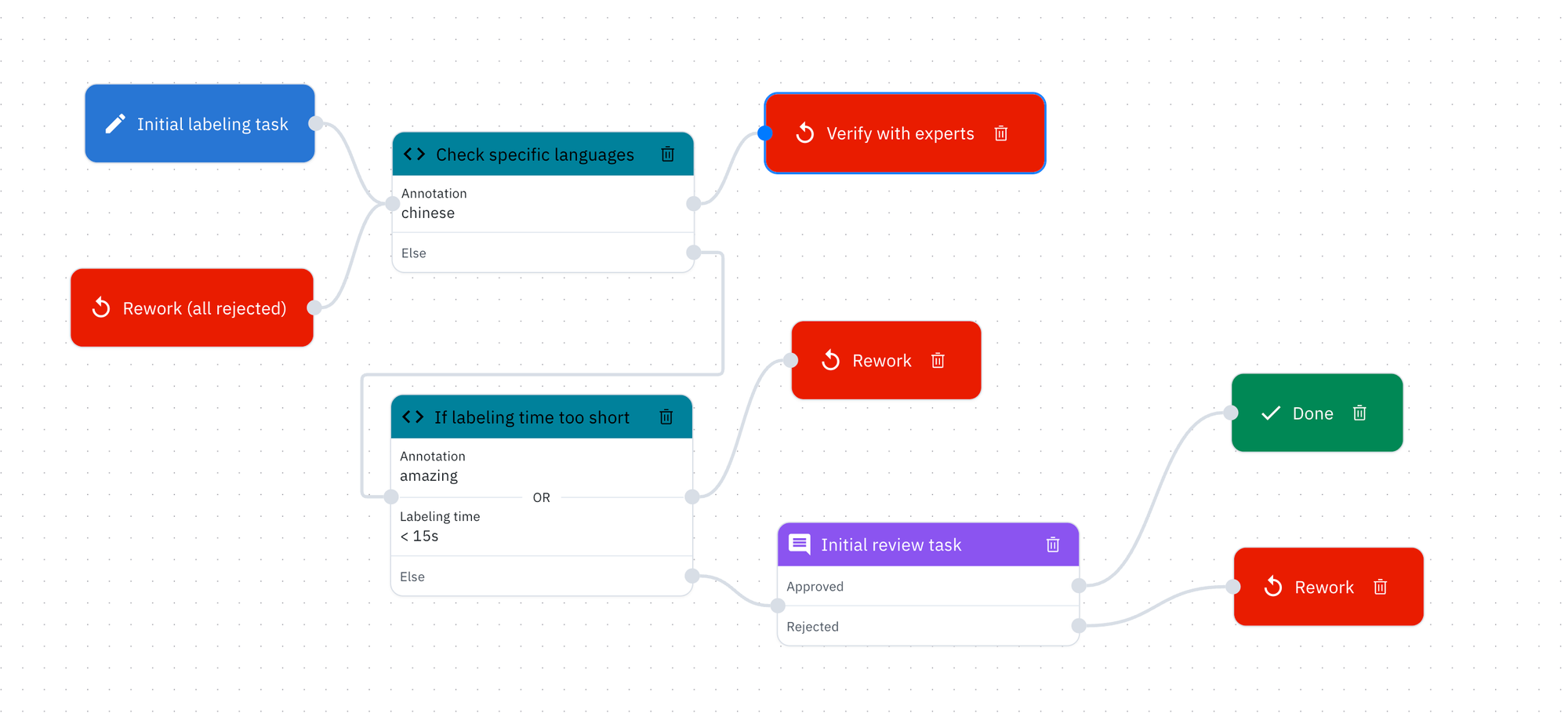
What are Labelbox Workflows and what’s new?
Workflows allow you to create custom, multi-step pipelines for labeling, review, and rework. A workflow consists of configurable Tasks (like "Initial Review" or a custom "Expert QA") associated with predefined Statuses (like "To label", "In review", "In rework", "Done"). This provides a structured yet flexible way to manage data progression. The default workflow includes Initial Labeling, Initial Review, Rework, and Done tasks, but the real power lies in customization.
Our latest version of Workflows, which is now available to everyone, enhances the experience by delivering the following capabilities:
- Interactive, graphical interface: Visualize your entire end-to-end process. Easily add, connect, and configure tasks (nodes) using a drag-and-drop interface. Understand data flow at a glance.
- Easy AutoQA integration: Seamlessly add AutoQA nodes into your workflow. These nodes can leverage LLMs or other models to automatically check labels against predefined criteria, providing feedback, flagging potential issues, and adding a layer of AI-assisted quality control.
- Advanced customization: Go far beyond the default. Create distinct review paths for initial submissions versus reworked data. Implement multi-stage reviews (e.g., peer review followed by expert review).
- Powerful filtering logic: Precisely control which data rows enter specific tasks. Set up filter logic (e.g., based on labeler, consensus score, metadata, or annotation type) using dedicated logic nodes, making complex routing easy to manage and reuse. Try it yourself in this quick click-through demo.
- Group assignments for tasks: Assign specific review or rework tasks to designated Groups within Labelbox. This allows you to direct specialized tasks to the right experts or teams, ensuring efficient use of resources and expertise.
- Built-in validation: The editor includes validation checks to prevent improper configurations, ensuring your workflows are robust and functional.
- Transparent status tracking: Easily see a breakdown of how many data rows are in each status ("To label", "In review", "In rework", "Done") directly within the workflow view.
Technical guidance and usage
Ready to explore the new interface? If you are an Admin or Project Lead, navigate to the Workflow tab within your Labelbox project settings. You'll find the new interactive editor ready to use. Your existing workflow configurations remain unchanged, but you can now visualize and modify them with much greater ease.
The default workflow for new projects includes four tasks: Initial Labeling, Initial Review, Rework, and Done, with the Initial Review task allowing name changes and filtering criteria adjustments. Workflows automatically update as data rows are labeled or rejected, reflecting an iterative review process.
To explore these features in action, users are encouraged to check out the interactive click-through demos in the Labelbox Docs page on the New Workflow Experience.
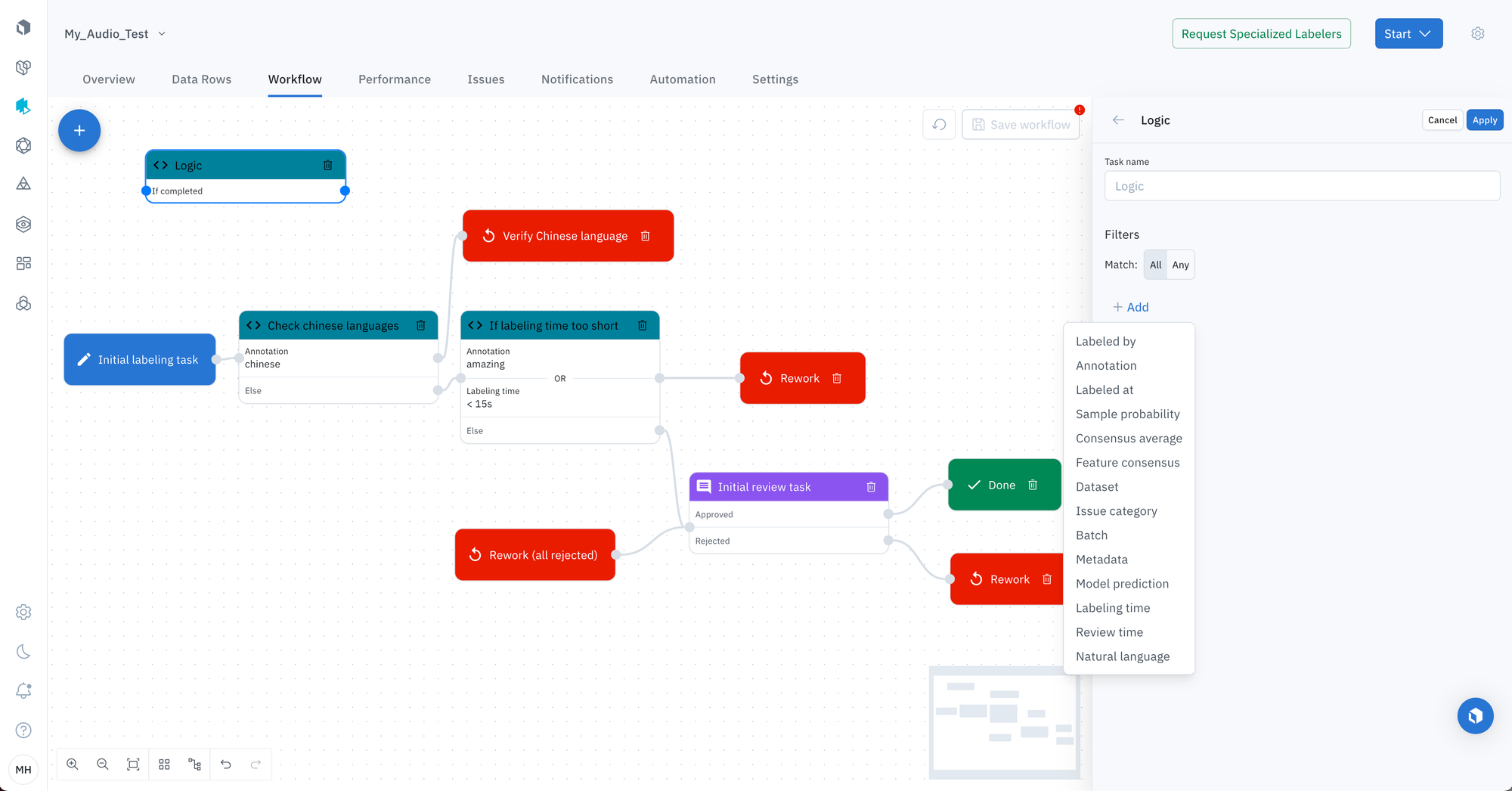
Experiment on your own by adding custom review tasks, assigning groups, implementing filtering logic, and even adding an AutoQA node to see how you can tailor the process to your specific needs.
Closing thoughts and next steps
The new Workflow editor in Labelbox Platform is poised to redefine AI training data management, offering unparalleled flexibility and quality assurance. Whether scaling operations or refining model performance, Labelbox stands ready to support organizations in their AI journey.
We invite you to start exploring Workflows today by signing up for a free trial at Labelbox’s website, or to reach out to the team to discuss tailored solutions for their specific AI needs, ensuring alignment with strategic goals.