Labelbox•February 4, 2020
Announcing $25M Series B led by Andreessen Horowitz to build the Training Data Platform
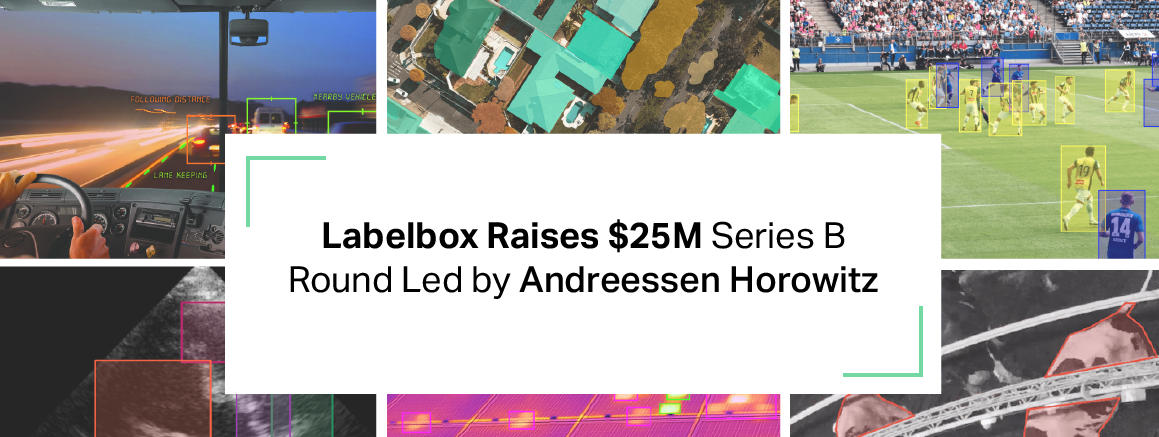
We’re humbled to announce a new round of venture investment led by Peter Levine, general partner at Andreessen Horowitz. Our early investors: Gradient Ventures, Kleiner Perkins, and First Round Capital also participated. Peter is a true visionary who has invested in some of the world’s most iconic companies, including GitHub. We’ve invited Peter to join our board alongside Anna Patterson of Gradient Ventures as we open the next chapter of our journey.
The world is living through a supervised learning revolution. This may not be the ultimate destination of artificial intelligence, but it is the future for economies around the world.
Origin Story
We started Labelbox in early 2018—just under 2 years ago—based on a simple observation: engineers were spending an exorbitant amount of time and effort building data labeling and collaboration platforms from scratch in order to train supervised learning models. There was no off-the-shelf solution. It was like they needed to be experts at car manufacturing in order to drive. So, we got together on nights and weekends and built a simple labeling collaboration tool and posted it on Reddit. When the first company approached us on Reddit asking if they could pay for support, that simple observation turned into a business.
Customers using AI across nearly every industry
Today, Fortune 500 companies and some of the most innovative tech startups—representing nearly every industry you can think of—use Labelbox to create and manage training data to ultimately supervise artificial intelligence. For instance, leading trucking fleet management companies use Labelbox to operate production AI systems that detect driver behavior and send accident alerts in near real-time from over a million dash-cam sensors. Top agriculture companies use Labelbox to train computer vision systems for a new generation of tractors that can selectively kill weeds, reducing herbicide application by over 90%. Some of the biggest healthcare companies use Labelbox to build AI systems to detect tumors and disease in medical imagery—a few of them are already at the cusp of receiving FDA approval. It is exhilarating to see this wide swath of world-changing AI applications being developed with Labelbox. And it’s just getting started.
What we’ve learned
AI is already here, and there are many practical applications for it. Our customers have taught us a few valuable lessons about where the world of AI is going and how Labelbox can serve the community of data scientists, data engineers, and developers building it.
- In its simplest form, machine learning is a “thing-labeler,” according to Cassie Kozyrkov, Chief Decision Scientist at Google. We’ve found that the 3 key ingredients required for machine learning to add business value are (1) a data stream, (2) an opportunity to augment or automate a decision, and (3) an explicit moment when value is created by that decision. This simple framework helps clarify a good AI project from a project designed for AI’s sake.
- Our customers constantly surprise us with novel ways to implement AI. The world is full of potential decisions where AI can help and assist humans. There is no master AI model that can be built to solve for every one of these opportunities with the technology at hand today. As a result, we will need supervised learning to encode human knowledge for the foreseeable future. That knowledge is often quite unique to your business or project.
- The real-world, practical applications of AI are limited not by access to world-class AI models (those are typically free and open source), nor are they limited by compute resources (which is outpacing Moore’s law). The creation and management of high-quality training data is the bottleneck that most AI project teams encounter. The limitation stems from both the tooling needed to train and refine an AI model and the large teams of accurate labelers needed to develop datasets at scale.
The larger movement to Software 2.0
There is a massive paradigm shift in computing underway. Traditionally, software code is written with logic (if, then, else, etc.) with explicit instructions given to the computer by the software developer. The challenge is that in the real-world, it’s hard for a developer to anticipate every scenario possible and to create the logic needed for the application to tackle each scenario. With the advent of machine learning, for the first time in history, we are able to teach a computer about a task through training data, and for the computer to effectively write the software itself by looking for the patterns in the training data. This new paradigm is known as Software 2.0 or data-centric programming.
With data-centric programming, the need for high-quality training data is the fundamental input for production-ready AI software. Our customers, with their imaginative and diverse use cases, need the capabilities to not just treat their training data as a set of images (or other assets) to be labeled, but as their source code and proprietary IP. The requirements our customers ask us to solve are not for merely labeling tools, but to provide the infrastructure to get their unique AI systems into production.
The need for a Training Data Platform
Any team building production AI stumbles upon a glaring omission in the world today: there is no commonly accepted AI software development workflow, nor the infrastructure to create, edit, collaborate on, and manage high-quality training data at scale. We see this as a fundamental barrier to wider AI development and adoption.
Labelbox is a training data platform for the development of AI software. It serves as a standard workflow and single source of truth for an entire organization’s training data. We believe that companies, with their distinctive and innovative use cases should own their workflows and data for developing AI software; it’s the IP and unique advantage of any AI-enabled business. If AI software is to see widespread adoption, we need the robust infrastructure to enable companies to oversee these processes, just like we have for the logically-oriented software development lifecycle.
And labeling data is just one step on the path to production AI software. Iterating on training data is a critical and often over-looked aspect of improving model accuracy. Through editing existing labels, augmenting datasets with new examples, modifying instructions, and collaborating tightly with your labelers, organizations can achieve higher levels of model performance. The ability to create and manage a continuous, iterative improvement process for AI software calls for a training development platform rather than just labeling services.
We can’t do this without great people
If it wasn’t immediately apparent, the market is pulling us forward to fulfill an underserved need at incredible speed. We began 2019 with just nine people and we are on pace to eclipse a hundred teammates this year. We’ve talked about the technology bottlenecks to creating production AI, but the bottleneck for our work at Labelbox is in finding great people who believe in this vision and want to share this journey together.
For anyone who wants to build something great together, we’re hiring! We'd be thrilled if you explored our current career openings or just wanted to chat to explore options. Don't worry about picking exactly the right one as we're happy to give you more options after starting the conversation. Check out the careers page to learn more and please encourage your talented friends to apply.