- Data is the final frontier
- Iterating is the fundamental challenge in AI today
- Model-assisted labeling should be optimized to target costly labels
- Aerial and mobile imagery are transforming how AI is used in insurance
- Higher quality data and labels can help AI teams overcome numerous challenges
- Labelbox features enable a constant stream of data for complex AI projects
- Ease of use is a vital component in a labeling tool
- Building an AI model is easier than it used to be
- An iteration-friendly process is key to a successful labeling operation
- Your business requirements should determine your labeling operations strategy
Labelbox•January 13, 2021
10 insights from the 2020 customer summit
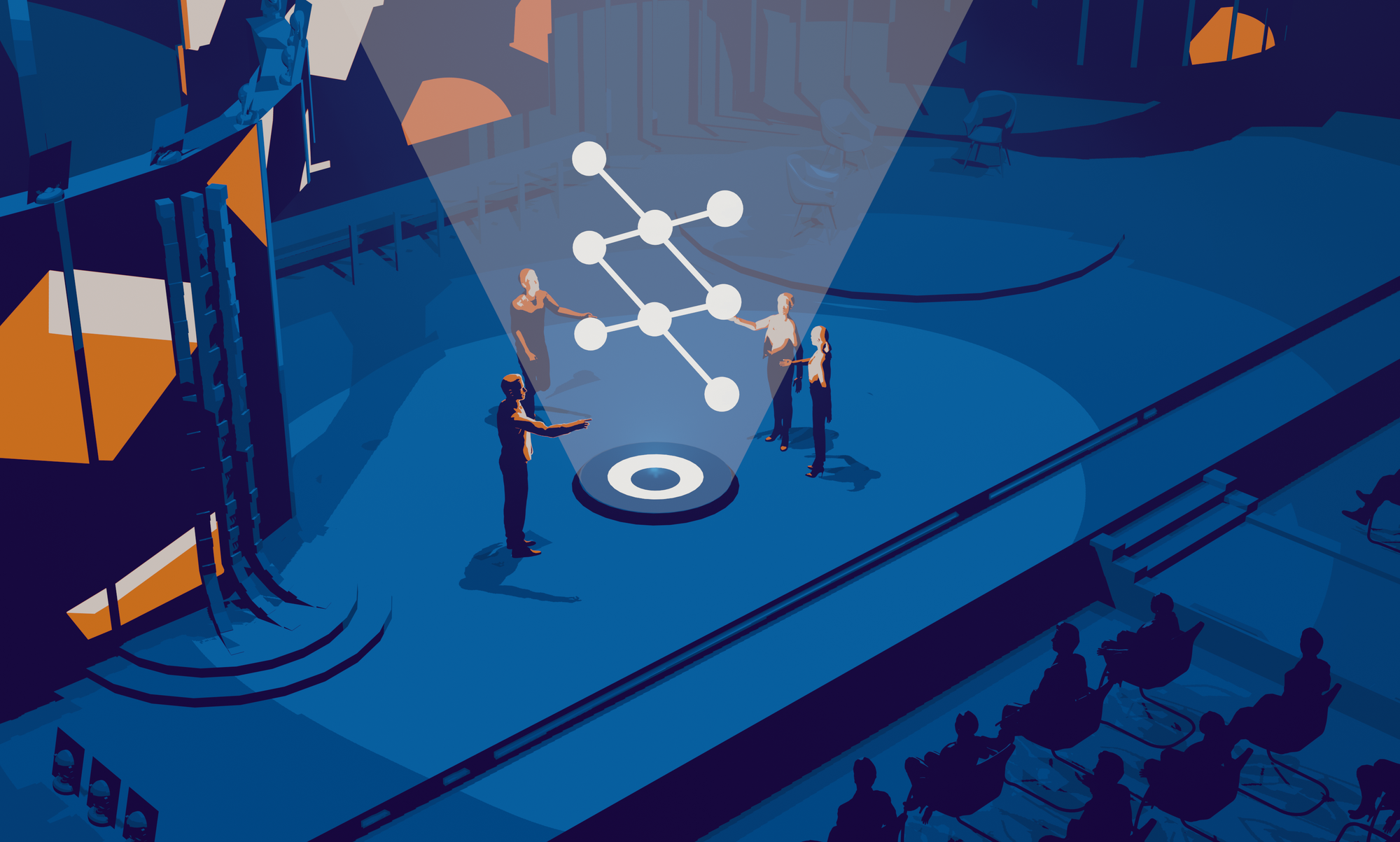
On December 10th, we had the privilege of hosting the first Labelbox customer summit. The virtual event featured discussions with leaders from enterprises like Blue River Technology, Allstate, Orbital Insights, and more. Read on for a recap of ten important moments from the event.
Data is the final frontier
The first session of the summit was a discussion between Manu Sharma, CEO and Cofounder of Labelbox, and Peter Levine of Andreesen Horowitz. The talk kicked off with Manu describing the paradigm shift in computing that’s occurring today: moving from programming with logical statements to teaching computers via training data. “It's complex and messy, and there's a lot of work to do to get it working effectively. The big breakthrough involves the collection and processing of that information,” said Levine.
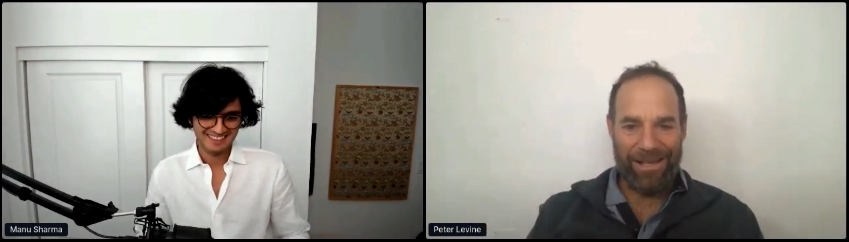
Iterating is the fundamental challenge in AI today
Building AI systems is similar to software programming, but with a few key differences. “The cornerstone for success in software is to have many iterations, quick iterations. AI is no different,” said Sharma. However, in AI, iterations can take weeks longer than in software development. This is the fundamental challenge in the AI industry today. When “we can label more quickly, iterate more quickly, reduce complexity, on massive amounts of unstructured data — that is going to unlock the power of AI,” added Levine.
Model-assisted labeling should be optimized to target costly labels
Emma Bassein of Blue River Technology walked the audience through their labeling journey to train an ML model to differentiate between crops and weeds within field images. To increase labeling speed and accuracy, Bassein’s team conducted a series of experiments, including one with model-assisted labeling. The team discovered that time saved per label varied significantly across images based on the weed pressure and crop size. “It's most important for us to pre-label our large, high weed-pressure images because it saves us, in absolute dollars, the most,” said Bassein. “So optimizing our pre-labeling strategy to target those high cost images was really important.”
Aerial and mobile imagery are transforming how AI is used in insurance
Valerie Fischer of Allstate presented on how AI, enabled by tools like Labelbox, is transforming nearly every aspect of the insurance company. She highlighted two emerging trends for AI use in insurance companies: the use of aerial imagery and data sent through mobile devices. Aerial imagery has allowed insurers to assess damage in areas too dangerous to send their human adjusters, as well as get better information on properties. Data sent from mobile devices, including images taken after vehicle accidents, has helped insurers speed up the claims process. "This improved ability around image detection and identification is helping to bring about a lot of automation...driving down processes that used to take weeks and shortening them to days, and in some cases, even hours,” said Fischer.
Higher quality data and labels can help AI teams overcome numerous challenges
Mohsen Hejrati of Genentech Research and Early Development detailed how his team builds models of diseases — and the challenges their data engineers and annotators must overcome. The data they receive via clinical trials often have gaps, are occasionally inaccurate, heterogeneous due to the patient population and different devices used to collect data, and biased due to the nature of the studies themselves. “The key to success for us was having better data, more data, more high quality labels,” said Hejrati. With Labelbox, the Genentech team was able to generate better quality annotations 5x faster, at a fraction of the cost.
Labelbox features enable a constant stream of data for complex AI projects
David Borsos of Winnow discussed how his team built an ML model that identifies and weighs foods that are thrown away in large kitchens such as restaurants, hotels, and resorts. By providing detailed information on the types and quantities of food that goes to waste to decision-makers in these kitchens, the team at Winnow helps save $40 million of food waste per year — equivalent to 61 tons of carbon dioxide in the Earth’s atmosphere. “Our data is continuously received from around the globe,” said Borsos, “and we have a lot of variety in that data.” The Winnow Vision system retrieves and pushes data constantly, and they leveraged the GraphQL API to upload data continuously without human intervention.
Ease of use is a vital component in a labeling tool
Scott Ehling from Argonne National Laboratory discussed how his team developed training data for an ML model that identifies vehicles that go in and out of their main gate, and uses that information to determine the total pollution in the area. Their labelers were tasked with identifying each vehicle in the dataset and determining the pollutants released by each vehicle. However, their labeling team was pulled from the Argonne workforce, and were not experienced data labelers. “This was our first foray into labeling and annotation, and the ease of use and real-time analytics were a real benefit,” said Ehling of the Labelbox platform.
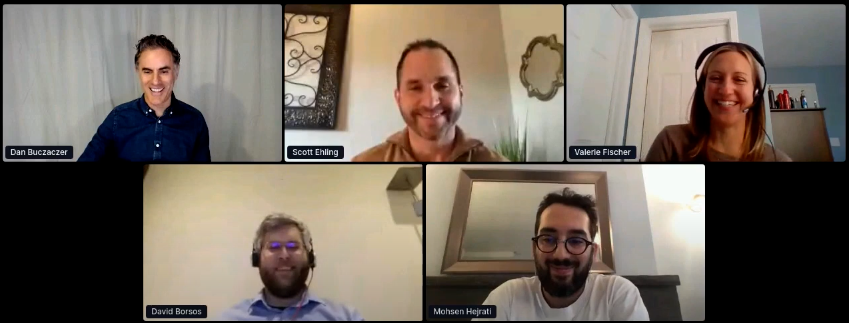
Building an AI model is easier than it used to be
Building an AI model today is more straightforward than ever before. "Just eight years ago, the entire pursuit for the industry was to come up with better, faster algorithms. In fact, most of the organizations had data scientists...whose primary job was to come up with a novel architecture for a neural network,” said Manu Sharma in his presentation, 3 Key Layers to a Training Data Platform. But now, algorithms are freely available off the shelf.
An iteration-friendly process is key to a successful labeling operation
Labelbox COO Brian Rieger joined Annie Neligeorge of Orbital Insight and Brian Keller of Flyreel for a discussion on how to build a successful labeling operation. "Process is key. Most labeling operations involve a lot of different projects going on at once...I want to make sure that all my analysts, my GIS specialists, are performing their labeling operations exactly the same, so that we're repeatable and scalable,” said Neligeorge.
Your business requirements should determine your labeling operations strategy
When asked about critical things to get right when setting up a labeling operation, Brian Keller of Flyreel replied: "First understand the business problem. Everything flows from that...it indicates the type of model that you might want to use, the amount of accuracy that's required for the application...the amount of data that's required."