How a leading genAI text-to-image app accelerates product development with high-quality labeled data
Problem
To improve their text-to-image AI models, the company was looking for ways to rapidly generate high-quality human preference training data. However, creating and labeling this data internally would have diverted valuable resources and focus away from their core product development efforts. Generative AI data labeling required dedicated tooling and labeling teams to meet narrow timeframes given the company's pace of product development.
Solution
Labelbox’s efficient and reliable data labeling platform and Boost Labeling Services was used to improve their text-to-image data quality, while delivering highly-skilled labelers along with project management experts that allowed them to meet their deadlines. Using Labelbox’s LLM human preference editor, the company was now able to perform reinforcement learning from human feedback (RLHF), which enables them to easily create preference data for training a reward model based on multiple outputs from a single model.
Result
Improved speeds at which they could create high-quality human preference training data by 2x and speed up product development from months to weeks by using Boost Labeling Services.

A leading app developer of generative AI tools used to create realistic media (in the form of images, posters, logos, etc) was searching for a way to generate high-quality human preference training data for their text-to-image generative AI development. The company wanted to focus on their core product development efforts and did not want to label high-volumes of data themselves, so they selected Labelbox’s advanced tooling for LLM human preferences combined with Labelbox Labeling Services as an end-to-end solution for data annotation and comparing & classifying model outputs as an easy-to-use service.
As a developer of generative media tools, the company’s goal is to make creative expression more accessible and fun. The AI text-to-image company’s inception came from developing a tool similar to OpenAI’s DALL-E 3 and Midjourney, which takes a user’s text prompt and automatically generates and modifies images to the preference of the user, all behind the scenes and invisible to the user.
The company's platform offers state-of-the-art functionalities, enabling individuals to explore and experiment with creative endeavors through the use of generative AI. The company has also been prioritizing features that help users better communicate with the underlying AI model, effectively translating user input into a more machine-friendly format without requiring the user to engage in as much trial and error.
To improve their text-to-image AI models, the company was looking for rapid ways to generate high-quality human preference training data. However, generating and labeling this data internally would divert valuable resources and focus away from their core product development efforts. The complexity and labor-intensive nature of data labeling posed a significant hurdle, as it required dedicated tooling and labeling teams to meet narrow timeframes given the pace of product development.
To address these challenges, the company used Labelbox’s efficient and reliable data labeling platform and Labelbox Labeling Services to improve their text-to-image data quality, while delivering highly-skilled labelers along with project management experts that allowed them to meet their deadlines.
For advanced tooling, the company used Labelbox’s LLM human preference editor to perform reinforcement learning from human feedback (RLHF), which enabled them to easily create preference data for training a reward model based on multiple outputs from a single model.
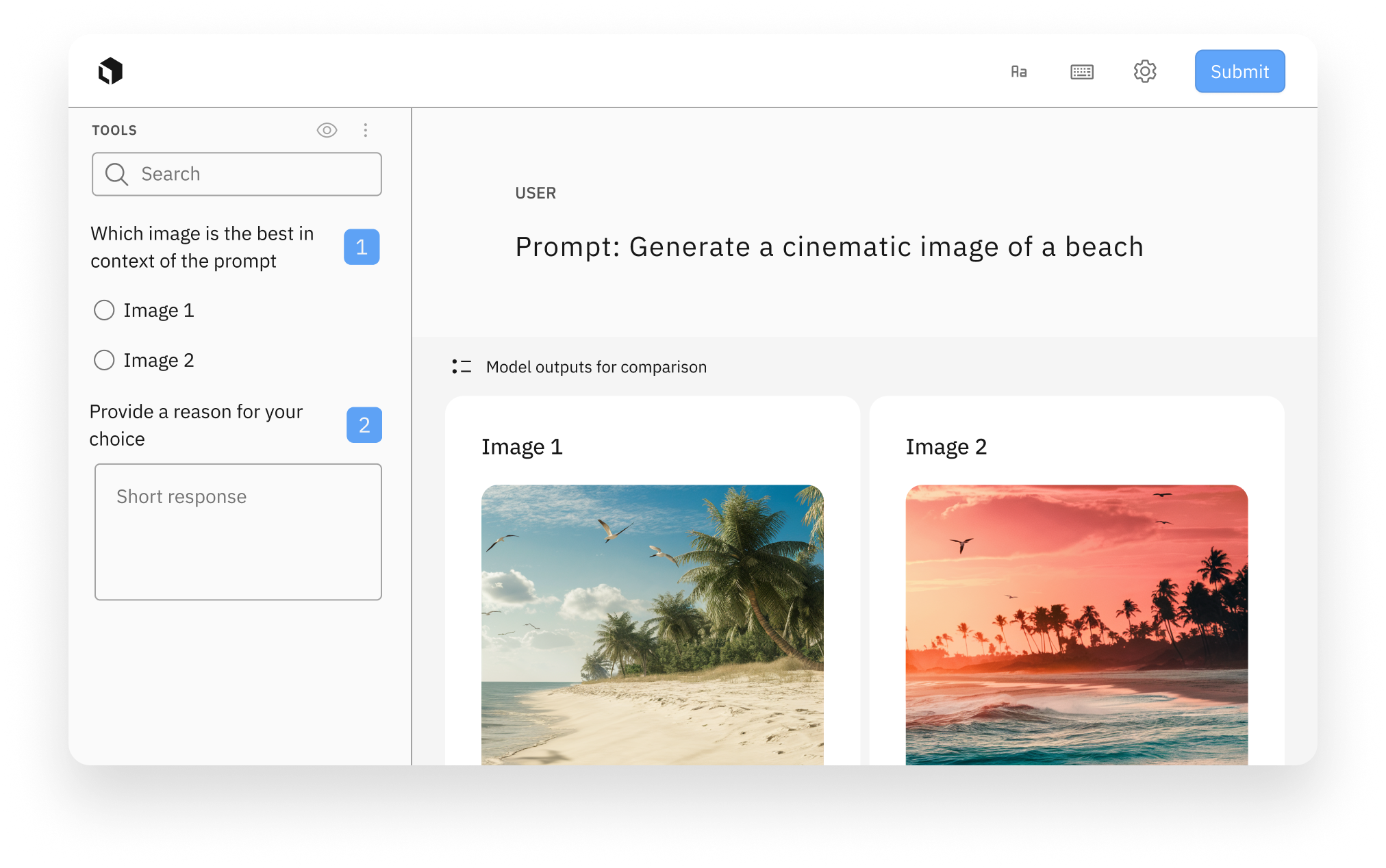
In addition, the company was able to leverage Labelbox's latest multimodal chat solution to improve throughput for creating additional data that would fix where their models were underperforming. This was done by comparing model outputs on text-to-image prompts versus other leading models.
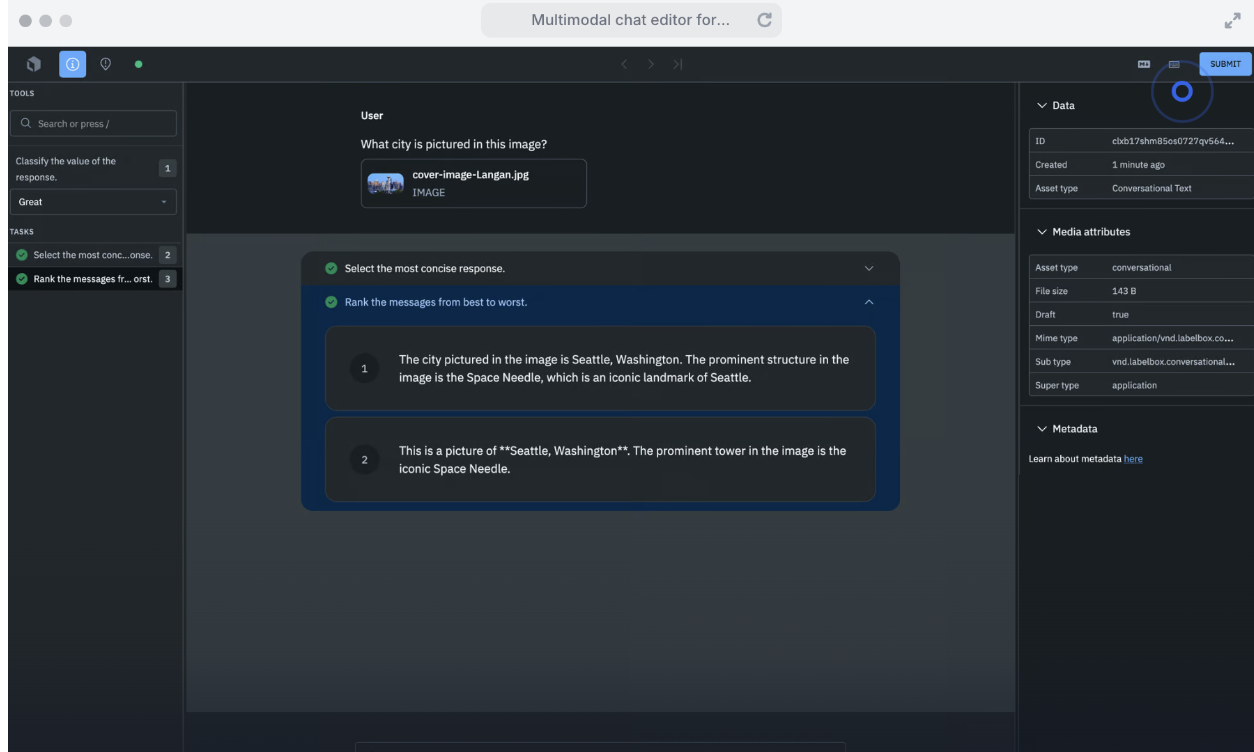
With this solution, the company was now able to implement a data engine workflow which allowed them to compare multiple models against each other in live, multi-turn conversations and rank outputs for a more data-driven evaluation of models. This human evaluation approach would then help them figure out how their models performed on a weekly basis against competitors, as well as their own models in development. Afterwards, the company would target training data creation towards the areas which needed the most improvement and which would have the most impact on model performance, and iteratively fed back and verified their results through another human evaluation run.
By using Labelbox, the company was now able to improve the speed at which they could create high-quality human preference training data by over 50% and sped up product development from months to weeks given Labelbox Labeling Services’ ability to deliver the labeling efforts needed to support their text-to-image generative AI development versus using their own internal teams. As a next step, the company is now evaluating highly-skilled labeling workforces with image expertise to improve their core products and to meet the significant pace of market interest and growing consumer adoption.