AI assisted alignment
AI Assisted Alignment is an approach developed by Labelbox to produce training data by leveraging the power of artificial intelligence to enhance every aspect of the process. From data curation to model-based pre-labeling and catching mistakes or providing feedback, AI assists humans to achieve significant leaps in levels of quality and efficiency.
Auto label data with AI
Auto label data with leading foundation or fine-tuned models. Achieve breakthroughs in data generation speed and costs. Re-focus human efforts on quality assurance.
View all modelsAI critic in the loop
As frontier AI models continue achieving greater capabilities, aligning them requires more scalable methods that help expert humans to make better judgment. Use specialized LLMs to provide feedback or score labels, automatically approve or reject labels for further review.
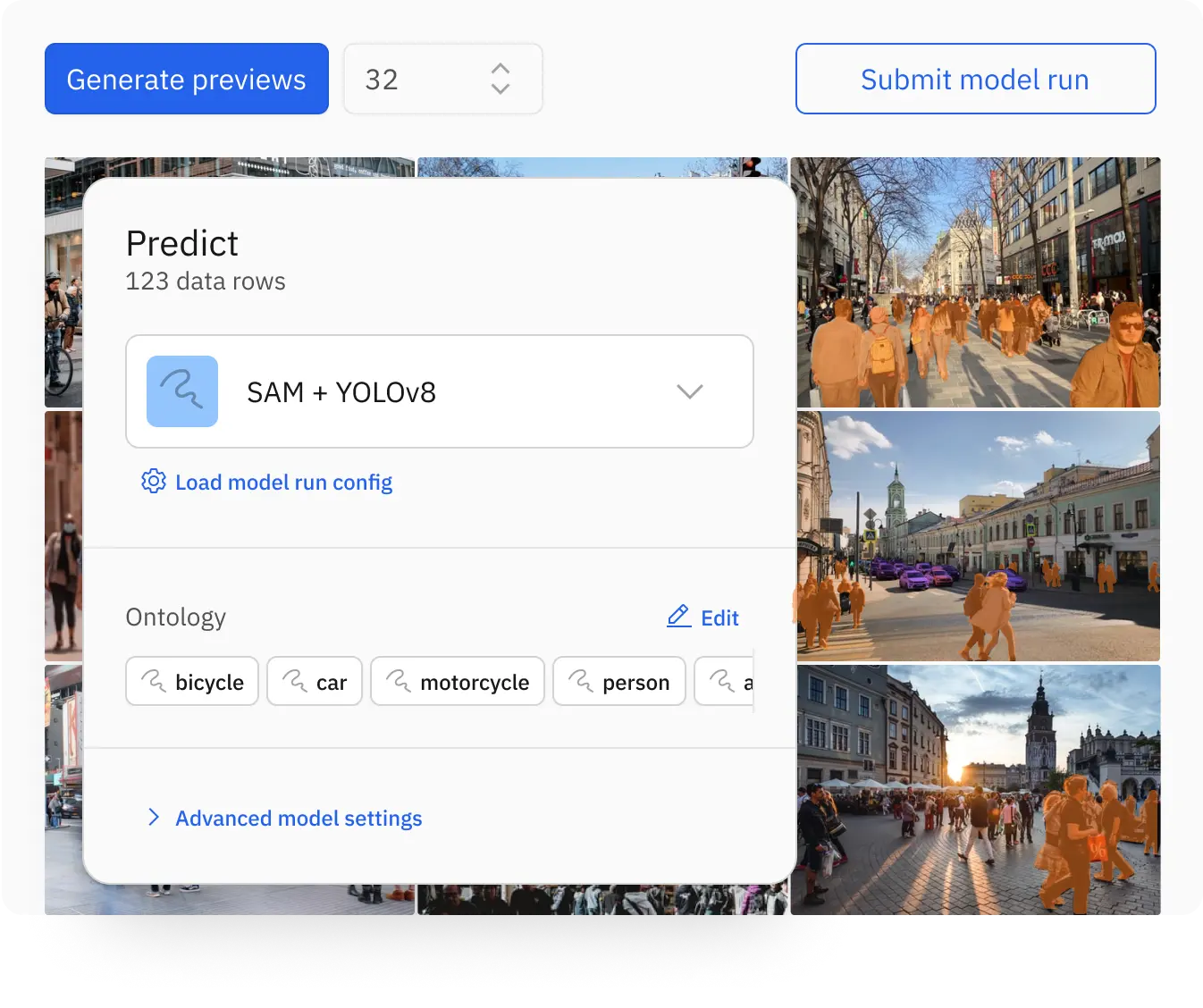
Pre label data in a few clicks
AI builders can now enrich datasets and pre-label data in minutes without code using foundation models offered by leading providers or open source alternatives. Model-assisted labeling using Foundry accelerates data labeling tasks on images, text, and documents at a fraction of the typical cost and speed.
Data curation with natural language
Prioritize right data to label by leveraging out-of-the-box search for images, text, videos, chat conversations, and documents across metadata, vector embeddings, and annotations.
Live in-editor assistance
Labelbox brings AI assistance to real-time to assist data labelers in annotating images or video. Discover how Labelbox uses Segment Anything model by Meta to accelerate image segmentation