Labelbox•April 20, 2020
Using model-assisted labeling to speed up annotation efficiency with Labelbox
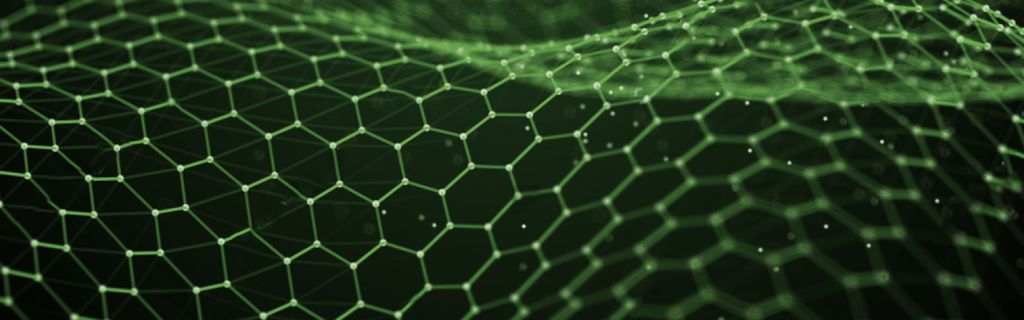
Labelbox is committed to partnering with researchers at universities globally to inspire cutting-edge research and to find new ways to help humans advance AI. One of the ways that we've accomplished this is by offering our software license for free for non-commercial research. Our goal is to empower the academic community to perform their work more efficiently and to collaborate with other experts from around the world using Labelbox's training data platform.
Recently featured in Nature's scientific journal, a team of researchers at the Institute of Industrial Science, a part of the University of Tokyo, leveraged our model-assisted labeling features in order to speed up their machine learning processes. The purpose of their research was to develop an autonomous robotic system to search for two-dimensional (2D) materials. Two-dimensional materials offer an exciting new platform for the creation of electronic devices, such as transistors and light-emitting diodes but historically, finding them under a microscope was a tedious job, which made it a strong candidate for automation with machine learning.
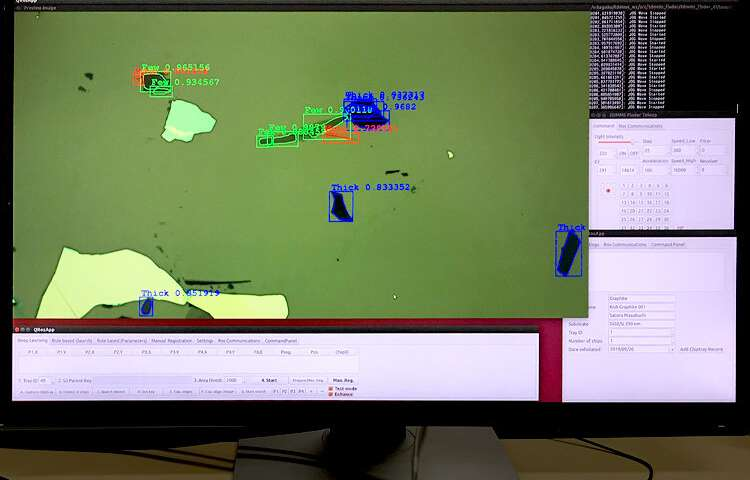
To prepare their training dataset, Satoru and his team obtained Mask-RCNN models to segment 2D crystals and employed a semi-automatic annotation workflow using Labelbox. We wanted to highlight their training data process in the event that it may help other researchers speed up their efforts.
- The team first trained the Mask-RCNN with a small dataset consisting of ~80 images of graphene.
- Afterwards, predictions were conducted on optical microscope images of the graphene.
- The prediction labels generated using the Mask-RCNN were all stored in Labelbox via API.
- Labelbox's model-assisted labeling workflow was leveraged to allow their team to import computer-generated predictions and load them as editable features on an asset.
- Once loaded, these labels were manually corrected by a human annotator.
- The result? This procedure greatly enhanced their annotation efficiency, allowing each image to be labeled in just 20–30 seconds as opposed to minutes.
We look forward to showcasing more cutting-edge use cases of Labelbox in the coming months. If you're interested in using model-assisted labeling for your own project, you can learn more here.
To apply for a Labelbox academic license, please visit here.
Lastly, a big thanks to Satoru and his team for their insightful research. You can check out the full paper below.
"Deep-learning-based image segmentation integrated with optical microscopy for automatically searching for two-dimensional materials" (link)
Satoru Masubuchi, Eisuke Watanabe, Yuta Seo, Shota Okazaki, et al. (2020)