Labelbox•January 26, 2021
4 Things we learned from the state of training data
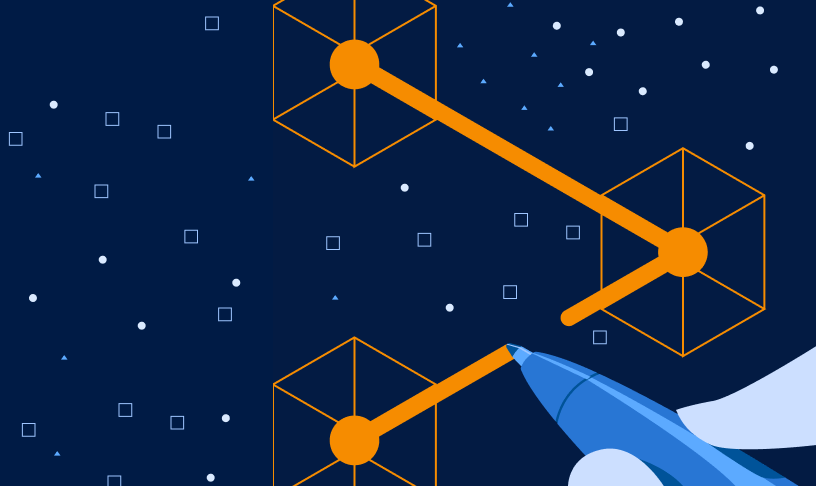
At the Labelbox Customer Summit in December, we held a short poll session to gauge how the attendees were handling the training data creation process at their companies. We asked attendees about how how long they've been training their models, how many they have in production, how many external teams they work with, and more. Below are four compelling takeaways from the poll.
More than a third of responders are productizing AI
Surprisingly, 36% responded that they were in the productizing phase of building AI models. The rest of the respondents were about evenly split between the assembling, testing, and building phases. Companies are now increasingly successful in their AI efforts, and they’re getting closer to realizing the competitive benefits of AI.
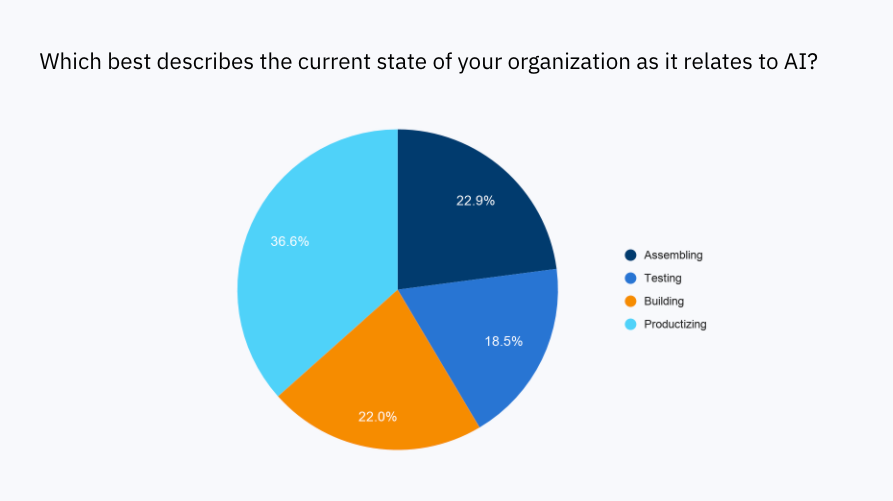
Most companies are labeling images — but the text and video categories are set to grow over the next 1-2 years
80% of companies polled said they are currently labeling images, with text and video splitting the remaining 20%. However, when asked what they’re looking to label in the near future, the text and video categories nearly doubled in size. Labeling video presents a few more challenges compared to images, but also more possibilities, like training your AI model to understand the concept of time.
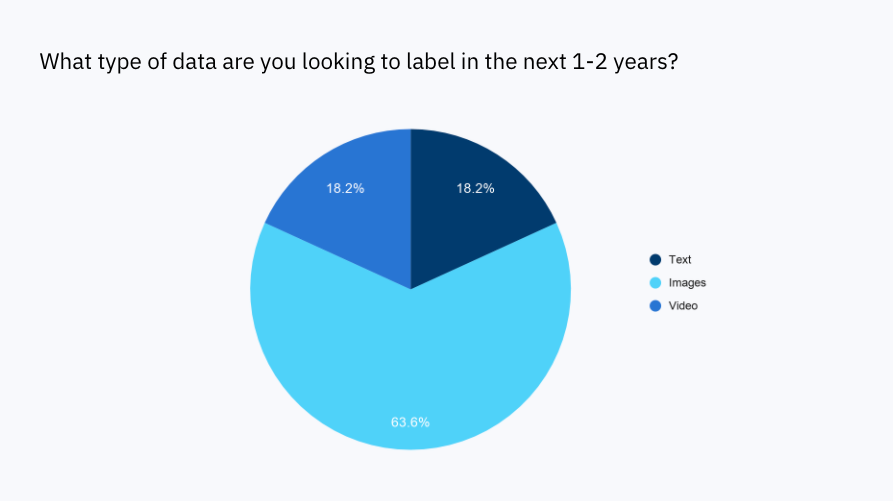
50% of respondents use automation in their labeling process
Automating parts of the labeling process is becoming more common in enterprise AI teams, typically used to speed up some of the more tedious tasks to save both time and costs. As more teams embrace automation in labeling, particularly methods like weak supervision and model-assisted labeling, we can expect to see ML models become production ready faster than ever before.
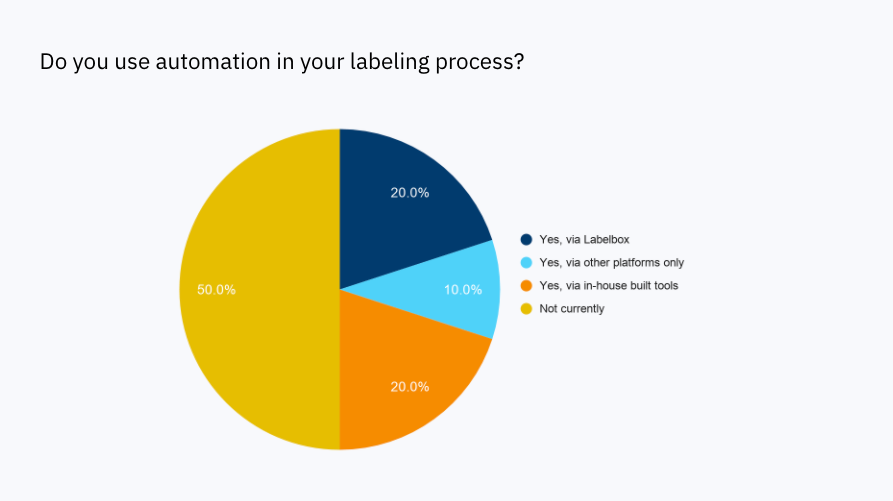
41% of respondents say their proprietary data sources are the biggest differentiator in how they use AI
There’s no doubt that data is an increasingly valuable asset for most organizations, and this holds true for how they build and use AI as well. It’s no longer enough to train an algorithm on off-the-shelf, public data; the real benefits to AI are revealed when organizations use their own data.
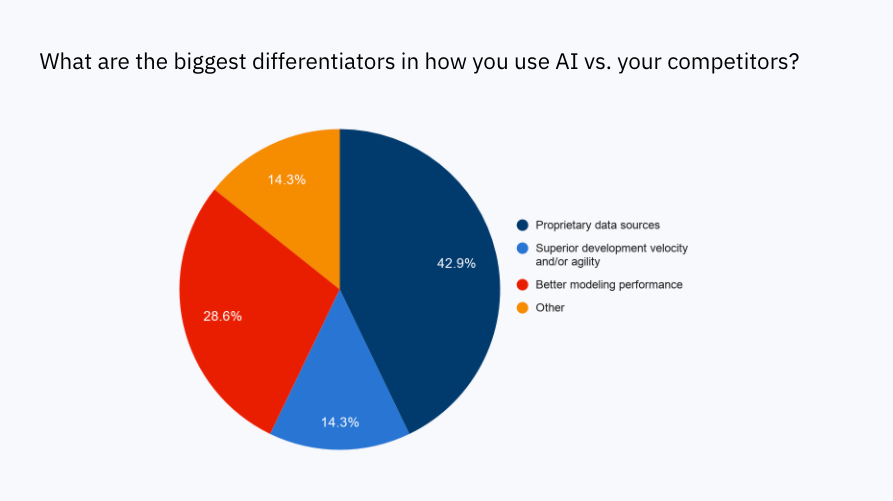
It’s clear that organizations are building more sophisticated ML models and innovating on ways to make the labeling process work better. The right tools — such as a training data platform — can make the process much more efficient as your organization moves toward production ML.
Take the poll below to learn how your ML efforts compare.