Labelbox•June 16, 2022
How Deque uses data prioritization and model diagnostics to unlock AI breakthroughs in digital accessibility
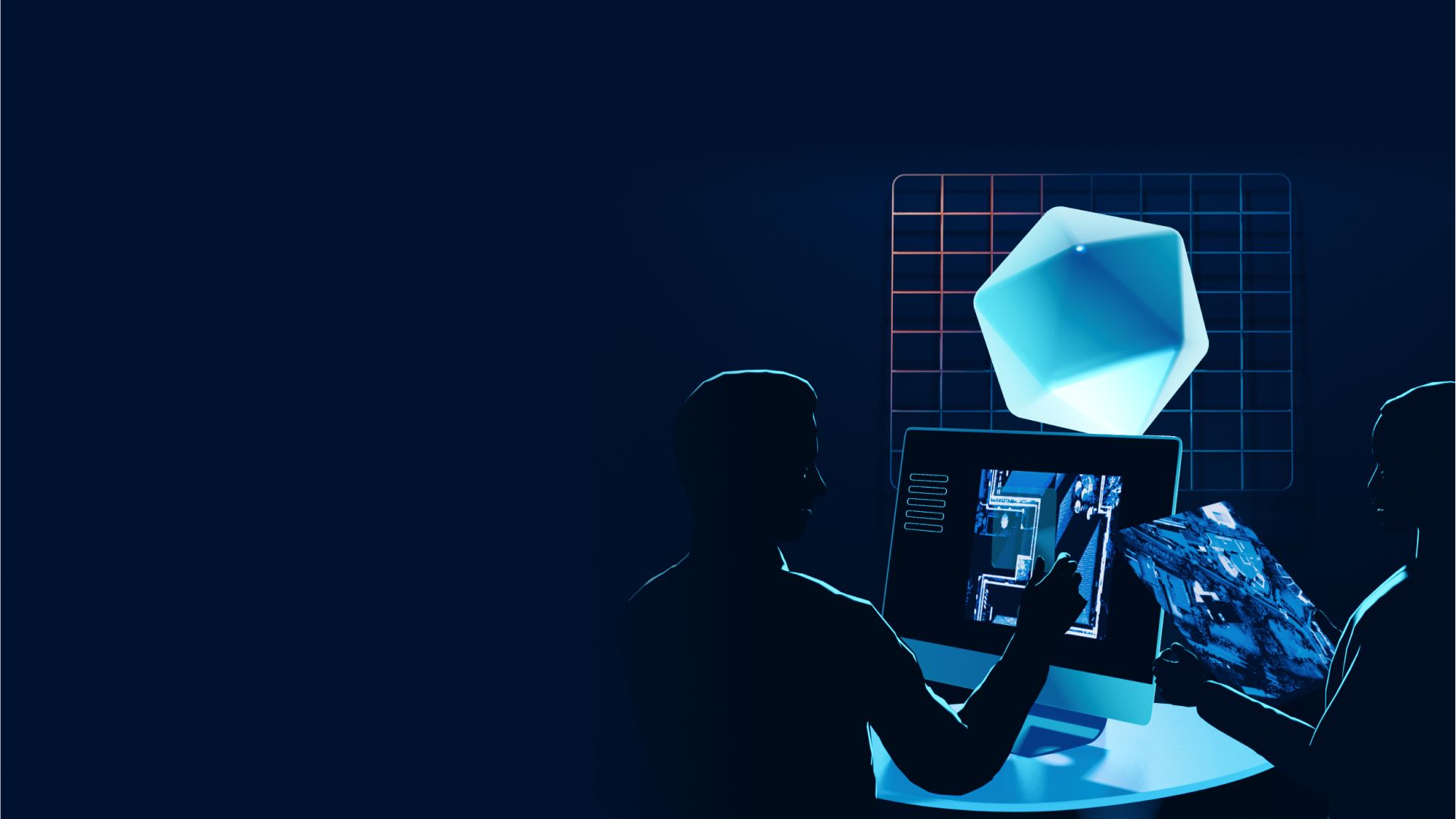
Deque has a storied journey on their mission of delivering digital equality to all. From the early days of the internet in the nineties, they made it their priority to pioneer and democratize digital accessibility. Digital accessibility is the practice of making digital documents, web and mobile apps accessible to everyone, including people with disabilities. Now, the power of machine learning is enabling the Deque team to lead the next generation of accessibility testing. Building out the components of their ML program has been challenging, but they have developed a sophisticated data engine that’s capable of prioritizing the most performant classes of data, discovering model errors quickly, and fueling their iterations with high-quality data.
In 2015, Deque made a bold decision to help advance automated accessibility testing. They decided to open source their most valuable asset, their accessibility testing rules library called axe-core. This rules engine powers Deque’s axe tools, the accessibility checker in Google’s Lighthouse and thousands of other notable projects around the globe amassing over half a billion downloads. Through automated testing alone, Deque’s axe can detect 57% of accessibility issues. While this might not sound like a lot if you’re new to this space, it’s more than double what the rest of the industry can offer and makes practicing digital accessibility a manageable task. To extend testing coverage even further, Deque turned to computer vision and machine learning to automatically catch even more accessibility issues, which were previously only detectable manually, using highly educated experts armed with various assistive technology and lots of time.
The Deque team is currently using their axe DevTools application to generate a pipeline of data that feeds into the machine learning system powered by Labelbox. The models that are generated are then being leveraged to expand coverage with an eventual target of being able to find 100% of accessibility issues in an automated fashion, thereby making true digital equality for all easy to achieve.
Challenge
It takes an average of about 10 minutes to fully annotate a single webpage screen. Between the thousands of web datasets and mobile datasets, the Deque team amassed a trove of useful data and looked to Labelbox and the Labelbox Workforce team for guidance and manpower. Prior to implementing Labelbox, the Deque team mostly relied on a combination of disparate open-source annotation tools, and hacking together Jupyter Notebooks with Google Sheets.
Solution
“Before using Model Diagnostics in Labelbox to target the model’s weaknesses, we had to visualize the predictions on our own and everything was much more manual,” said Noé Barrell, ML Engineer at Deque. “We had to calculate all these metrics on our own, and it was a disjointed and difficult process. Being able to convert this workflow into something we could now do simply within Labelbox made diagnosing errors a much more streamlined experience. It’s become so much easier to iterate.”
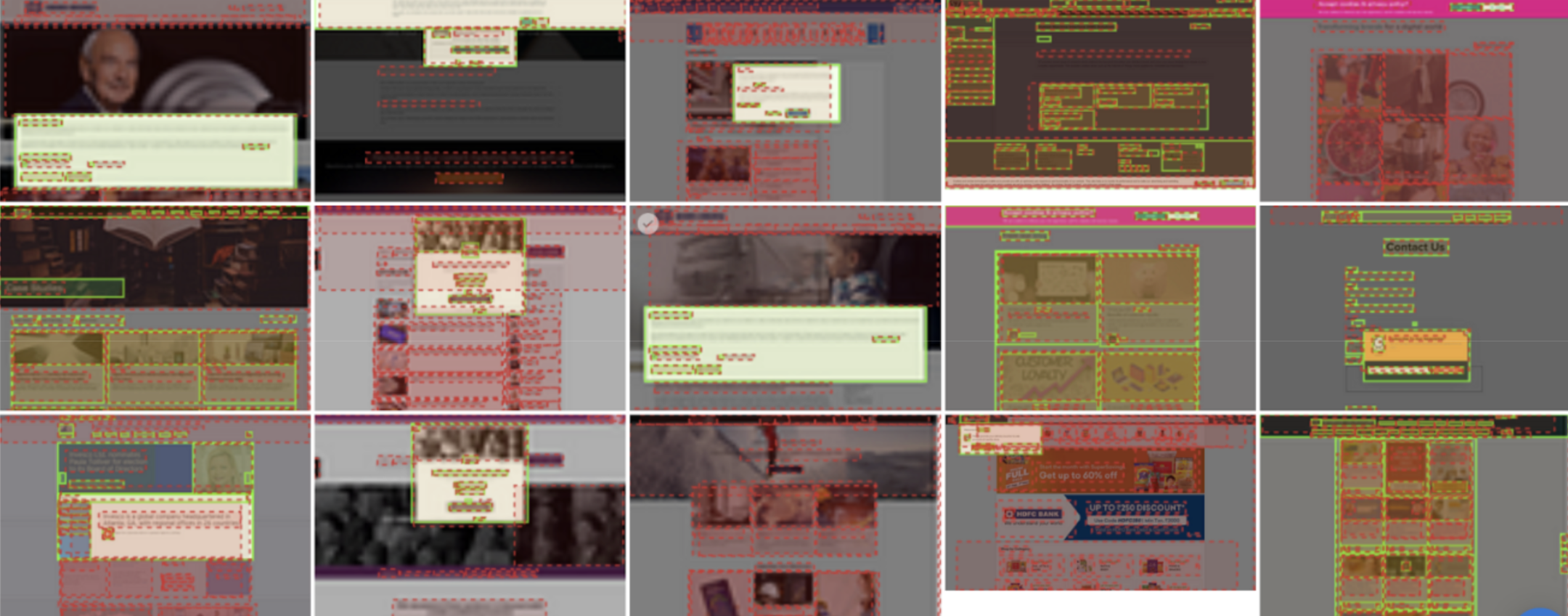
Noé and the ML team at Deque were able to make considerable improvements to model performance by seeing the ability to evaluate and visualize model performance. “We detected some noise issues in our dataset and thanks to Model Diagnostics, we were able to filter out about one-third of data points we considered less trustworthy,” said Barrell. “By doing so, model performance went up 5%. We re-labeled some data and we saw the performance went up again after we added the re-labeled points. It was challenging data for humans to label and for the model to understand. Being able to target the data we already had in Labelbox and make changes and fixes to it was really helpful to us as a team to save time and target where we knew it would make a difference in our model’s performance.”
The Deque team made huge leaps in several areas via Model Diagnostics and were able to target their data collection in a way that addresses model failures more quickly. For example, they boosted performance on classes of data such as improving detection of checkboxes from 47% accuracy to 75% accuracy, presentational tables from 66% accuracy to 79% accuracy, and radio buttons from 37.9% accuracy to 74% accuracy.
In another time saving measure, the Deque team found they could search, discover, and prioritize the right data with Catalog. “The Catalog feature in Labelbox is also huge for us. Pre-Catalog, for our data selection process, we’d look at the performance metrics of our model and let’s say, for example, we discovered it was indicating 50% accuracy on models, we would have to tediously and manually collect data surrounding that. But with the Catalog feature in Labelbox, we can target data collection for our models easily and quickly. Embeddings allow us to do unsupervised classification of models and select a lot of models. It’s just easier to create batches and sample around that. It takes a lot of the time and effort out of the data selection process,” said Barrell.
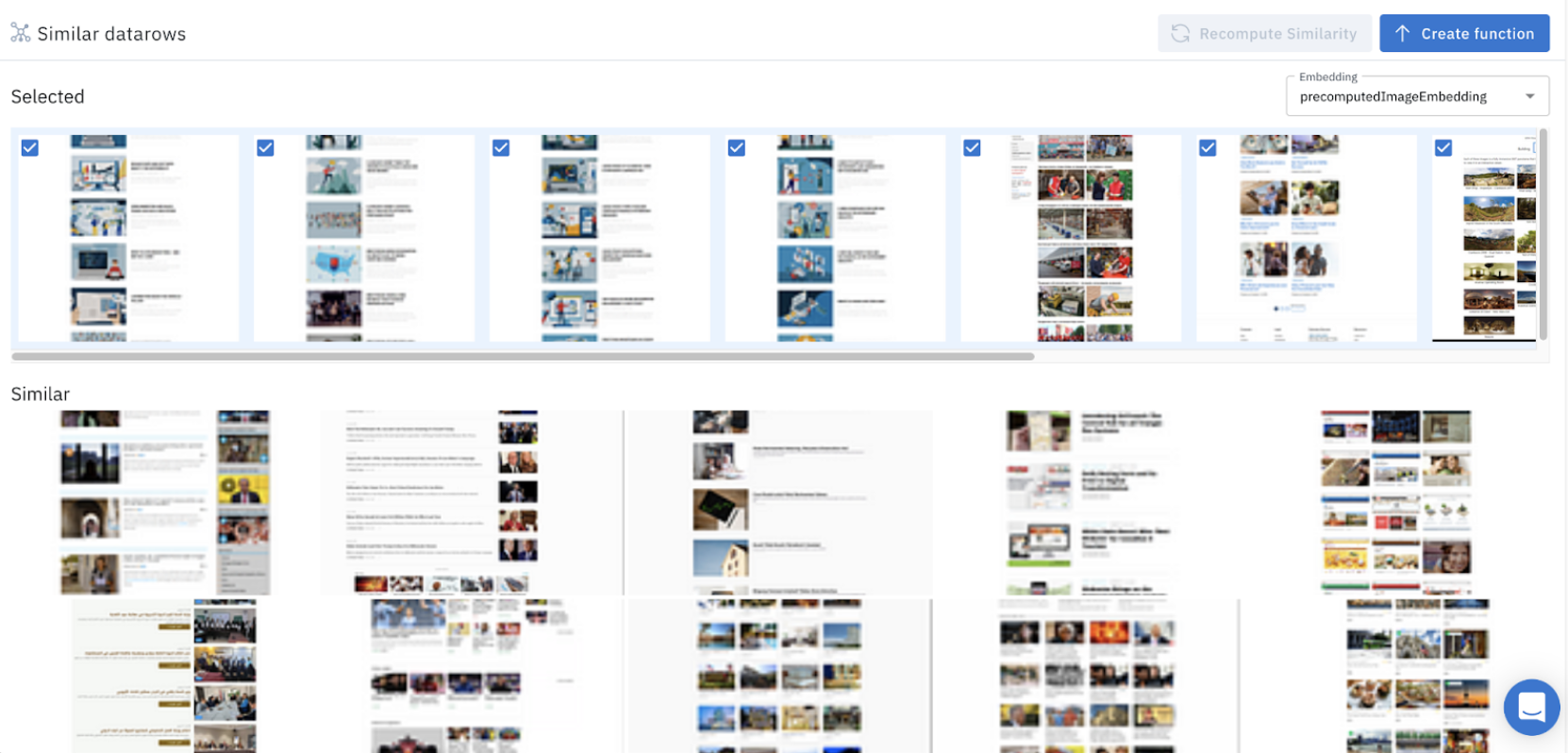
“Being able to reduce the data requirement is huge because you can see the same amount of improvement in the model’s performance in half the time and with half the effort. That was enabled through targeting the model’s weaknesses with Model Diagnostics and then being able to prioritize the right data through Catalog. Before, if we were doing a scattershot data collection, we would have been roughly labeling twice as much data and with twice as much effort,” said Barrell.
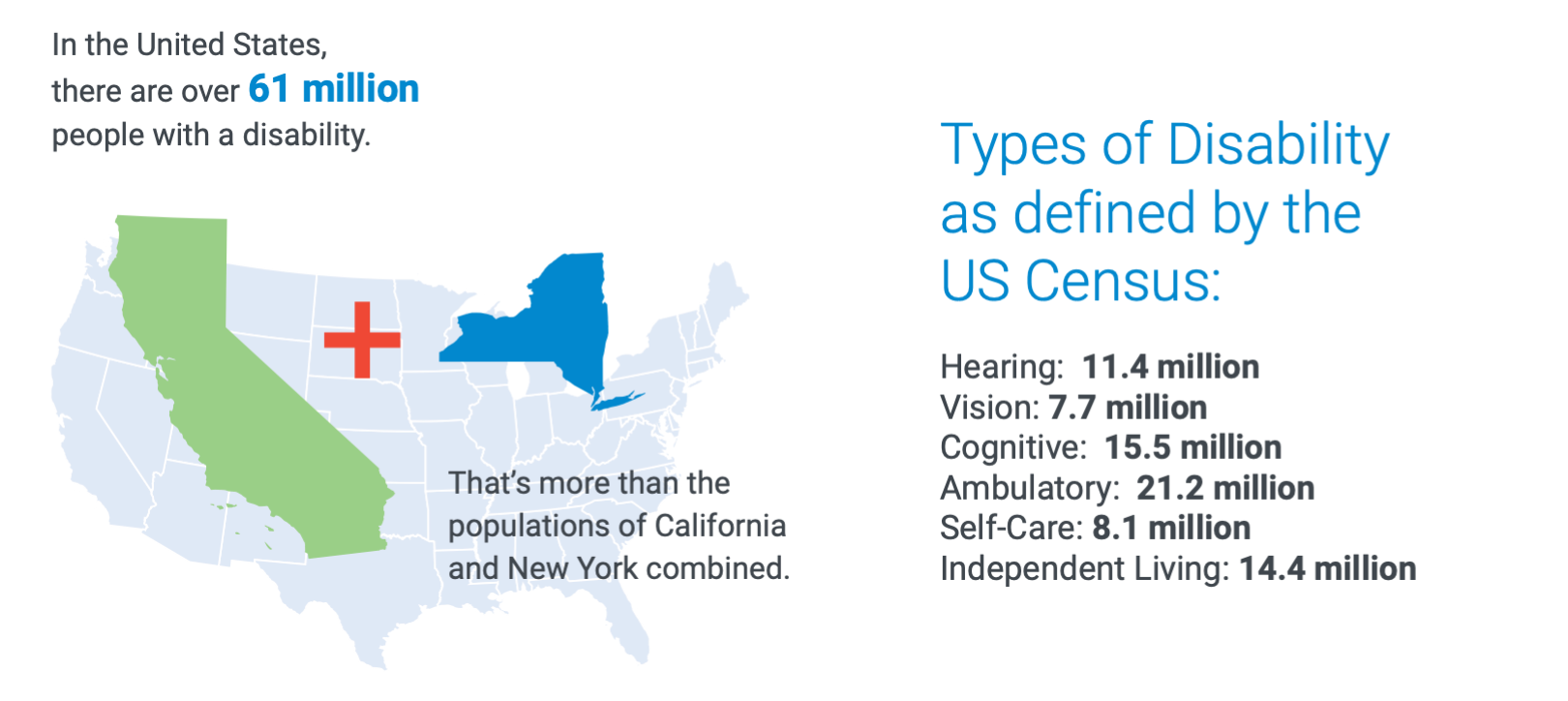
Building accessible sites or apps can be tricky without the right guidance. The Web Content Accessibility Guidelines, or WCAG, published by the W3C, is the standard that defines what makes an application accessible. Developers, testers, app owners and accessibility experts from around the world rely on these standards for proper accessibility testing direction. Not only is accessibility a requirement for compliance with many laws, but accessibility, by ensuring all information is available in text form, also increases your website’s SEO and can improve the user experience for all users. For example, video captions don’t just assist those with hearing impairments but can be used by viewers in noisy environments or in environments that are not conducive to listening to audio. Accessibility features are for everyone and create a more inclusive world.
To learn more about the Deque team and their mission, visit their website.
To understand how Labelbox can help you in your AI journey and help your team develop its own data engine, request a free demo now.