Labelbox•September 24, 2024
Labelbox leaderboards: Redefining AI evaluation with private, transparent, and human-centric assessments
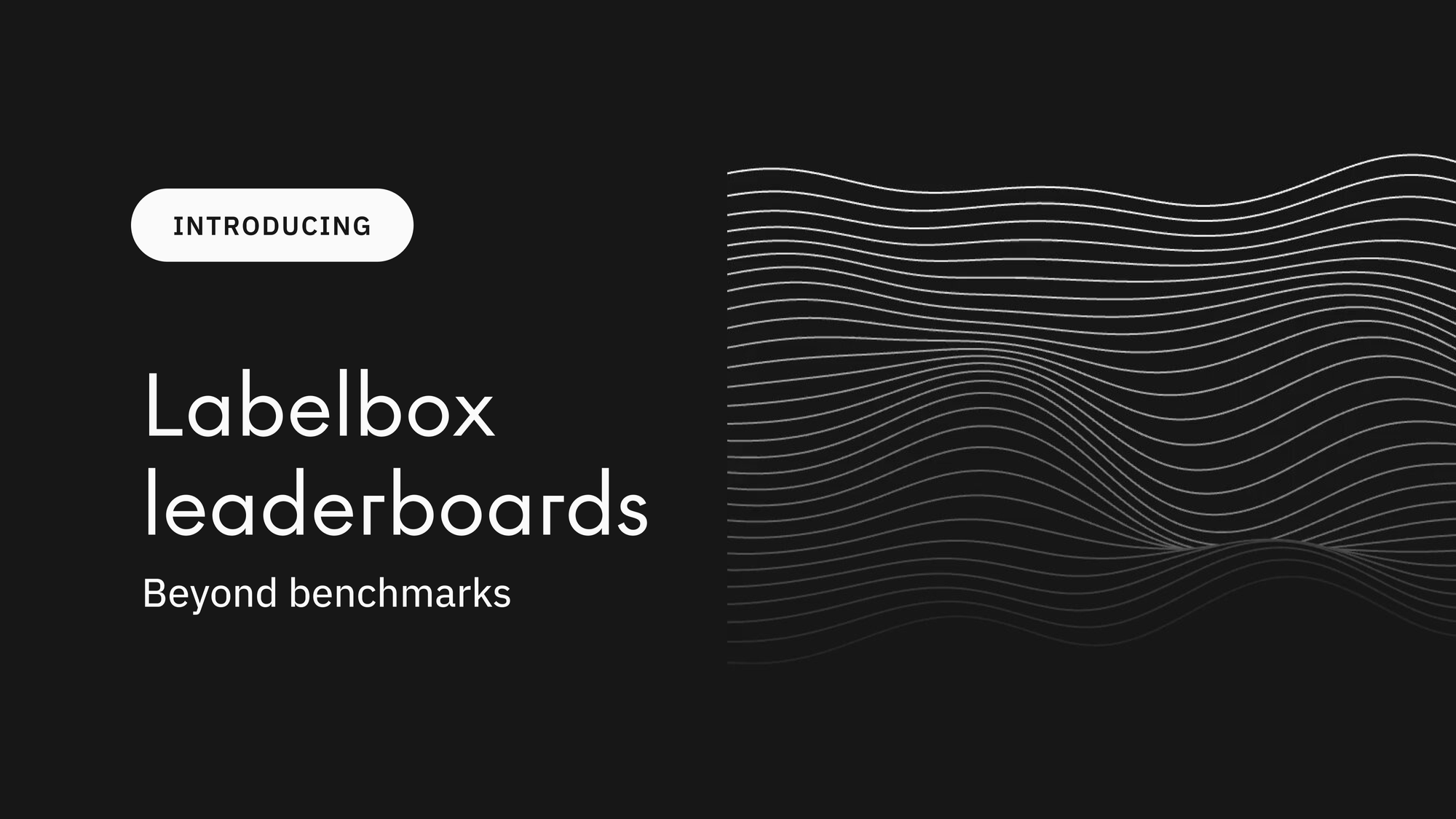
In the rapidly evolving landscape of artificial intelligence, traditional benchmarks are no longer sufficient to capture the true capabilities of AI models. At Labelbox, we're excited to introduce our groundbreaking Labelbox leaderboards — an innovative, scientific process to rank multimodal AI models that goes beyond conventional benchmarks.
The limitations of current benchmarks and leaderboards
Benchmark contamination
One of the most pressing issues in AI evaluation today is benchmark contamination. As large language models are trained on vast amounts of internet data, they often inadvertently include the very datasets used to evaluate them. This leads to inflated performance metrics that don't accurately reflect real-world capabilities. For example:
- The LAMBADA dataset, designed to test language understanding, has been found in the training data of several popular language models, with an LM Contamination Index of 29.3%.
- Portions of the SQuAD question-answering dataset have been discovered in the pretraining corpora of multiple large language models.
- Even coding benchmarks like HumanEval have seen their solutions leaked online, potentially contaminating future model training.
This contamination makes it increasingly difficult to trust traditional benchmark results, as models may be “cheating” by memorizing test data rather than demonstrating true understanding or capability.

Existing leaderboards: A step forward, but not enough
While several leaderboards have emerged to address the limitations of traditional benchmarks, they each come with their own set of challenges.
LMSYS chatbot arena
LMSYS Chatbot Arena, despite its broad accessibility, faces notable challenges in providing objective AI evaluations. Its reliance on non-expert assessments and emphasis on chat-based evaluations may introduce personal biases, potentially favoring engaging responses over true intelligence. Researchers worry that this approach could lead companies to prioritize optimizing for superficial metrics rather than genuine real-world performance. Furthermore, LMSYS's commercial ties raise concerns about impartiality and the potential for an uneven evaluation playing field, as usage data may be selectively shared with certain partners.
Scale AI's SEAL
Scale’s Safety, Evaluations, and Alignment Lab (SEAL), released few months ago, offers detailed insights/evaluations for topics such as reasoning, coding, and agentic tool use. However, the infrequent updates and primary focus on language models, while useful, may not capture the full spectrum of rapidly advancing multimodal AI capabilities.

Challenges in AI evaluation
These and other existing leaderboards all run into core challenges with AI evaluations:
1) Data contamination and overfitting to public benchmarks
2) Scalability issues as models improve and more are added
3) Lack of standards for evaluation instructions and criteria
4) Difficulty in linking evaluation results to real-world outcomes
5) Potential bias in human evaluations

Introducing the Labelbox leaderboards: A comprehensive approach to AI evaluation
The Labelbox leaderboards are the first to tackle these challenges by conducting structured evaluations on subjective AI model outputs using human experts and a scientific process that provides detailed feature-level metrics and multiple ratings. Leaderboards are available for Image Generation, Speech Generation, and Video Generation.
Our goal is to go beyond traditional leaderboards and benchmarks by incorporating the following elements:
1. Multimodal and niche focus
Unlike leaderboards that primarily focus on text-based large language models, we evaluate a diverse range of AI modalities and specialized applications, including:
- Image generation and analysis
- Audio processing and synthesis
- Video creation and manipulation
2. Expert human evaluation
For every evaluation, public or private, it’s critical for the raters to reflect your target audience. We place expert human judgment, using our Alignerr workforce, at the core of the evaluation process to ensure:
- Subjective quality assessment: Humans assess aspects like aesthetic appeal, realism, and expressiveness.
- Contextual understanding: Evaluators consider the broader context and intended use.
- Alignment with human preferences: Raters ensure evaluations reflect criteria that matter to end-users.
- Resistance to contamination: Human evaluations on novel tasks are less prone to data contamination.
3. Reliable and transparent methodology
We are committed to performing trustworthy evaluations using a variety of sophisticated metrics. Labelbox balances privacy with openness by providing detailed feature-level metrics (e.g. prompt alignment, visual appeal, and numerical count for text-image models) and multiple ratings.
In addition to critical human experts performing the evaluations, our methodology utilizes the Lablebox platform to generate advanced metrics on both the rater and model performance. We provide the following metrics across our three leaderboards:
- Elo rating system: Adapted from competitive chess, our Elo system provides a dynamic rating that adjusts based on head-to-head comparisons between models. This allows us to capture relative performance in a way that's responsive to improvements over time.
- TrueSkill rating: Originally developed for Xbox Live, TrueSkill offers a more nuanced rating that accounts for both a model's performance and the uncertainty in that performance. This is particularly useful for newer models or those with fewer evaluations.
- Rank percentages: We track how often each model achieves each rank (1st through 5th) in direct comparisons. This provides insight into not just average performance, but consistency of top-tier results.
- Average rating: A straightforward metric that gives an overall sense of model performance across all evaluations.
In addition to these key metrics, our methodology incorporates the following characteristics to ensure a balanced and fair evaluation:
- Expert evaluators: Utilizing skilled professionals from our Alignerr platform to provide nuanced, context-aware assessments.
- Comprehensive and novel datasets: Curated to reflect real-world scenarios while minimizing contamination.
- Transparent reporting: Detailed insights into our methodologies and results without compromising proprietary information.
4. Continuously updated evaluations
Our leaderboard isn't static; we plan to regularly update our evaluations to include the latest models and evaluation metrics, ensuring stakeholders have access to current and relevant information.

Leaderboard insights: A glimpse into model performance
To illustrate the power of our comprehensive evaluation approach, let's look at some recent data from our image generation model leaderboard:
1) Elo ratings:
- DALL-E 3 leads with 2825, followed by Flux 1.5 at 2763
- DALL-E 3 consistently outperforms other models in head-to-head comparisons
2) TrueSkill ratings:
- DALL-E 3 again leads with 1009.46, with Stable Diffusion 3 following at 988.26
- This indicates high expected performance for DALL-E 3 with relatively low uncertainty
3) Rank percentages:
- DALL-E 3 achieves the top rank 27.07% of the time, followed by Ideogram 2 at 23.07%
- This shows DALL-E 3's consistency in achieving top results, but also highlights Ideogram 2's strong performance
4) Average rank:
- Imagen 3 slightly edges out DALL-E 3 with an average rating of 2.88 vs 2.89 (lower is better)
- This suggests Imagen 3 performs well in direct comparisons despite lower Elo and TrueSkill ratings
These metrics provide a multi-faceted view of model performance, allowing users to understand not just which model is "best" overall, but which might be most suitable for their specific use case. For instance, while DALL-E 3 leads in most metrics, Imagen 3's strong average rating suggests it is a reliable choice for consistent performance across a range of tasks.

Join the revolution: Beyond the benchmark
The Labelbox leaderboards represent a significant advance in AI evaluation, pushing past traditional leaderboards by incorporating expert human evaluations for subjective generative AI models using comprehensive metrics. We are uniquely able to achieve this thanks to our modern AI data factory that combines human experts and our scalable platform with years of operational excellence evaluating AI models.
We invite you to:
- Check out the Labelbox leaderboards to explore our latest evaluations across various AI modalities and niche applications.
- Let us know if you have suggestions or want a specific model included in future assessments.
- Contact us to learn more about how we can help you evaluate and improve your AI models across all modalities.
Ready to go beyond the benchmark? Let's redefine AI evaluation — together—and drive the field toward more meaningful, human-aligned progress that truly captures the capabilities of next-generation AI models.