Mikiko Bazeley•July 2, 2024
What the focus on multimodal, generative, and 3D AI means for the future at CVPR 2024
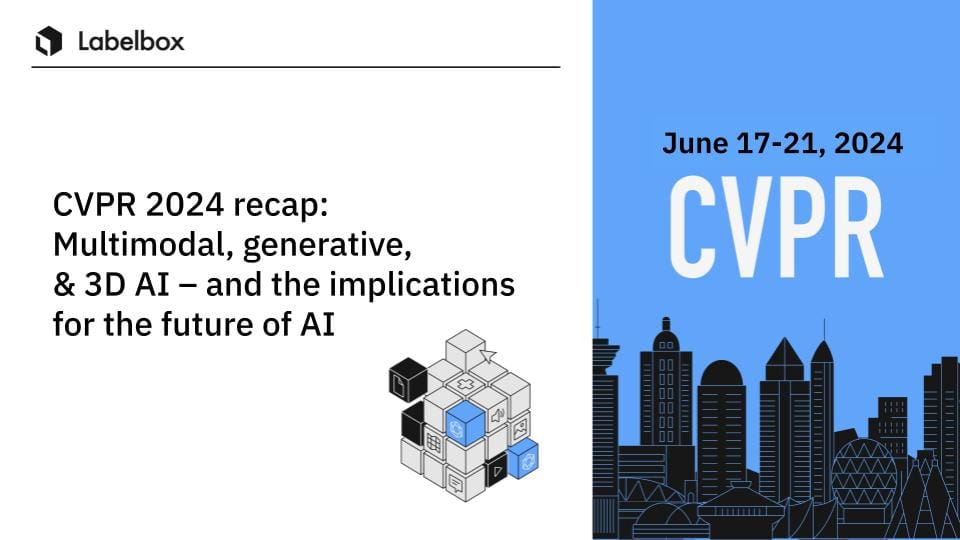
If CVPR is the pulse of SOTA, then innovation is stronger than ever
Reflecting on the key takeaways from CVPR 2023 and observing the advancements at CVPR 2024, it's evident that innovation in computer vision and AI is surging forward at an unprecedented pace. This year’s CVPR has been groundbreaking, breaking previous records with over 10,000 attendees and more than 2,500 research papers presented. This scale underscores the growing interest and investment in computer vision and pattern recognition research.
And if the sheer number of papers and attendees isn’t convincing enough, maybe all the tweets showing packed rooms, attendees zooming in from hallways because of capacity challenges, and the packs of attendees wearing orange and green CVPR badges does.
The most popular workshops according to attendees*
Each day we would take an informal survey of the hundreds of attendees that visited the Labelbox booth, asking the following question:
“Which workshop was your favorite or stood out the most?”
The clear winners were the following, all of which have recordings available (most likely because participants who weren’t lucky enough to make it in were encouraged to watch virtually).
The top workshops (in no particular order) were:
AI for 3D Generation
The workshop on generative models for 3D data focused on the development of algorithms capable of creating realistic, high-quality 3D content at scale. It aimed to address the challenges in generating and controlling complex 3D environments, including human interactions and scene dynamics. The discussions covered the latest advancements in 3D object synthesis, intuitive control mechanisms, realistic human actions, and the ethical implications of artificial 3D content generation. This session featured speakers from top institutions and companies, including the University of Oxford, Stanford University, Carnegie Mellon University, Meta AI, Stability AI, Snap Research, NVIDIA Research, Adobe Research, Columbia University, etc.
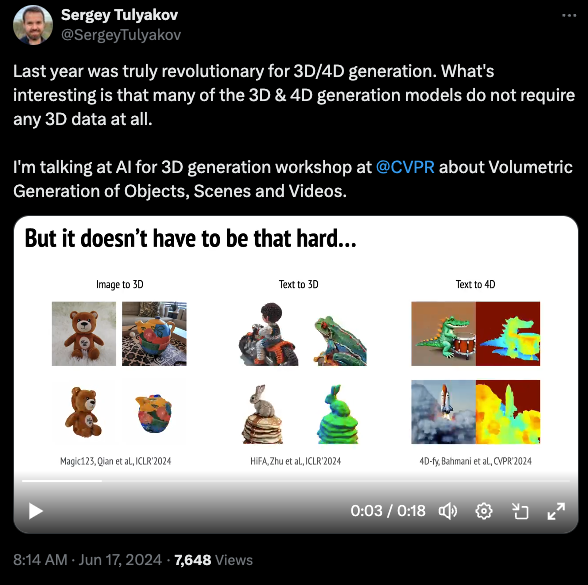
Towards 3D Foundation Models: Progress and Prospects
The workshop on 3D foundation models focused on developing scalable, generalizable, and adaptable 3D AI frameworks. It aimed to address the challenges of creating foundational 3D models for applications like 3D content creation, AR/VR, robotics, and autonomous driving. Discussions included necessary datasets, training tasks, architecture consensus, and potential applications. The session featured speakers from leading institutions and companies, including the University of Oxford, Stanford University, Columbia University, Technical University of Munich, UC San Diego, Adobe Research, KAUST, Allen Institute for AI, University of Toronto, CUHK Shenzhen, and Naver Labs.
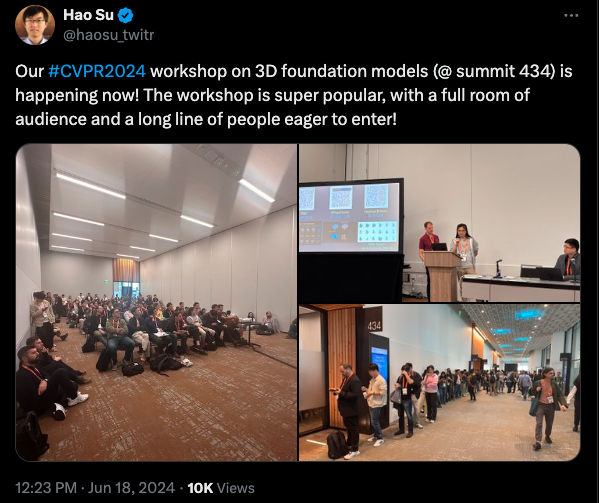
The Future of Generative Visual Art
The workshop on generative visual art, part of the 4th AI for Creative Video Editing and Understanding (CVEU) series, focused on advancing machine learning technologies for creative visual content creation and understanding. It aimed to explore intuitive video editing tools, human-AI collaboration, reducing production costs, and addressing AI biases. The session featured speakers from top institutions and companies, including OpenAI, Carnegie Mellon University, Adobe Research, RunwayML, DeepMind, ByteDance, Google, Meta, Pika, and Eyeline Studios.
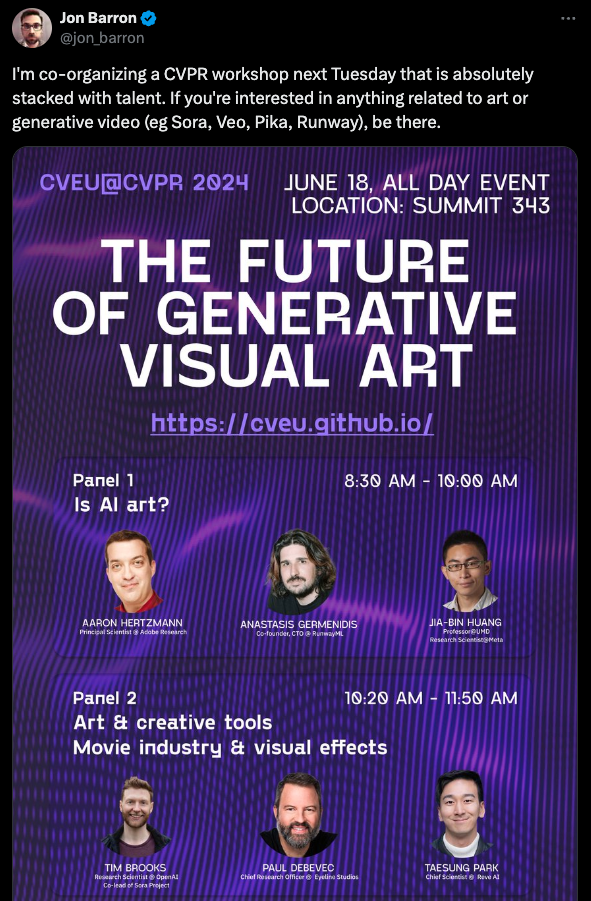
The 5th Annual Embodied AI Workshop
The workshop on Embodied AI focused on developing intelligent agents, such as robots, that interact with their environment to solve challenging tasks. It aimed to address the integration of perception, action, and reasoning in dynamic, unstructured settings. Discussions included topics like embodied mobile manipulation, generative AI for data and policy generation, and language model planning for task execution. The session featured speakers from leading institutions and companies, including AI2, Microsoft, Meta, Google, DeepMind, Stanford University, and 1X Technologies.
Additional tracks
Outside of the workshops, CVPR 2024 offered an extensive array of tracks and workshops, catering to various interests such as:
- Human Understanding: Tracks such as New Challenges in 3D Human Understanding and the Workshop on Human Motion Generation explored the latest research in understanding and modeling human actions and interactions.
- Medical Vision: The 9th Workshop on Computer Vision for Microscopy Image Analysis and the Foundation Models for Medical Vision workshop highlighted the integration of AI in medical imaging and diagnostics.
- Mobile and Embedded Vision: The 4th Mobile AI Workshop and Challenges and the Embedded Vision Workshop addressed the unique challenges and innovations in mobile and embedded AI applications.
- Neural Rendering: Tracks such as the 1st Workshop on Neural Volumetric Video and Neural Rendering Intelligence explored the cutting-edge developments in neural rendering technologies.
- Open World Learning: The VAND 2.0: Visual Anomaly and Novelty Detection workshop and the Visual Perception via Learning in an Open World workshop examined the challenges and methodologies for learning in open-world environments.
- Physics, Graphics, Geometry, AR/VR/MR: Workshops like the 4th Workshop on Physics Based Vision meets Deep Learning and the Computer Vision for Mixed Reality workshop showcased the intersection of computer vision with physical simulations, graphics, and mixed reality applications.
A significant number of workshops were recorded this year, so be sure to grab a digital pass if you weren’t able to attend.
High-quality data is the secret ingredient in the race for the next frontier models by AI labs
Alongside our samples of High Brew coffee, common discussions overhead at the booth included:
- The importance of synthetic data in overcoming the hurdles and expenses of annotating large datasets.
- Palpable excitement about new video models and the combined modeling of video and audio, reflecting a keen interest in multimodal techniques.
- As well as a resurging sense of optimism about progress in robotics.
Attendees were also avidly discussing using both supervised and unsupervised data to mitigate data scarcity, revealing innovative approaches within the field (as well as an appetite for high-quality data).
Labelbox is at the forefront of supporting these trends with visitors highly interested in our newest multimodal chat solution, which integrates advanced tooling and managed services to facilitate the creation, evaluation, and optimization of model responses across diverse data modalities. By offering robust tools and services with cross-modal support (such as text, images, videos, audio, and documents), AI researchers and enterprises are enabled to accelerate model building and refinement.
Labelbox's new services model provides access to a network of highly-skilled experts, ensuring high-quality, human-evaluated data essential for Reinforcement Learning with Human Feedback (RLHF) and Supervised Fine Tuning (SFT).
Alignerr, a community of subject matter experts, further complements these efforts by generating high-quality data to enhance AI models' safety, reliability, and accuracy. This collaboration between advanced platforms and expert networks underscores the transformative potential of integrating multimodal support and human expertise in the development of next-generation generative AI systems.
What needs to happen over the next 6-9 months based on CVPR 2024
The future of AI is undeniably multimodal, extending to the data layer itself.
For foundation model developers and early innovators, each wave of generative AI has emphasized the critical importance of training data quality and availability. Initially, it was unstructured text data for LLMs, then image data for generative vision models, and now prompt-response data for visual language models.
As new foundation models are integrated into user-facing products, measuring and evaluating quality has become a key challenge. Developers must address questions like what constitutes good quality, how to train models to evaluate prompts and responses, and how to efficiently incorporate human expertise to ensure high-quality labels and responses. Systematically enforcing and improving quality across multiple modalities without wasting resources on fragmented tools and services is essential for progress in this field.
Labelbox plays a crucial role in supporting AI labs with high-quality data, enabling the development of advanced multimodal generative AI models.
With a network of global expert labelers and a comprehensive suite of tools, Labelbox offers a fully managed service tailored to specific AI use cases. Our live multimodal chat editor allows users to compare model outputs side-by-side, while prompt and response generation tools create datasets for fine-tuning tasks.
Additionally, preference, selection, and ranking features incorporate human-in-the-loop (HITL) processes to ensure nuanced, high-quality labels for reinforcement learning with human feedback (RLHF).
This integrated approach helps AI labs systematically enforce and enhance data quality across various modalities, accelerating model development and deployment.
While current multimodal AI solutions often fall short of integrating multiple data types effectively, Labelbox addresses these challenges by providing seamless tools and expert support, ensuring robust and reliable multimodal AI systems.
Ready to be a part of the future of generative-AI?
Sign up for a free Labelbox account to try it out or contact us to learn more and we’d love to hear from you.
If you're interested in joining Labelbox, check out our careers page.