Labelbox•June 6, 2022
Why your ML team needs a data engine
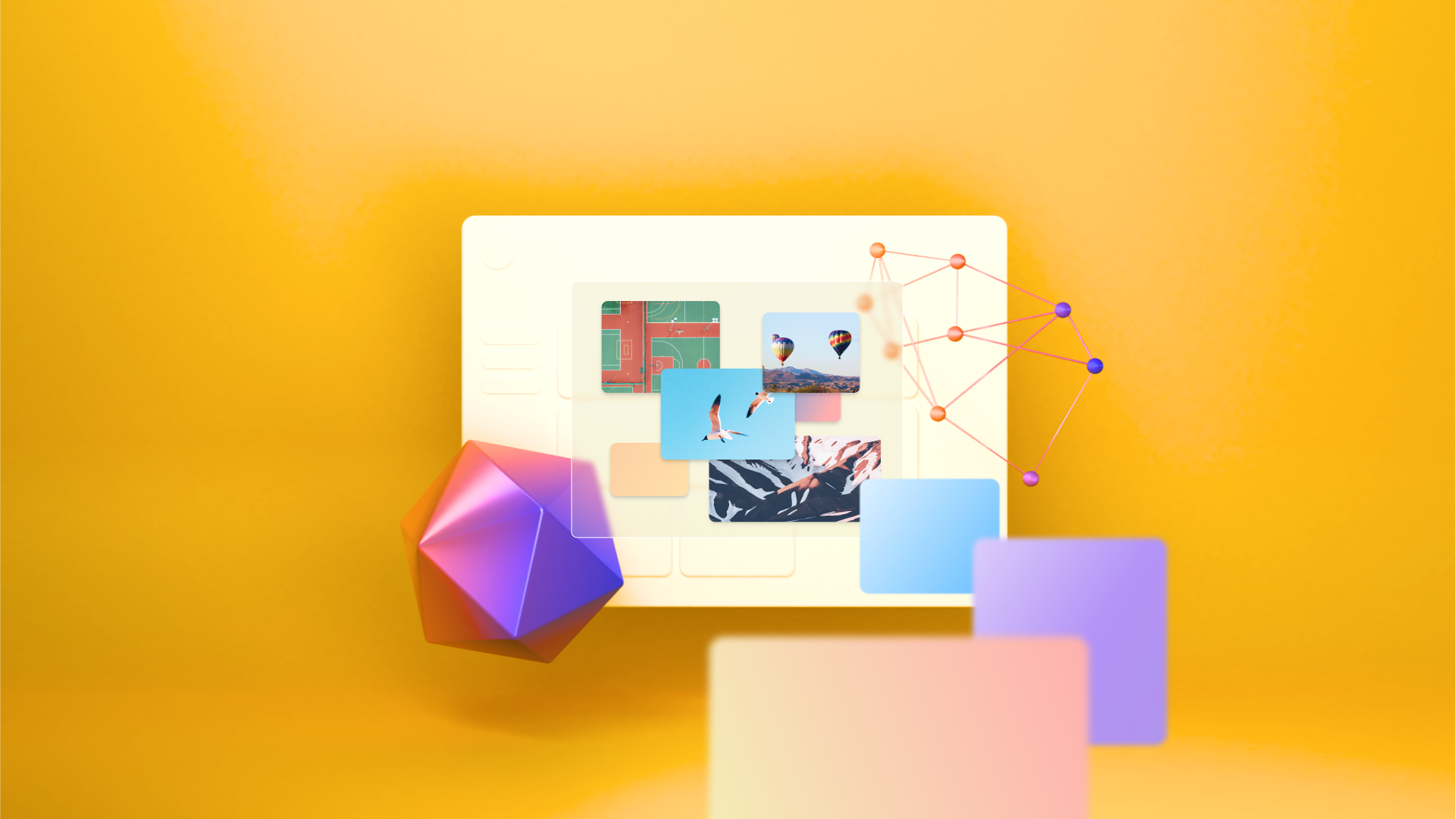
Machine learning teams today face several common challenges, including the need to produce better quality training data, increase the speed of iterations on their models, and help their organization build competitive advantage quickly with performant AI. A seamless and efficient data engine can help teams building even the most complex models tackle these tasks.
What is a data engine?
An AI data engine is the foundational infrastructure for how team members interface with data and models in order to build better AI products — and do it remarkably fast. The ideal data engine ensures that whenever humans interface with data, they can do so easily and efficiently, and that programmatic solutions and automations are set in place to keep data moving quickly through these workflows.
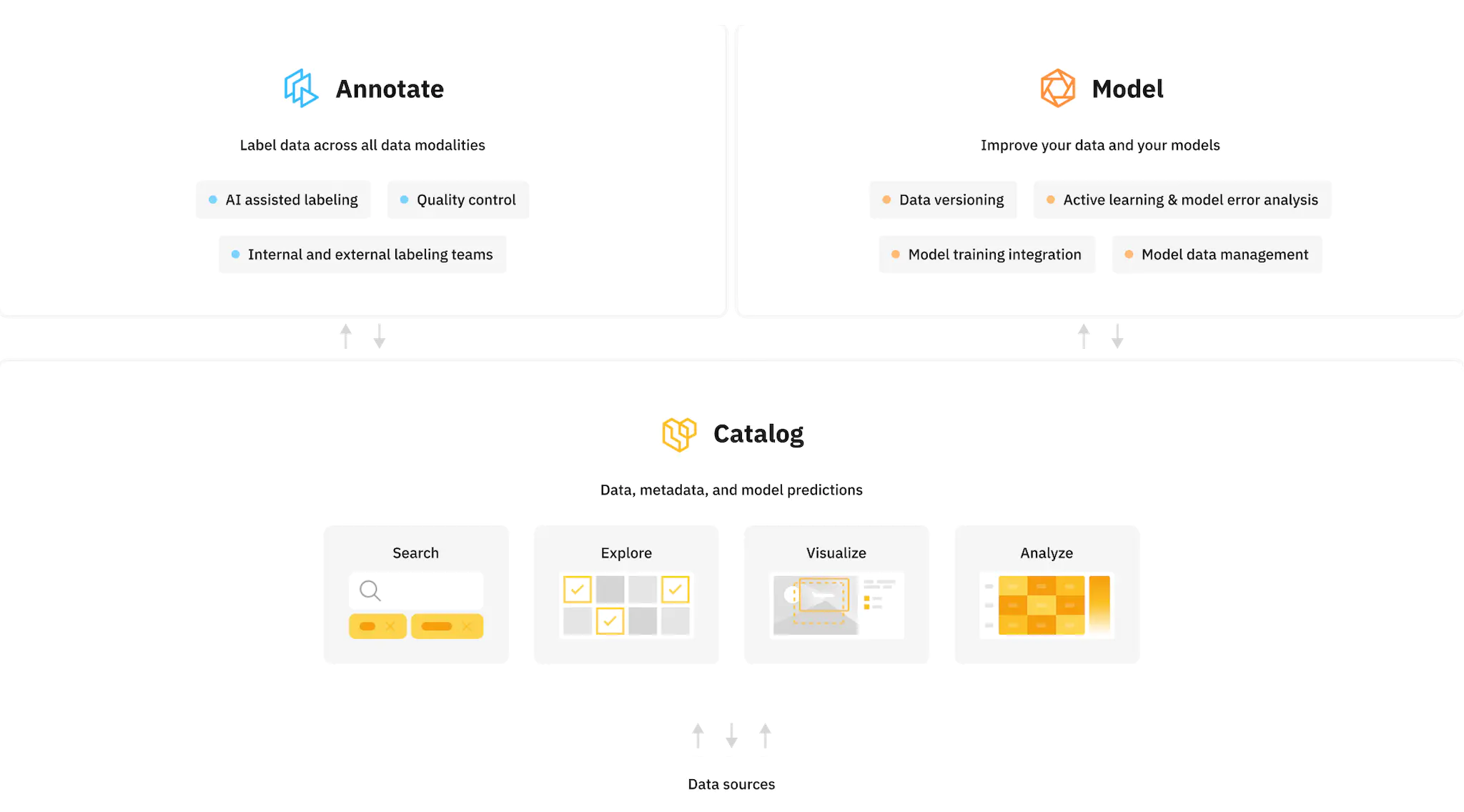
Read on to learn how a data engine can help your team save time throughout the training process and deliver more measurable ROI for your ML efforts.
Data engines generate quality training data faster
For teams taking a data-centric approach to AI — a technique proven to increase model performance — high-quality training data is a priority. However, the quality management workflows required to produce this training data can add days or even weeks to the timeline. A data engine powered by a training data platform can easily integrate workflows such as consensus, benchmarking, and review queues, blending them seamlessly into your labelers’ work. By eliminating delays caused by manual data transfers, miscommunications, and time spent waiting for the next asset to label or review, a data engine can help teams produce quality training data in record time.
The quality of training data also depends as much on the efficacy of the data that was labeled as the quality of its annotations. The closed loop system of a data engine ensures that the performance of the model in training informs which assets are labeled next. This active learning technique enables ML teams to produce smaller training datasets that significantly improve model performance. Because the datasets labeled with this technique are smaller, the labeling process moves fast while reducing costs and labeling budgets.

Data engines enable teams to iterate faster and more efficiently on their models
In AI, just as it is in software, the ability to iterate quickly on a product is usually a marker for success. While code can be written, tested, and revised in a matter of minutes or hours, the iteration cycle for ML has historically been much slower, taking weeks – and sometimes months. Typically, the slow pace of labeling and reviewing training data is the main roadblock to a rapid iteration cycle.
A data engine that empowers teams to create high-quality training data quickly enables ML teams to pick up the pace of their iterative cycles and train accurate models. Systems that use the active learning technique discussed in the section above will also train models more efficiently. While traditional training techniques can result in diminishing returns with late stage iterations even with a training dataset exponentially larger than those used in the first few iterations, active learning can ensure that models make significant leaps in performance with every iteration — and with less training data.
ML teams equipped with an efficient data engine are also able to maintain model performance by retraining them quickly whenever necessary. Models in production can be updated efficiently without causing long delays and interruptions in service.
Data engines help ML teams build competitive advantage for their organizations
With AI proliferating every industry and department, it’s no longer enough for organizations to use (or even lightly adjust) off-the-shelf models and publicly available datasets to gain and maintain competitive advantage. They must develop and train models of their own or make significant alterations to existing models. Enterprises are quickly discovering that their best results are from AI trained on their own proprietary data, as these models perform better for their specific use cases.
However, even AI teams building models from scratch and training them on data considered valuable IP can struggle to build competitive advantage for their organizations when their iteration cycle moves at the same pace (or a slower one) than their competitors. Teams equipped with a data engine can not only produce performant models faster; they can improve on them until the models become impossible to reproduce by any competing team, even if they have the same original model and similar training data.
“The argument for building a data engine goes beyond just improving model performance. For many companies, it’s become their competitive moat. If a competitor emerges that wants to create a similar product, they have a hard time replicating what you do because you have a data engine at work that’s been improving your product from the beginning. They can’t copy that.” — Peter Welinder, VP of Product and Partnerships, Open AI
A rapid iteration cycle is not only key to building a performant AI product; it’s integral to building a one-of-a-kind model that continues to outperform its peers in competing organizations.
To learn more about how you can build a data engine that best serves your AI team and organization, watch our webcast featuring Labelbox CEO and Cofounder Manu Sharma and OpenAI VP of Product and Partnerships Peter Welinder: How to build a data engine for your organization. You can also discover more about what data engines offer in our ebook entitled, "The complete guide to data engines for AI."