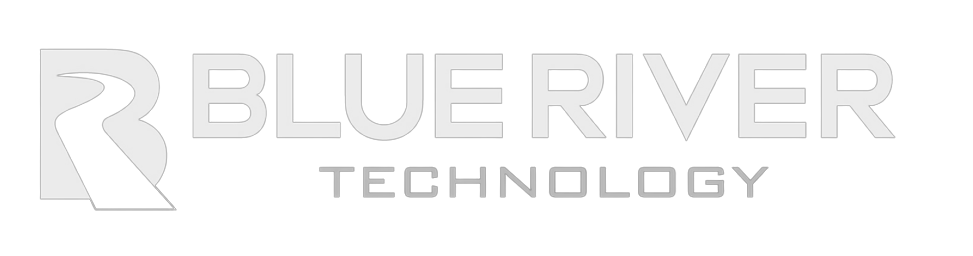
How Blue River Technology used model-assisted labeling to reduce labeling costs by 50%
Problem
High labeling costs associated with the costs of crops vs. weeds on full image segmentation images.
Solution
Labelbox’s model-assisted labeling and collaborative annotation suite.
Result
The company is now able to standardize how they create and manage data all in a single location and using Labelbox's automation suite, they’ve seen a reduction in labeling times by 50%, worth millions of dollars in cost savings per year.
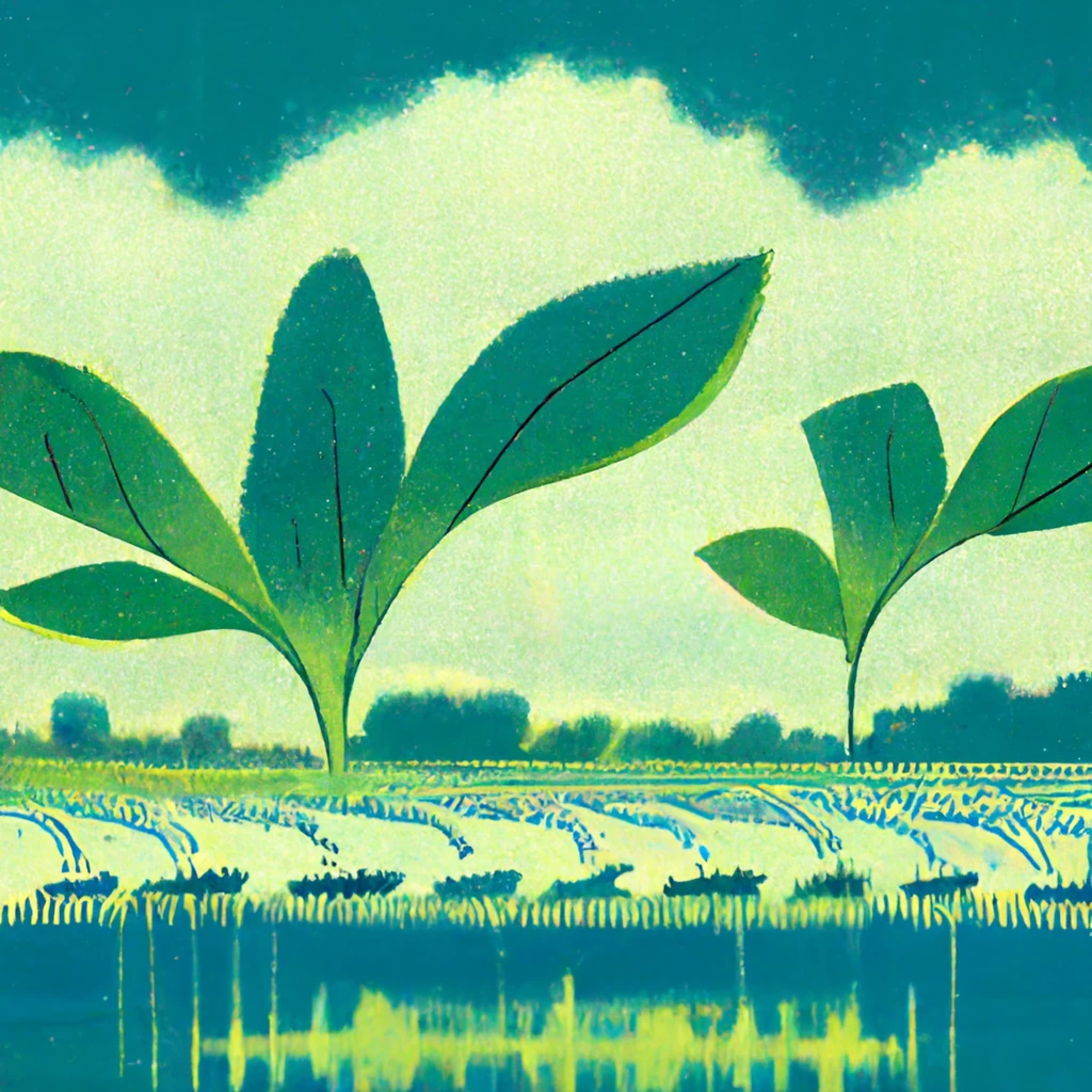
Blue River Technology is building the next generation of smart agriculture equipment and enabling a world of sustainable farming through robotics and computer vision. Farmers struggle with combating weeds that take away important nutrients and reduce the likelihood of the plants growing to full maturity. A difficult tradeoff exists between doing a broad automated spray which can potentially damage crops and having human labor spray the weed instances which can be costly and manually intensive.
Blue River’s See & Spray technology solves this problem by using smart machines and computer vision to help farmers optimize for their spending and usage of herbicides in the field. The backend technology behind See & Spray are advanced machine learning models that are able to correctly identify weed versus crop instances. The goal is simple: Preserve valuable crops and spray the weeds.
By using model-assisted labeling, annotators would only have to correct the errors that Blue River’s model makes which is far quicker and easier than starting from an image with no masks. With masks already present, human attention is drawn to common problem areas for the model: the edges and differentiating weed and crop within contiguous segments of organic material. Blue River recently achieved a productivity breakthrough by improving the training data that is fed into their machine learning models; a system that is trained up using hundreds of thousands of images containing crops and weeds.
The Blue River team was able to cut down the labeling time drastically by over 50%, In addition, their labeling team could now focus on correction rather than labeling from scratch; merely correcting classes and labels as opposed to hand labeling the majority of images. Blue River’s team annotated these images using image segmentation, which is the most complex and intricate of image labeling tasks as it required each plant to have a specific class.
The ultimate goal of Blue River’s technology is to reduce herbicide use by 70-80% since it is one of the costliest line items in a modern farmer's budget. Further gains will come from leveraging complementary technologies inside the Labelbox platform such as active-learning and additional automation.