How Cape Analytics uses active learning to get to production AI faster
Problem
In edge case geographic areas, models can be confused by natural features and incorrectly tag objects (such as yard debris, trees, etc) which leads to inccurate predictions and low performant models. Collaborating on fixing these edge cases is a complex challenge which requires countless hours of coordination and communication between many stakeholders.
Solution
The Cape team ran an iterative active learning cycle within their model training pipeline, determining areas of low model confidence and then prioritizing those areas for additional labeling via Labelbox. This iterative cycle increased overall model accuracy in a targeted manner. From their experience, specifying where a model may be uncertain allowed for more rapid fixes which led to more performant model outcomes.
Result
The aggregate time saved from utilizing Labelbox’s active learning workflows represented an estimated 30%+ increase in total time savings, as well as months of custom development work in engineering hours.
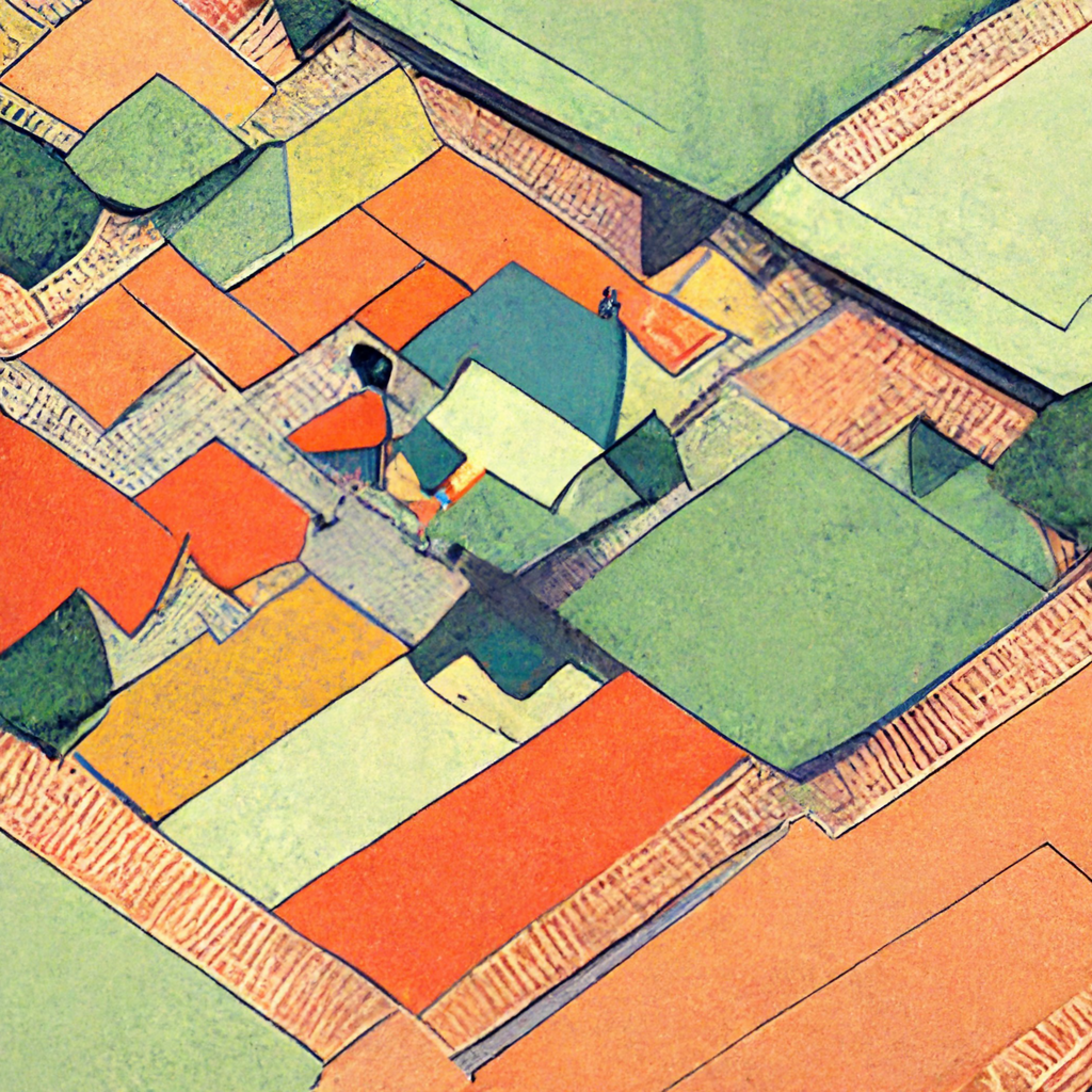
Cape Analytics enables insurers and other property stakeholders to access valuable property attributes during underwriting, by using computer vision algorithms to extract information from geospatial imagery. The power of this solution lies in combining the accuracy and detail of property information traditionally relegated to in-person inspections, with the lightning speed of a living database covering the entire property base of the US, and delivering this information in a matter of seconds.
The Cape team wanted to explore ways to speed up labeling times and found active learning tools to be particularly useful for accomplishing this goal. By creating a labeling workflow whereby low confidence predictions were visible and prioritized to their data scientists and labelers, their team was able to quickly analyze and correct labels.
One vivid example involves the Cape team’s efforts to identify yard debris. It’s important to identify debris on residential properties because it typically results in higher risk from an insurance point of view. However, it is challenging to build training models that correctly identify such materials because the taxonomy is difficult to explain to an annotation team—for example, it can be hard to distinguish debris from yard furniture that isn’t neatly arranged, or construction materials lying around. In fact, by managing their iteration cycle via Labelbox, the Cape team discovered that in some geographic areas, models could be confused by natural features and incorrectly tagged these objects as yard debris.
To solve this problem, the Cape team ran an iterative active learning cycle within their model training pipeline, determining areas of low model confidence and then prioritizing those areas for additional labeling via Labelbox. This iterative cycle increased overall model accuracy in a targeted manner. From their experience, specifying where a model may be uncertain allowed for more rapid fixes which led to more performant model outcomes.
Showing labelers lower confidence areas also required well-designed queue management systems because of the complexity in automatically routing labeling tasks to the right team members. The aggregate time saved from utilizing Labelbox’s queue management systems in tandem with active learning cycles, represented an estimated 30%+ increase in total time savings, as well as months of custom development work in engineering hours.
“There are many labeling tools out there but the Labelbox backend is the real differentiator. With dynamic queueing, our labelers are never out of work, which was a major upgrade compared to our old internal tools — both from a productivity and speed-to-production point of view.” - Cape Analytics, Head of Engineering
Cape Analytics’s finely tuned approach to defining risk is one of the key reasons the company is growing so quickly. Since their founding, Cape Analytics applied rigorous deep learning model building techniques to geospatial imagery in order to build impactful solutions for insurance and beyond.