Genentech develops breakthrough labeling process for medical imagery ML
Problem
Early clinical development team launched AI initiatives in 2019 and needed advanced quality workflows for training data & a highly secure infrastructure in place immediately to enable a faster go-to-market motion.
Solution
Labelbox’s collaborative platform and flexible/secure deployment options.
Result
Effectively scaled training data operations by allowing subject matter experts to teach labelers what to look for; Genentech is now producing labeled data that is 10x cheaper, 5x faster & with better quality.
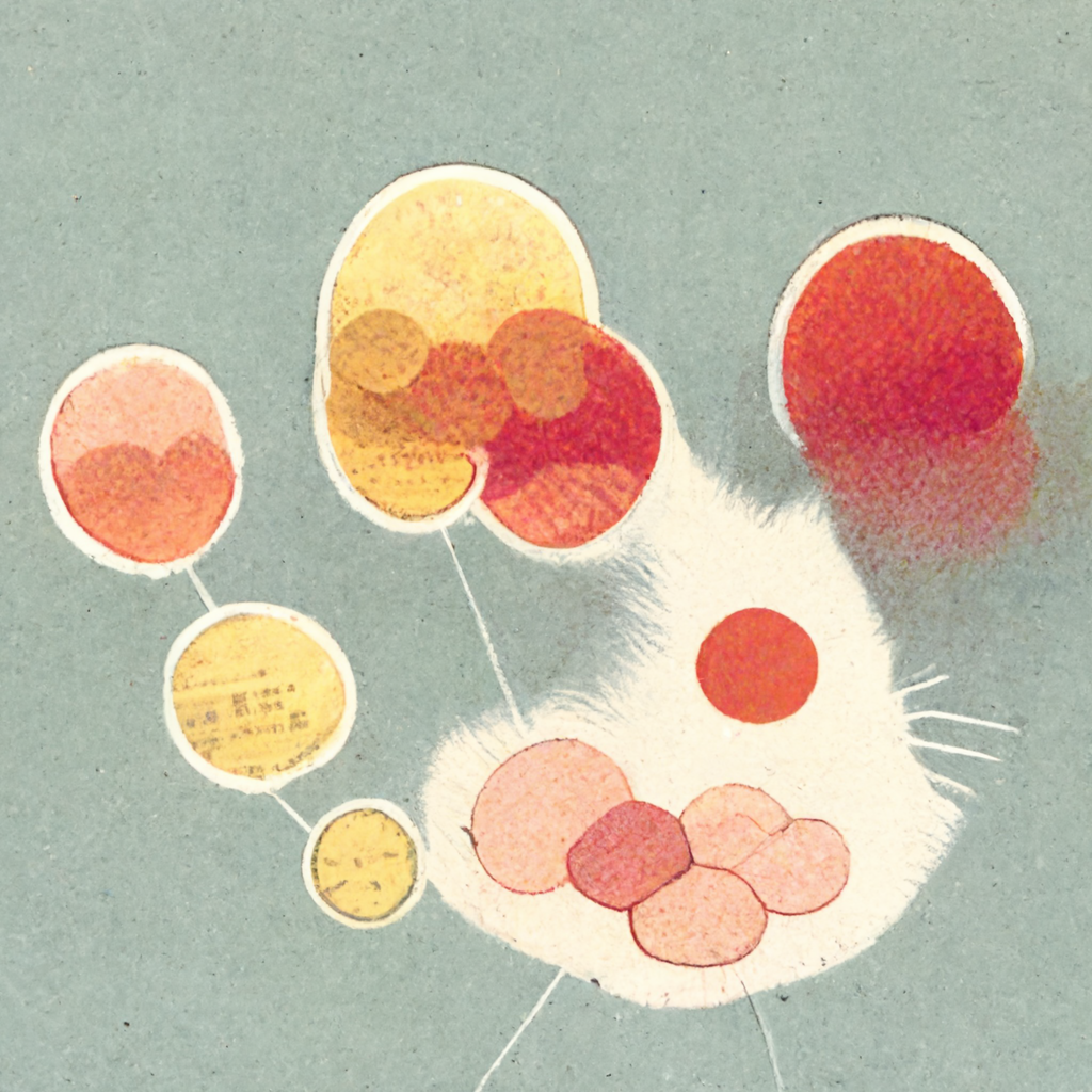
Genentech is a biotechnology enterprise that has developed breakthroughs in medical research and medicine since 1976. Today, Genentech researchers are building convolutional neural networks to help diagnose illnesses and aid medical professionals.
While traditional, classic algorithms can perform when faced with "perfect" data, actual patient data is often riddled with small abnormalities. For example, images of a perfect retina are easily analyzed by a classical algorithm, but a real patient's retina might have multiple lesions, signs of illness, and other issues. Training deep learning algorithms to find and classify images taken from real patients often requires meticulously labeled medical imagery numbering in the hundreds and even thousands.
Typically, only trained medical experts are trusted to correctly annotate training data for these use cases, as inaccurate model predictions can result in misdiagnosis and even loss of life. Labeling the required amount of data this way, however, is a costly and time-consuming endeavor.
Genentech adopted Labelbox to revolutionize their ML process by having domain experts train teams of labelers on medical imagery annotation tasks. With regular labelers creating annotations, which are then sampled and reviewed for quality by experts, Genentech has now developed a much faster and less expensive way to create training data and get their life-saving algorithms into production.