Labelbox•April 14, 2023
Auto-Segment 2.0 powered by Meta's Segment Anything Model
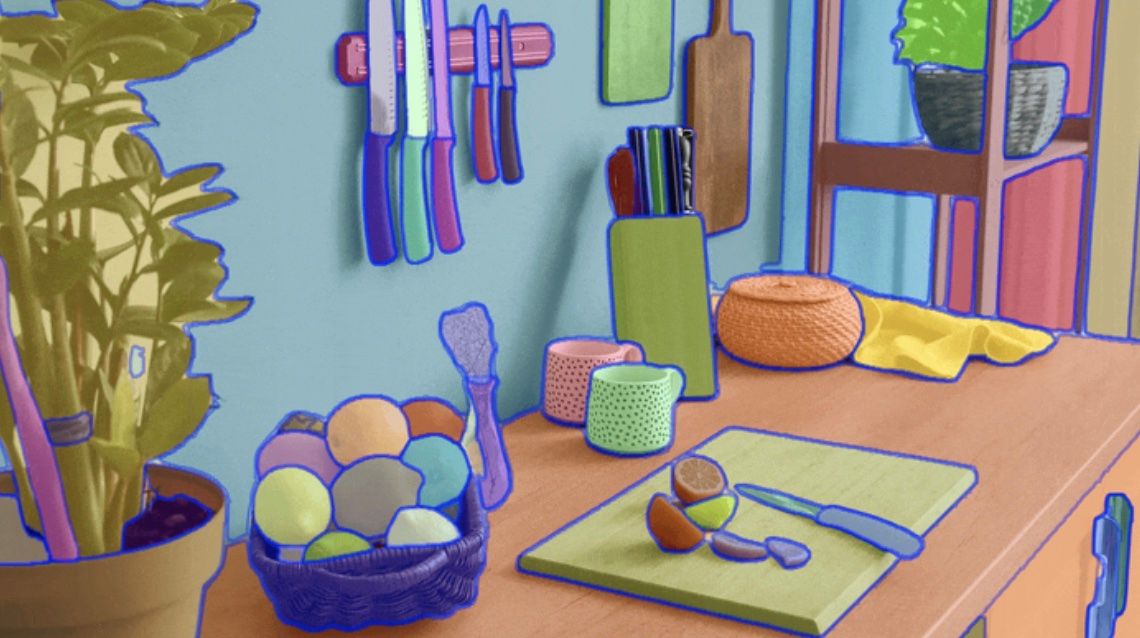
Image segmentation is one of the most challenging and manually cumbersome labeling tasks. To train semantic segmentation models, many teams traditionally use a pen tool to manually trace the outline of an object. Not only is this method painfully slow, but it typically results in errors that negatively impact downstream model performance. These costs compound as labeling teams complete this cumbersome and error-prone workflow for hundreds of objects — sometimes in one single image.
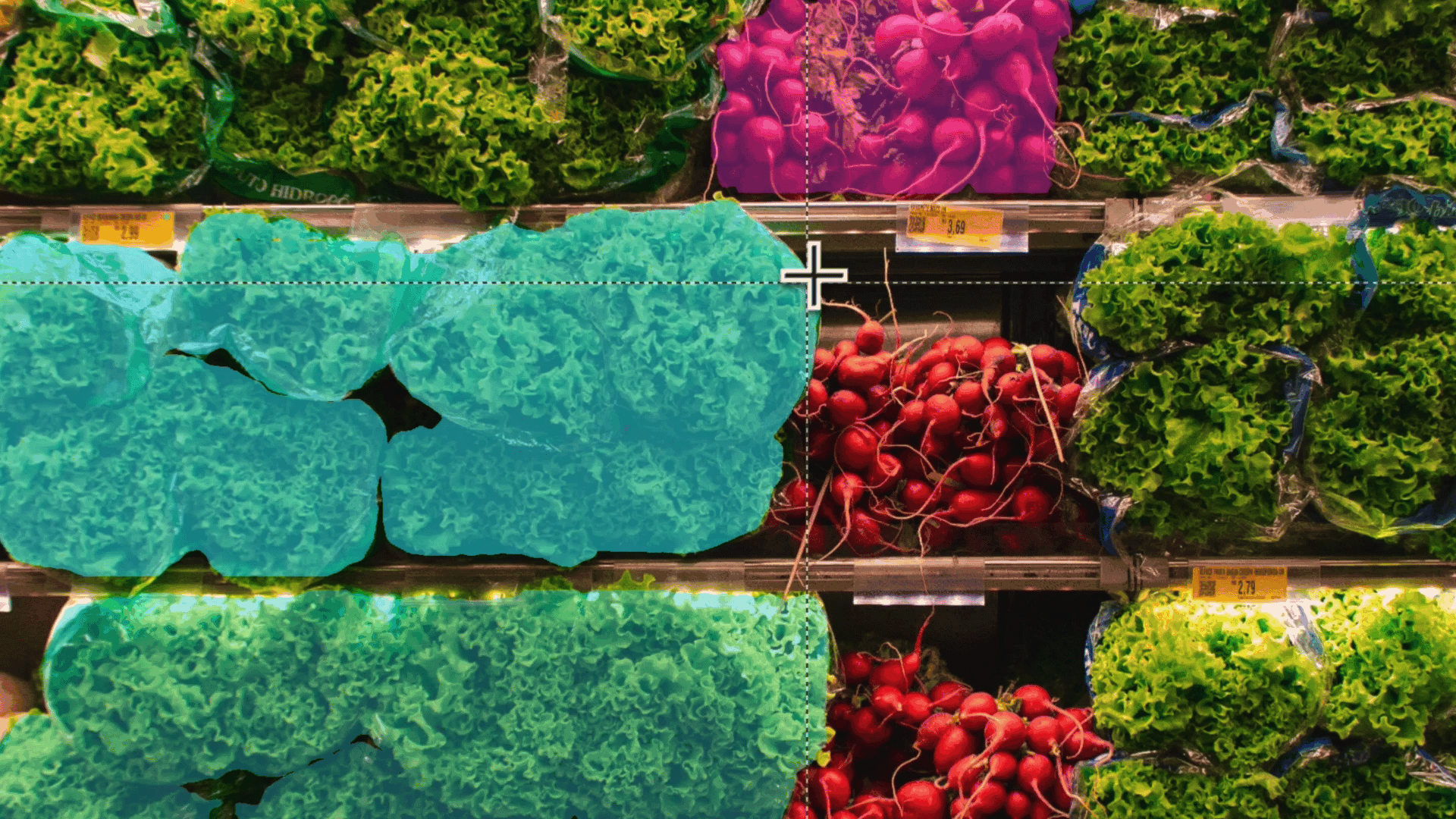
Labelbox is introducing an improved auto-segmentation tool that empowers teams to significantly speed up labeling projects through effortless and intuitive mask drawing editors. As the fastest and most accurate semantic segmentation in the world, the Auto-Segment 2.0 tool will apply the power of Meta AI’s recently released Segment Anything Foundation (SAM) Model in a workflow that helps users rapidly generate high performance training data for their most complex computer vision use cases.
Built for performance and accuracy
Instead of decomposing the mask to vector points, Auto-Segment 2.0 uses a raster-based rendering system that offers unmatched performance at large amount of instances per image. Using a combination of pen and brush tool, users can label detailed nuances and contours for each feature – ultimately yielding higher model performance. Machine learning teams can use Auto-Segment 2.0 to intuitively make automated mask predictions for multiple individual objects in their images across diverse, real-world computer vision use cases, as captured below.
Detecting features from overhead imagery for insurance compliance
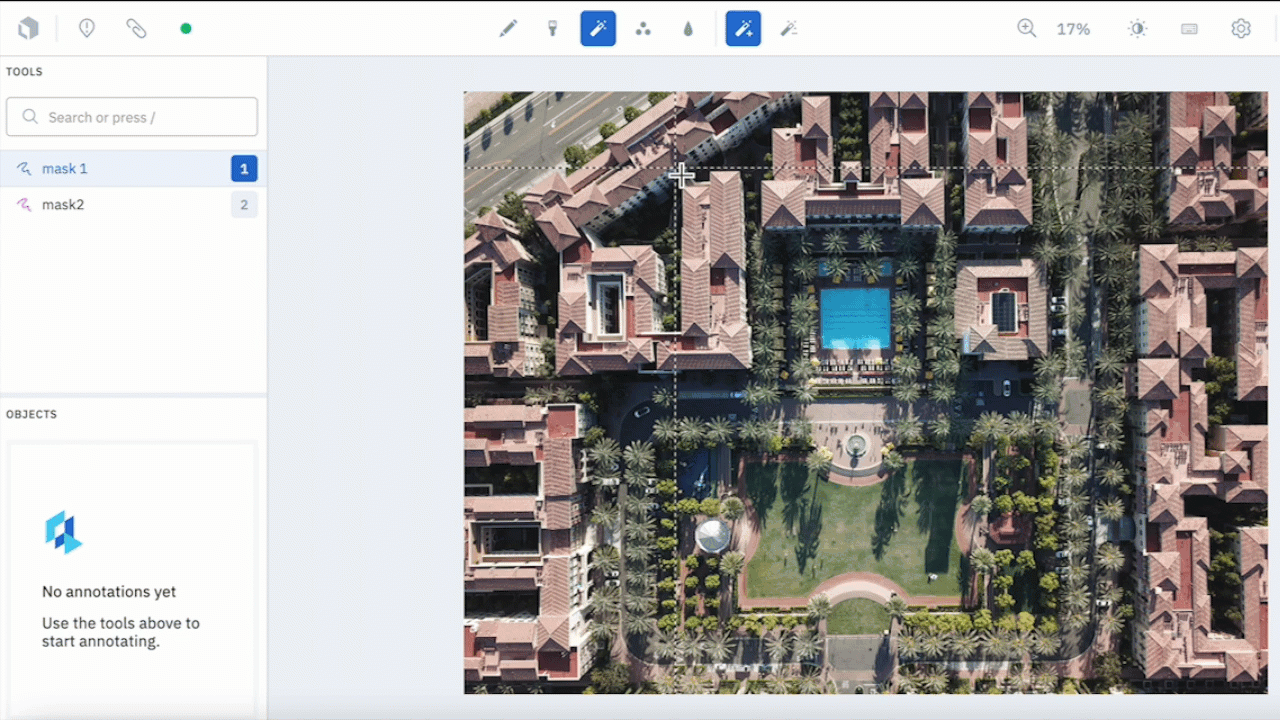
Insurance companies increasingly rely on AI systems for claims automation, underwriting, risk assessment, and more. Computer vision models trained on geospatial data enable insurance companies to assess risks and value for any area or property without human inspection.
Classifying diverse plant species for agriculture
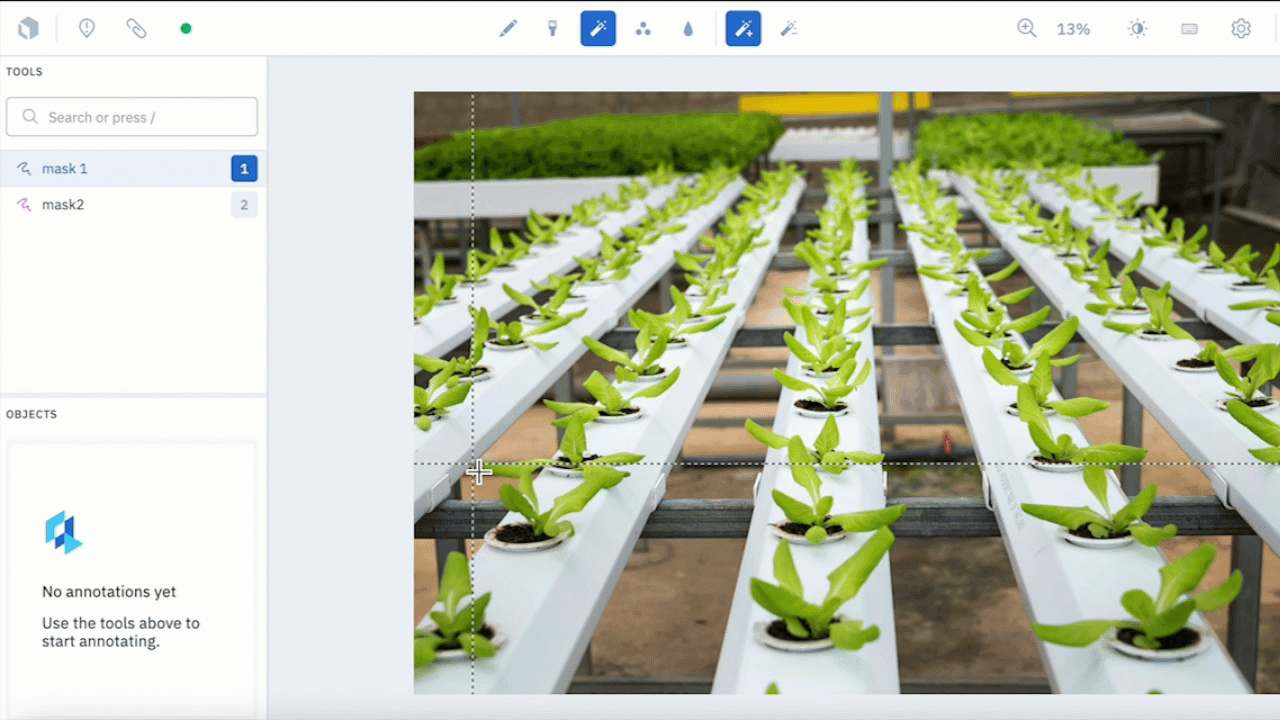
Smart agriculture equipment leverages a suite of computer vision capabilities to detect and classify crops. Applying image segmentation allows those systems to detect detailed patterns and textures on the surface of leaves – playing a crucial role in crop monitoring by preventing the spread of disease to an entire field of crops.
Detecting and diagnosing medical conditions
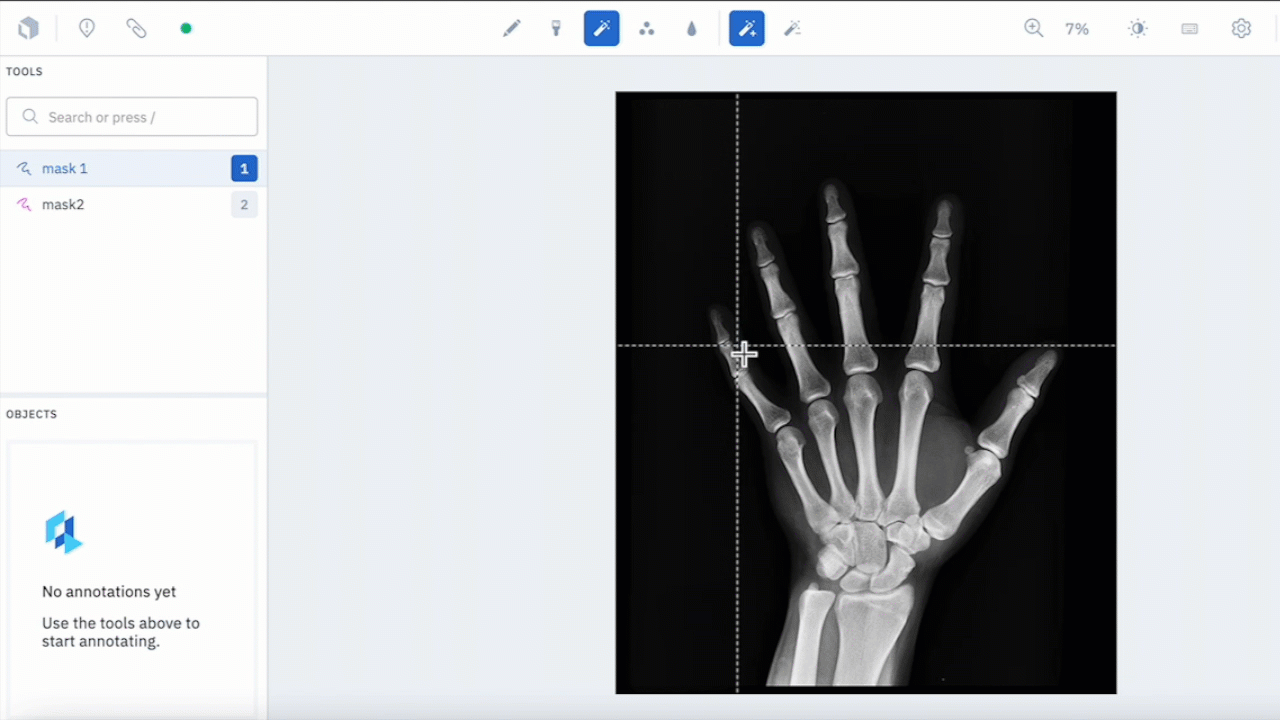
Automatic medical segmentation accelerates rapid and accurate diagnosis and treatment for patients experience symptoms of acute and chronic disease. Image segmentation helps benchmark and classify both healthy and unhealthy regions in an image empowering medical professionals to assess the effectiveness of treatment over time.
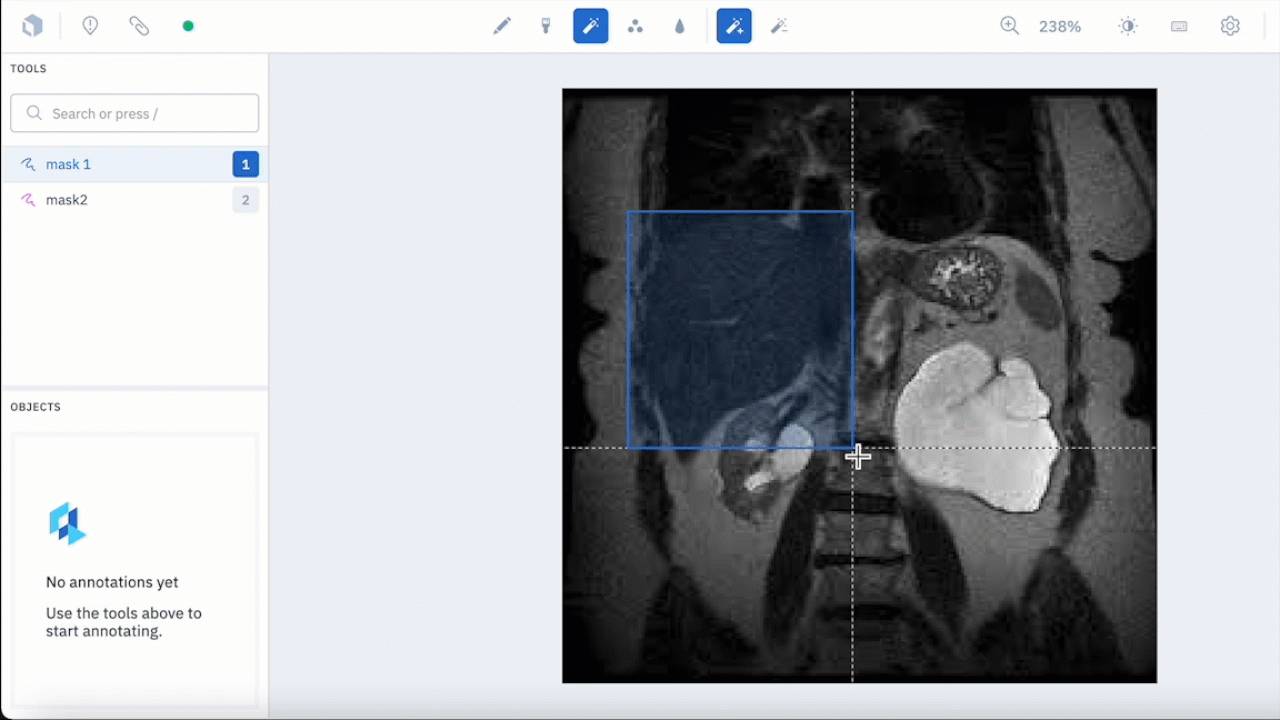
See Auto-Segment 2.0 in action on more examples
Auto-Segment 2.0 performance on use cases: Labeling House Roofs for Inspection; Plants in a Hydroponics Farm; Produce at a Grocery Market; and Bone Scans from a Diagnostic X-ray.
Although image segmentation results vary based on the nuances of each real world application, SAM is already improving the future outlook for machine learning teams leveraging computer vision.
By introducing Auto-Segment 2.0, Labelbox combines innovation with the best zero-shot and transfer learning applications available to solve segmentation challenges, accelerate AI development, and empower users solving the world’s toughest problems.
SAM data engine and model-assisted labeling
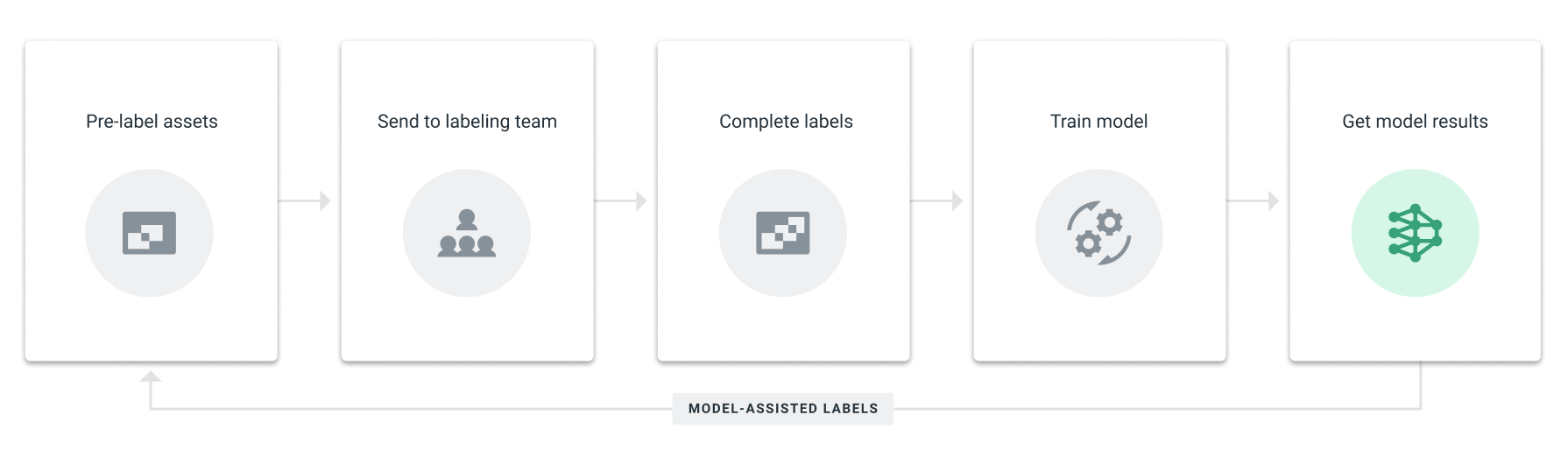
In the original paper, the authors describe their data engine to train SAM model.
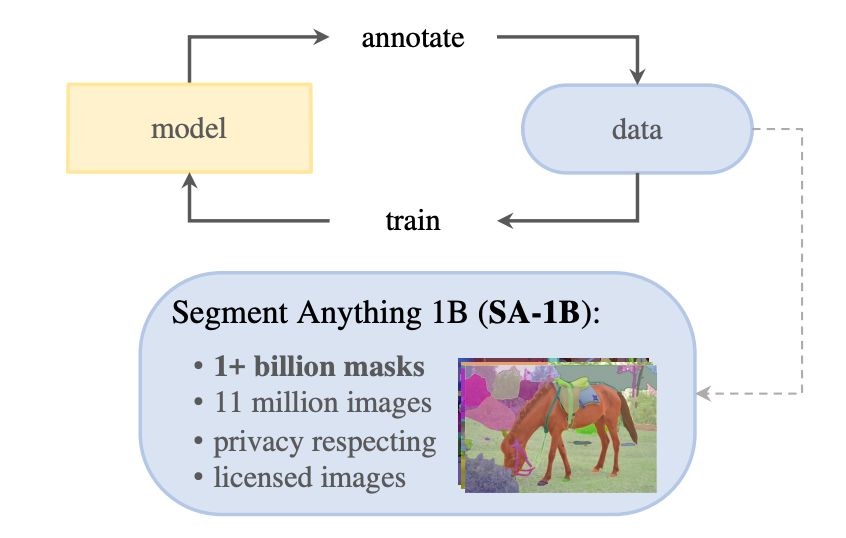
With Labelbox, you can setup this data engine, quickly. You can also use the SAM model to generate pre-labels in bulk and send to humans for corrective / review work such as assigning a correct feature class name. You can even chain multiple models such as detect the object with classname using Yolo V8 and then pass the bounding box and image to SAM model to generate segments. Learn more