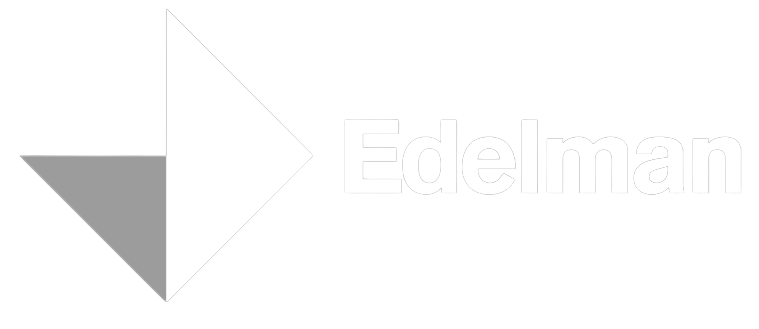
How Edelman harnesses machine learning to better predict consumer trust in brands
Problem
The challenge their ML team wanted to solve was tapping into scalable ways to collect text data from the web and enriching it so that it would increase the chances of their ML models to learn and make accurate predictions on consumer trust in brands.
Solution
The Edelman DxI team adopted the Labelbox platform to annotate their training data. The platform enabled a simple way to select labelers, guide them on what to annotate, as well as assess label quality and labeler performance.
Result
The Edelman team now possesses the infrastructure to continuously collect and iterate on their training data as their projects scale, while having a single labeling solution to serve the data science and machine learning team within Edelman DxI and release projects given tight timelines without delays.
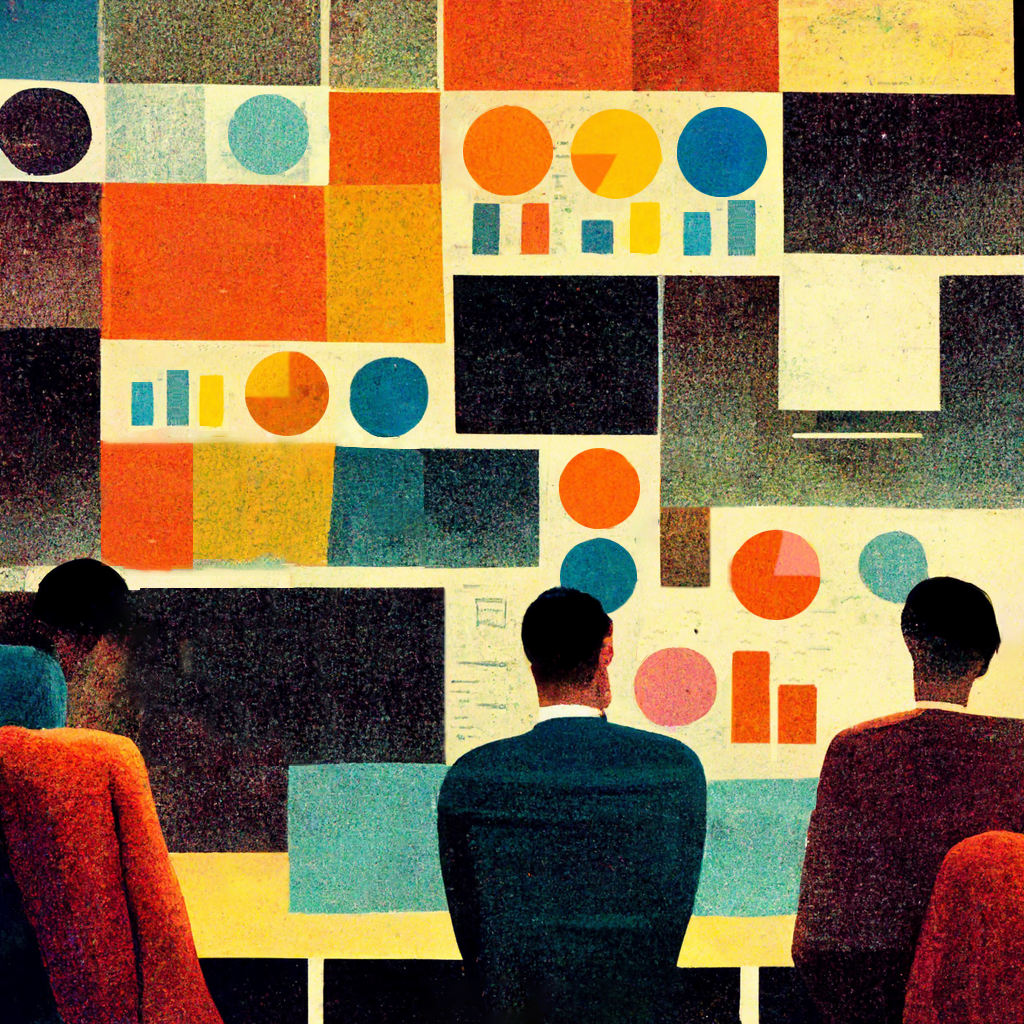
Note: This post is a shortened recap of a virtual talk from David Bartram Shaw (SVP of Global Head of Data Science & Machine Learning at Edelman) during Labelbox Accelerate (Nov 2022).
For more than 20 years, Edelman has been at the forefront of pioneering trust research around the world by turning complex data into real-world insights. These insights have allowed them to identify early signals of changing tides that have led to seismic shifts in culture and society. Edelman Data & Intelligence (also known as DxI) is their analytics and data consultancy which tackles ambitious research projects such as building models to find (1) all the ways that impact how consumers trust brands and (2) in what ways ML models can reasonably detect this from the multitude of unstructured text data available on the web.
As strong models that can score trust currently do not exist, the Edelman DxI is currently working on building proprietary trust algorithms specifically trained to predict consumer trust by examining thousands of data points from earned media, social content, marketing research and numerous other sources to uncover tangible insights and predict results. One of the key challenges, however, is finding scalable ways of collecting this data and enriching it to increase the chances for their ML models to learn and make accurate predictions. In addition, there is a huge volume and variety of datasets available that cover multiple topics & sectors so they want to ensure that they build a representative sample to reduce overfitting and classification bias.
In order to solve this, the Edelman DxI team adopted the Labelbox platform to annotate and create their training data. The platform enabled a simple way to select labelers, guide them on what to annotate, as well as assess label quality and labeler performance. Using the Labelbox Python SDK, the team benefited from a simplified data import and export process that tightly integrated with their AWS data lake. Projects and datasets could now be created programmatically and adding metadata to their assets could be done via an object-oriented way. As a key step in improving their annotation pipeline, the Edelman team wanted to include their internal domain experts who possess institutional knowledge about the specific brand trust domain. These experts would help refine the labeled data by providing their expertise on questions such as “what indicates trust?” and “how do you spot a crisis?”, etc.
In David Bartram Shaw’s words, who currently spearheads the data science and ML initiatives at Edelman DxI, “one of the most important components is around domain expertise. Labeling is expensive. It's less expensive than it used to be thanks to platforms like Labelbox, but it’s still a time intensive process, so we first need to incorporate the domain expertise that we have across our business and our clients and then pull that into ML projects. This enables us to not just rely on labeled data, but we're also relying on our subject matter experts when we incorporate their insights into our ML models.”
Edelman DxI is now able to build a data-centric process - leveraging some of the latest techniques in weak supervision and intelligent sampling - to create high-quality labeled data with minimal effort. In terms of results, their team has been able to train multiple production-grade models to hit their tight timelines. In addition, the Edelman team now possesses the infrastructure to continuously collect and iterate on their training data as their projects scale, while having a single labeling solution to serve the data science and machine learning team within Edelman DxI.