Labelbox•July 14, 2023
Seamlessly integrate Databricks data pipelines with Labelbox
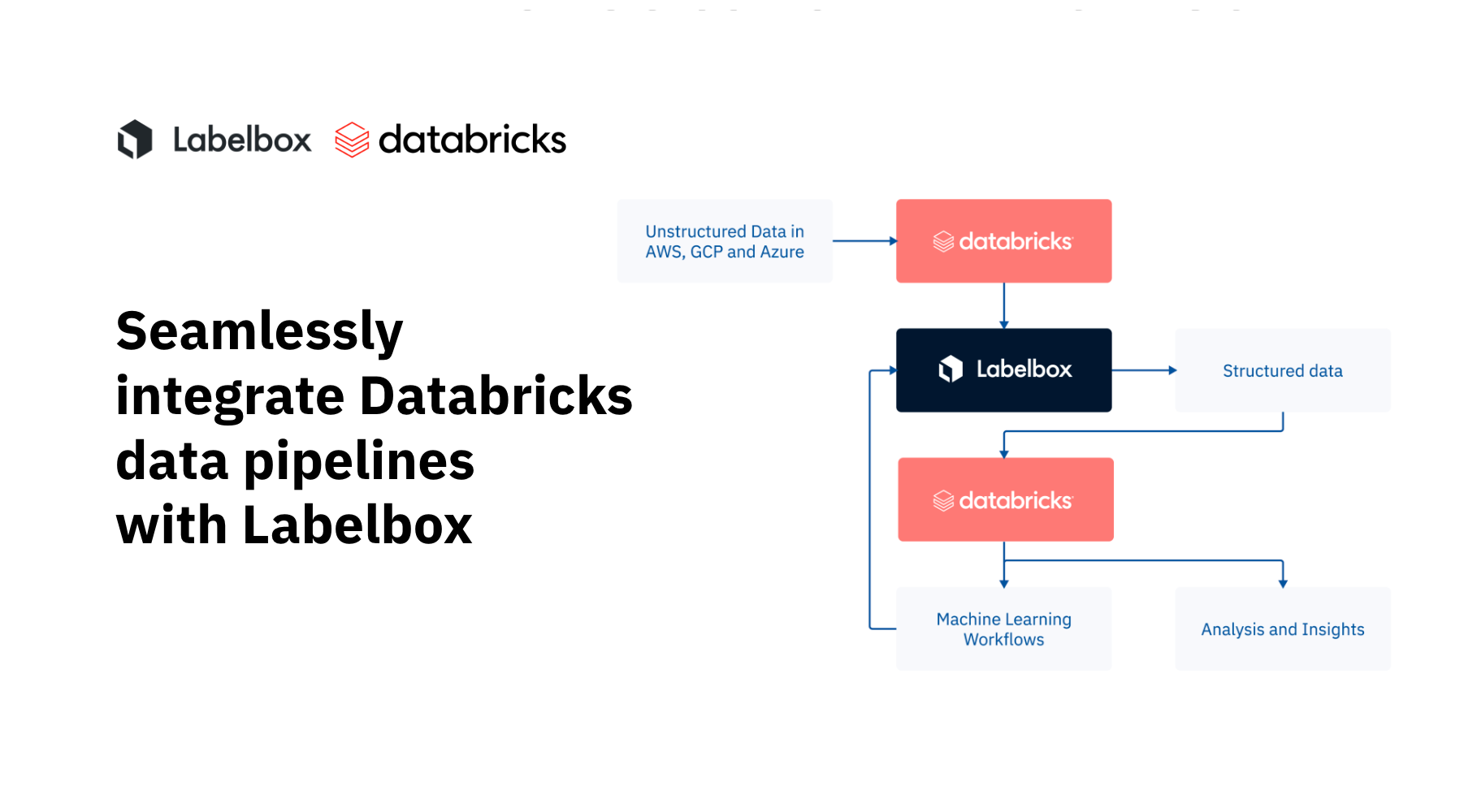
Introduction
In today's data-driven world, organizations across all industries are leveraging the transformative power of AI and machine learning to unlock the value of their unstructured data. These technologies have the potential to revolutionize industries, streamline operations, and drive innovation. With the recent advancements in foundation models, companies are quickly turning to a data-centic approach to building effective AI and ML models.
A data-centric approach offers a multitude of benefits for organizations venturing into the realm of AI and machine learning. By prioritizing data quality, diversity, and accessibility, organizations can unlock the true potential of these technologies and derive meaningful insights. The cornerstones of a data-centric approach are a reliable data pipeline and immense quantities of high quality labeled data.
Databricks, built on Apache Spark, provides a unified analytics and AI platform that empowers organizations to harness the full potential of their data. It offers a collaborative environment where data engineering, data science, and business analytics seamlessly come together.
At the core of Databricks' success lies the Delta Lake, a highly scalable and reliable data lake solution. Delta tables are a critical component of the Delta Lake, they provide data quality, reliability, and reproducibility, enabling organizations to efficiently store, process, and query vast amounts of unstructured data with ease and confidence.
By incorporating Databricks and Delta tables into their AI and machine learning workflows, organizations gain the necessary tools to embrace a data-centric approach fully. They can seamlessly ingest, transform, and analyze diverse and voluminous datasets, ensuring high-quality data inputs for their models. The integration allows organizations to bridge the gap between structured and unstructured data, enabling holistic and comprehensive insights that were previously untapped.
In addition to leveraging the power of Databricks and Delta tables, organizations can further expedite the development of production-ready ML models by leveraging foundation models. Foundation models, such as GPT-4 and Segment Anything Model, provide pre-trained models that serve as a starting point for specific AI applications. By utilizing foundation models, organizations can reduce the time and effort required to develop models from scratch, accelerating the deployment of ML solutions.
Labelbox, an official Databricks Partner, has recently upgraded the existing integration to enable users to quickly and easily connect their Databricks pipeline to upload their unstructured data to Labelbox. Then, users can quickly transform this unstructured data into model-ready training data with the data visualization, enrichment, and curation capabilities offered by Labelbox’s Catalog and Annotate tools. With exciting features such as the new auto-segment tool powered by Meta’s Segment Anything Model and the upcoming release of Model Foundry, generating production-ready training data will be easier than ever.
How it works
The new integration is possible thanks to the Labelbox Connector for Databricks, which provides the following features:
- Upload data and annotations to Labelbox from a Databricks Delta table or Spark DataFrame
- Export Labelbox annotations directly to a Databricks Delta table or Spark DataFrame
With this integration, you can easily automate your data ingestion into Labelbox through a Databricks workflow job. These jobs can be scheduled to run periodically, saving file links from your cloud storage bucket to a Delta table and then automatically triggering an upload job with Labelspark. With automated data ingestion, you can spend more time curating high quality training data in Labelbox.
Databricks integration demo
Check out the video below for an overview of how to upload data to Labelbox directly from your Databricks Delta table. To get started, feel free to follow along in the notebook here and we'd love to hear any feedback or how we can help you tailor this to your upcoming use cases.